Data stream event prediction based on timing knowledge and state transitions
Hosted Content(2020)
摘要
AbstractWe study a practical problem of predicting the upcoming events in data streams using a novel approach. Treating event time orders as relationship types between event entities, we build a dynamic knowledge graph and use it to predict future event timing. A unique aspect of this knowledge graph embedding approach for prediction is that we enhance conventional knowledge graphs with the notion of "states"---in what we call the ephemeral state nodes---to characterize the state of a data stream over time. We devise a complete set of methods for learning relevant events, for building the event-order graph stream from the original data stream, for embedding and prediction, and for theoretically bounding the complexity. We evaluate our approach with four real world stream datasets and find that our method results in high precision and recall values for event timing prediction, ranging between 0.7 and nearly 1, significantly outperforming baseline approaches. Moreover, due to our choice of efficient translation-based embedding, the overall throughput that the stream system can handle, including continuous graph building, training, and event predictions, is over one thousand to sixty thousand tuples per second even on a personal computer---which is especially important in resource constrained environments, including edge computing.
更多查看译文
AI 理解论文
溯源树
样例
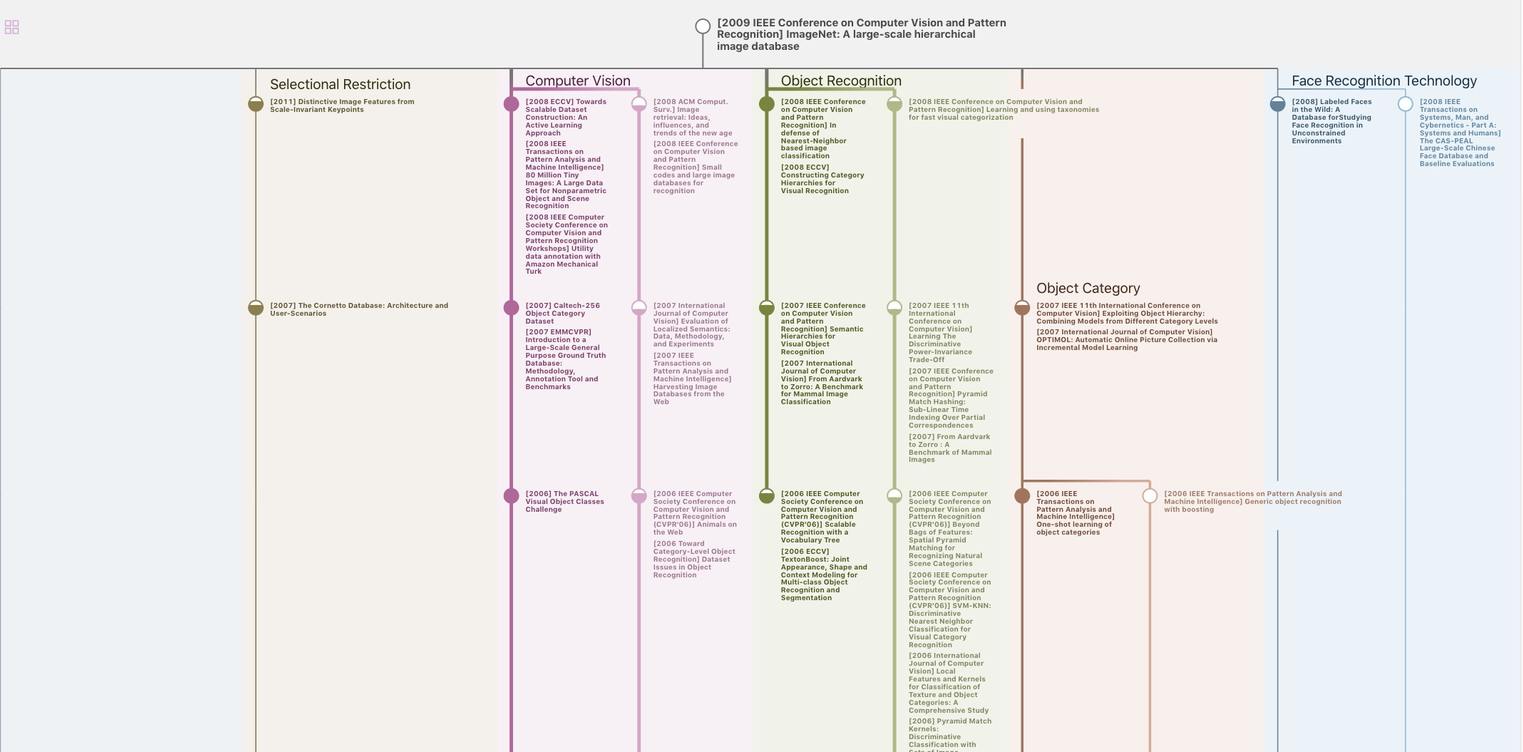
生成溯源树,研究论文发展脉络
Chat Paper
正在生成论文摘要