MDCC-Net: Multiscale double-channel convolution U-Net framework for colorectal tumor segmentation.
Computers in biology and medicine(2020)
摘要
PURPOSE:Multiscale feature fusion is a feasible method to improve tumor segmentation accuracy. However, current multiscale networks have two common problems: 1. Some networks only allow feature fusion between encoders and decoders of the same scale. It is obvious that such feature fusion is not sufficient. 2. Some networks have too many dense skip connections and too much nesting between the coding layer and the decoding layer, which causes some features to be lost and means that not enough information will be learned from multiple scales. To overcome these two problems, we propose a multiscale double-channel convolution U-Net (MDCC-Net) framework for colorectal tumor segmentation.
METHODS:In the coding layer, we designed a dual-channel separation and convolution module and then added residual connections to perform multiscale feature fusion on the input image and the feature map after dual-channel separation and convolution. By fusing features at different scales in the same coding layer, the network can fully extract the detailed information of the original image and learn more tumor boundary information.
RESULTS:The segmentation results show that our proposed method has a high accuracy, with a Dice similarity coefficient (DSC) of 83.57%, which is an improvement of 9.59%, 6.42%, and 1.57% compared with nnU-Net, U-Net, and U-Net++, respectively.
CONCLUSION:The experimental results show that our proposed method has good performance in the segmentation of colorectal tumors and is close to the expert level. The proposed method has potential clinical applicability.
更多查看译文
AI 理解论文
溯源树
样例
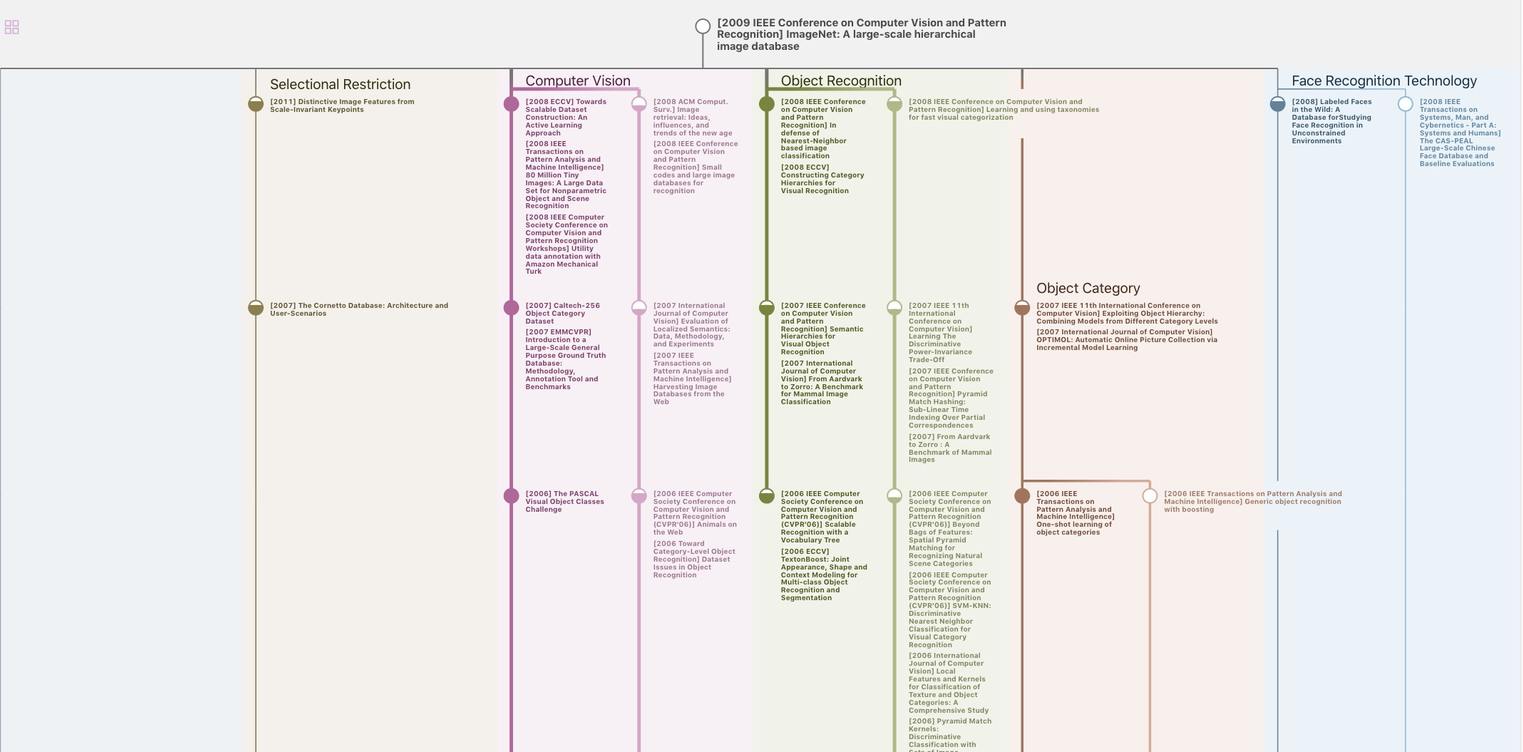
生成溯源树,研究论文发展脉络
Chat Paper
正在生成论文摘要