Active learning with deep Bayesian neural network for laser control
Proceedings of SPIE(2018)
摘要
New control techniques are required to utilize the full potential of next generation high-energy high-repetition-rate pulses lasers while ensuring their safe operation. During automated optimization of an experiment, the control system is required to identify and reject unsafe laser configurations proposed by the optimizer. Using conventional physics codes render impossible when applied to a high energy laser system with 1ms or less time between shots, and also including laser fluctuations and drift. To mitigate this, we are using a deep Bayesian neural network to map the laser's input power spectrum to its output power spectrum and demonstrate the speed of this approach. The Bayesian neural network can provide an estimate of its own uncertainty as a function of wavelength. A recently developed algorithm enables the uncertainty to be calculated inexpensively using multiple dropout layers inserted into the model. The uncertainty estimates are used by an active learning algorithm to improve the accuracy of the model and intelligently explore the input domain.
更多查看译文
关键词
machine learning,optical damage,control
AI 理解论文
溯源树
样例
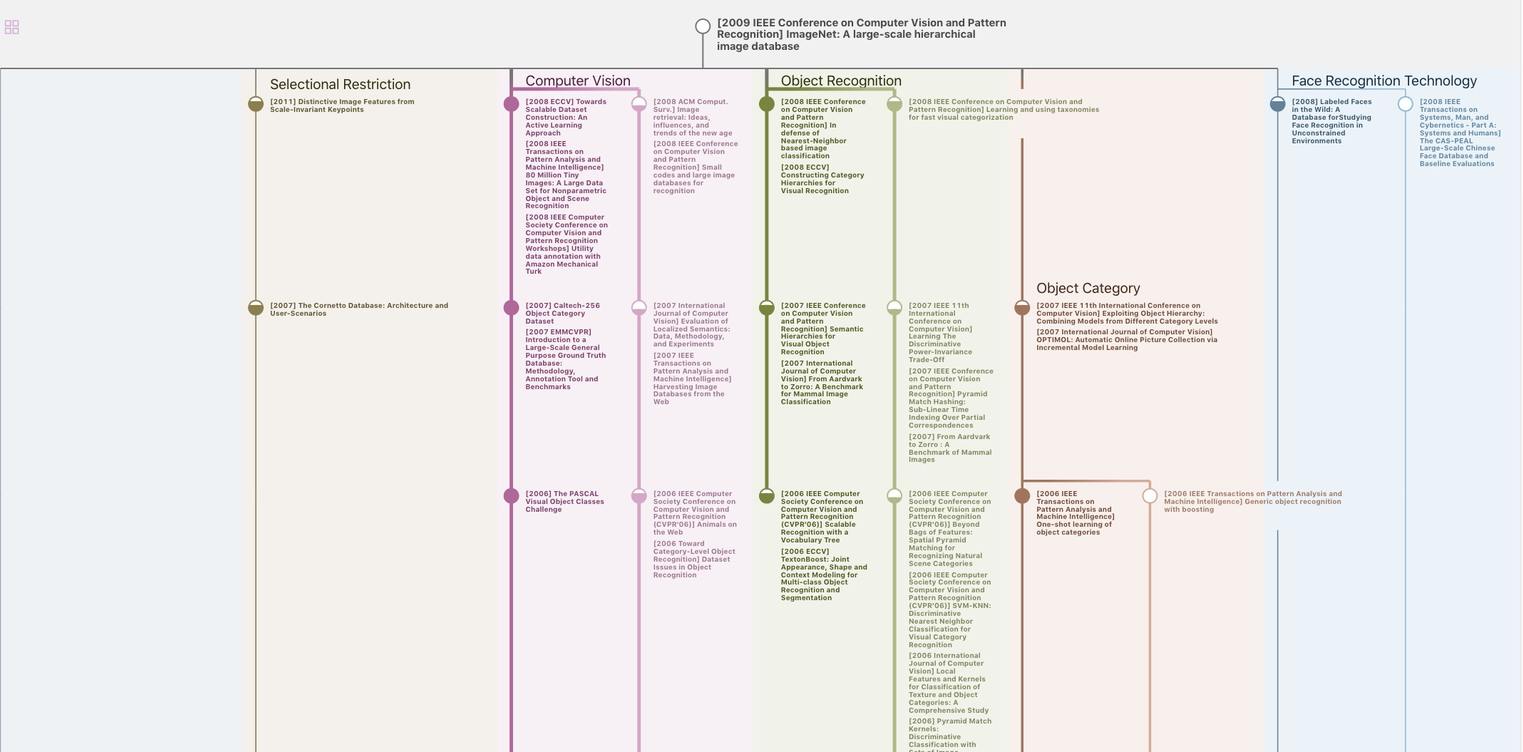
生成溯源树,研究论文发展脉络
Chat Paper
正在生成论文摘要