Smoothed Gaussian Mixture Models for Video Classification and Recommendation
arxiv(2020)
摘要
Cluster-and-aggregate techniques such as Vector of Locally Aggregated Descriptors (VLAD), and their end-to-end discriminatively trained equivalents like NetVLAD have recently been popular for video classification and action recognition tasks. These techniques operate by assigning video frames to clusters and then representing the video by aggregating residuals of frames with respect to the mean of each cluster. Since some clusters may see very little video-specific data, these features can be noisy. In this paper, we propose a new cluster-and-aggregate method which we call smoothed Gaussian mixture model (SGMM), and its end-to-end discriminatively trained equivalent, which we call deep smoothed Gaussian mixture model (DSGMM). SGMM represents each video by the parameters of a Gaussian mixture model (GMM) trained for that video. Low-count clusters are addressed by smoothing the video-specific estimates with a universal background model (UBM) trained on a large number of videos. The primary benefit of SGMM over VLAD is smoothing which makes it less sensitive to small number of training samples. We show, through extensive experiments on the YouTube-8M classification task, that SGMM/DSGMM is consistently better than VLAD/NetVLAD by a small but statistically significant margin. We also show results using a dataset created at LinkedIn to predict if a member will watch an uploaded video.
更多查看译文
关键词
video classification,recommendation,mixture,models
AI 理解论文
溯源树
样例
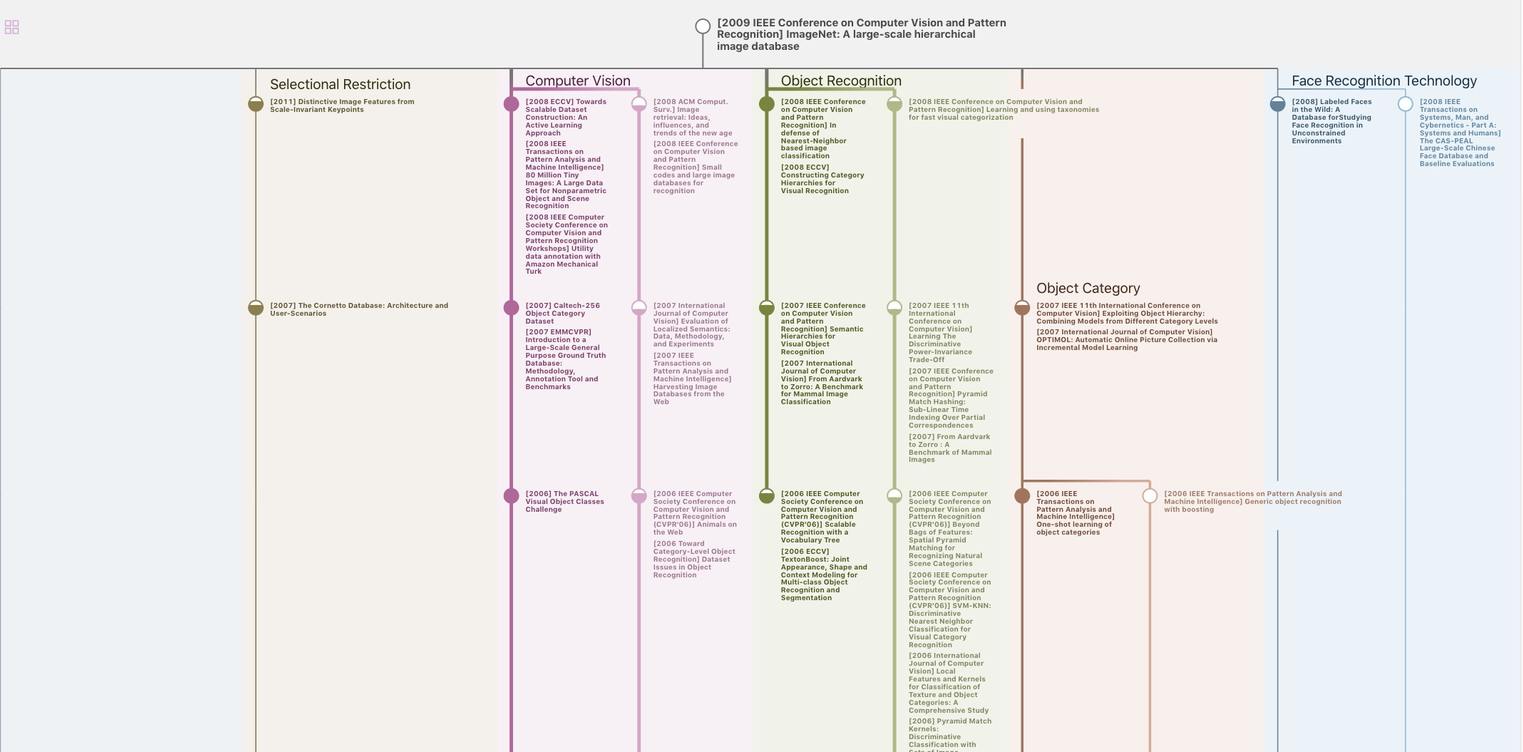
生成溯源树,研究论文发展脉络
Chat Paper
正在生成论文摘要