MYSTIKO: Cloud-Mediated, Private, Federated Gradient Descent
2020 IEEE 13th International Conference on Cloud Computing (CLOUD)(2020)
摘要
Federated learning enables multiple, distributed participants (potentially on different clouds) to collaborate and train machine/deep learning models by sharing parameters/gradients. However, sharing gradients, instead of centralizing data, may not be as private as one would expect. Reverse engineering attacks [1], [2] on plaintext gradients have been demonstrated to be practically feasible. Existing solutions for differentially private federated learning, while promising, lead to less accurate models and require nontrivial hyperparameter tuning. In this paper, we examine the use of additive homomorphic encryption (specifically the Paillier cipher) to design secure federated gradient descent techniques that (i) do not require addition of statistical noise or hyperparameter tuning, (ii) does not alter the accuracy or utility of the final model, (iii) ensure that the plaintext model parameters/gradients of a participant are never revealed to any other participant or third party coordinator involved in the federated learning job, (iv) minimize the trust placed in any third party coordinator and (v) are efficient, with minimal overhead, and cost effective.
更多查看译文
关键词
federated learning,gradient descent,aggregator,additive homomorphic encryption,all-reduce
AI 理解论文
溯源树
样例
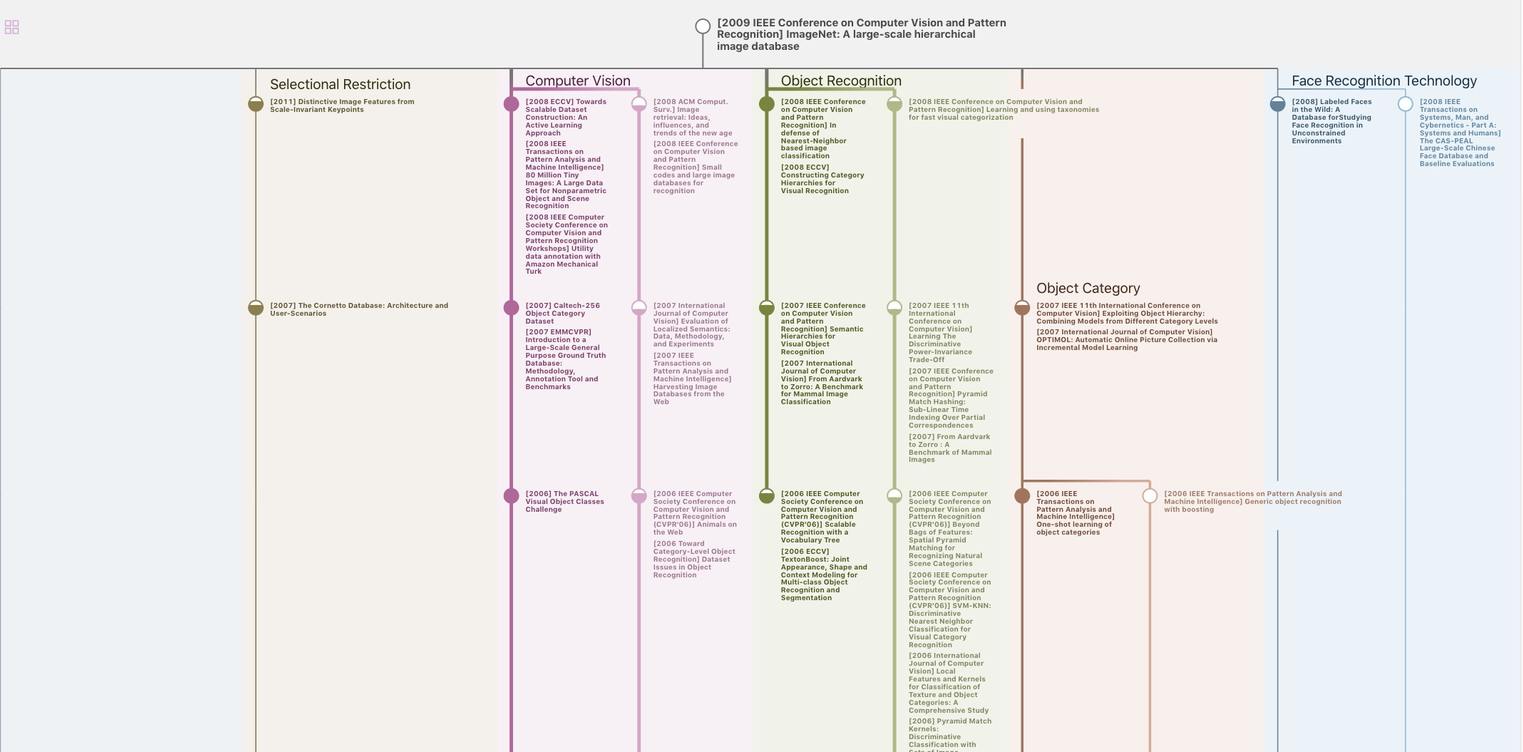
生成溯源树,研究论文发展脉络
Chat Paper
正在生成论文摘要