Planning from Pixels using Inverse Dynamics Models
ICLR(2021)
摘要
Learning task-agnostic dynamics models in high-dimensional observation spaces can be challenging for model-based RL agents. We propose a novel way to learn latent world models by learning to predict sequences of future actions conditioned on task completion. These task-conditioned models adaptively focus modeling capacity on task-relevant dynamics, while simultaneously serving as an effective heuristic for planning with sparse rewards. We evaluate our method on challenging visual goal completion tasks and show a substantial increase in performance compared to prior model-free approaches.
更多查看译文
关键词
planning,dynamics,pixels,models
AI 理解论文
溯源树
样例
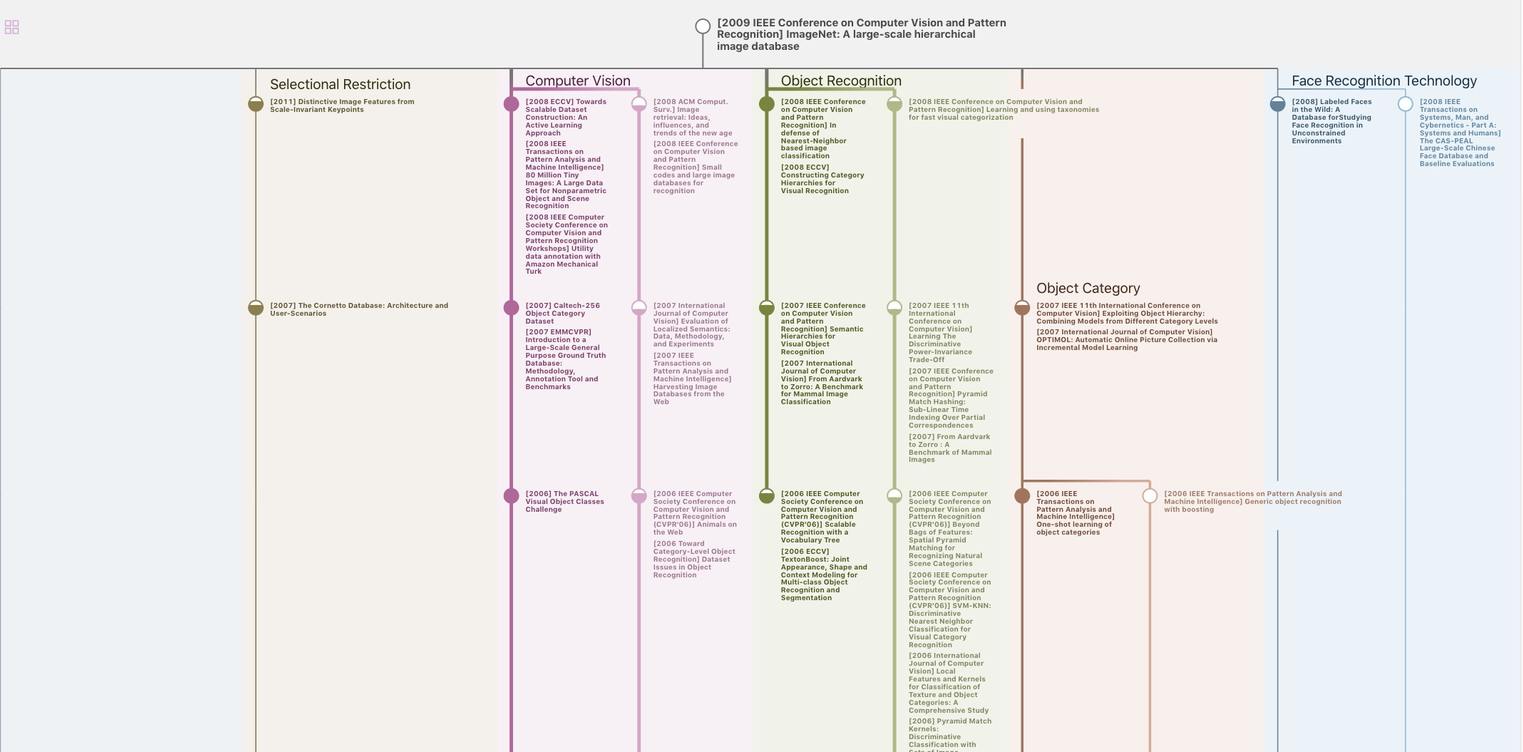
生成溯源树,研究论文发展脉络
Chat Paper
正在生成论文摘要