Quality assurance of streaming oceanographic data sets: using the data stream as a metric of quality
OCEANS-IEEE(2017)
摘要
The QARTOD methodology of a standard sequential string of tests on individual measurements has high applicability to constrained data systems. The QARTOD methodology has the benefit of a coherent and replicable test structure that enables relatively easy expansion into new measurements. However, as data streams become more complex the series of tests described becomes increasingly difficult to analyse as the number of potential flags increases. When data products that are derived from multiple data streams are included in real time accessible outputs, e.g. temperature or pressure corrected values, the flagging structure will become larger than the original data sets. A real time quality assurance structure will ultimately fail if the analytical methods generate more data than the original data stream. Effective data analysis of a constant input data stream is dependent on timely analysis, as a near constant stream of new data will overwhelm even the most dedicated team. Therefore, an effective quality assurance mechanism must become part of the data delivery system else there will always be a growing data overhang. While the promise of QARTOD is real time quality assurance, an ever increasing flag structure will quickly undermine the potential utility, and is already hampering implementation for the glider fleet (John Kerfoot, personal communication). We present a potential method to escape this fate. We consider that a timely assessment of data quality is dependent on an understanding of the nature of the sensors themselves. This includes quantification of the change in the sensor response and signal over the expected use envelope (e.g. temperature, pressure) and life of the instrument. With that described, the sensor response can be quantified with respect to the intrinsic scale of the measurement and the target. The QARTOD method currently encompasses this as a range test. We prefer to consider this as two separate stages: the range of variance expected from the sensor itself and the variance above that level that encompasses the expected range of the signal from the target. This does not remove the need for a target range envelope, but we can then consider where to derive the range from. Within the context of longer time series we recommend substituting an expected range for a range derived from the data stream itself. This makes the initialization of new data streams somewhat unstable, but avoids the need to assume a range of values from an unknown environment or an area where the application of previous data to current methods may involve step changes that inappropriately flag data. We can then treat each data stream separately with internally derived comparisons to previous data: Minimum Value (t0 to t n-1) < Value (t = n) < Maximum Value (t0 to t n-1) Since we are presumably collecting multiple measurands, and within the ocean there are expected relationships across measureands, i.e. temperature and salinity relationships between water masses, we can analyse each measurand as its own variance structure with respect to time and space and expect that we will have coherence in the variance if not the absolute values of the measurands. Presenting the data streams as variance structures allows for methodological comparisons that do not need defined boundaries from prior data sets. We use a public moored data set as our test data for this analysis to describe the applicability of the method to data streams with variances that are driven primarily by time. The applicability of this method to mobile resources (floats, gliders, underway systems) is left to the future.
更多查看译文
关键词
QARTOD,quality assurance,real-time data
AI 理解论文
溯源树
样例
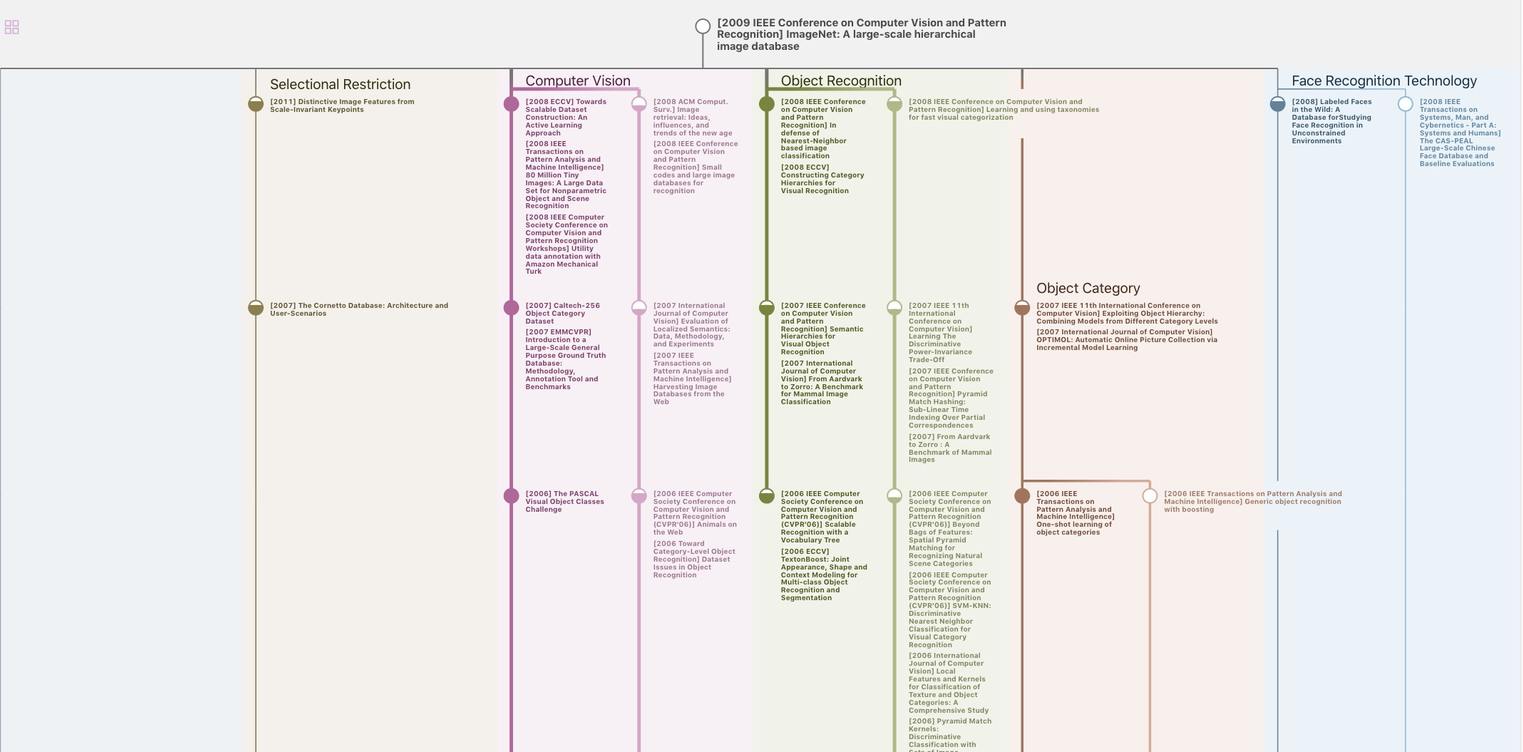
生成溯源树,研究论文发展脉络
Chat Paper
正在生成论文摘要