Scaled First-Order Methods for a Class of Large-Scale Constrained Least Square Problems
AIP Conference Proceedings(2016)
摘要
Typical applications in signal and image processing often require the numerical solution of large scale linear least squares problems with simple constraints, related to an m x n nonnegative matrix A, m << n. When the size of A is such that the matrix is not available in memory and only the operators of the matrix-vector products involving A and A(T) can be computed, forward backward methods combined with suitable accelerating techniques are very effective; in particular, the gradient projection methods can be improved by suitable step length rules or by an extrapolation/inertial step. In this work, we propose a further acceleration technique for both schemes, based on the use of variable metrics tailored for the considered problems. The numerical effectiveness of the proposed approach is evaluated on randomly generated test problems and real data arising from a problem of fibre orientation estimation in diffusion MRI.
更多查看译文
AI 理解论文
溯源树
样例
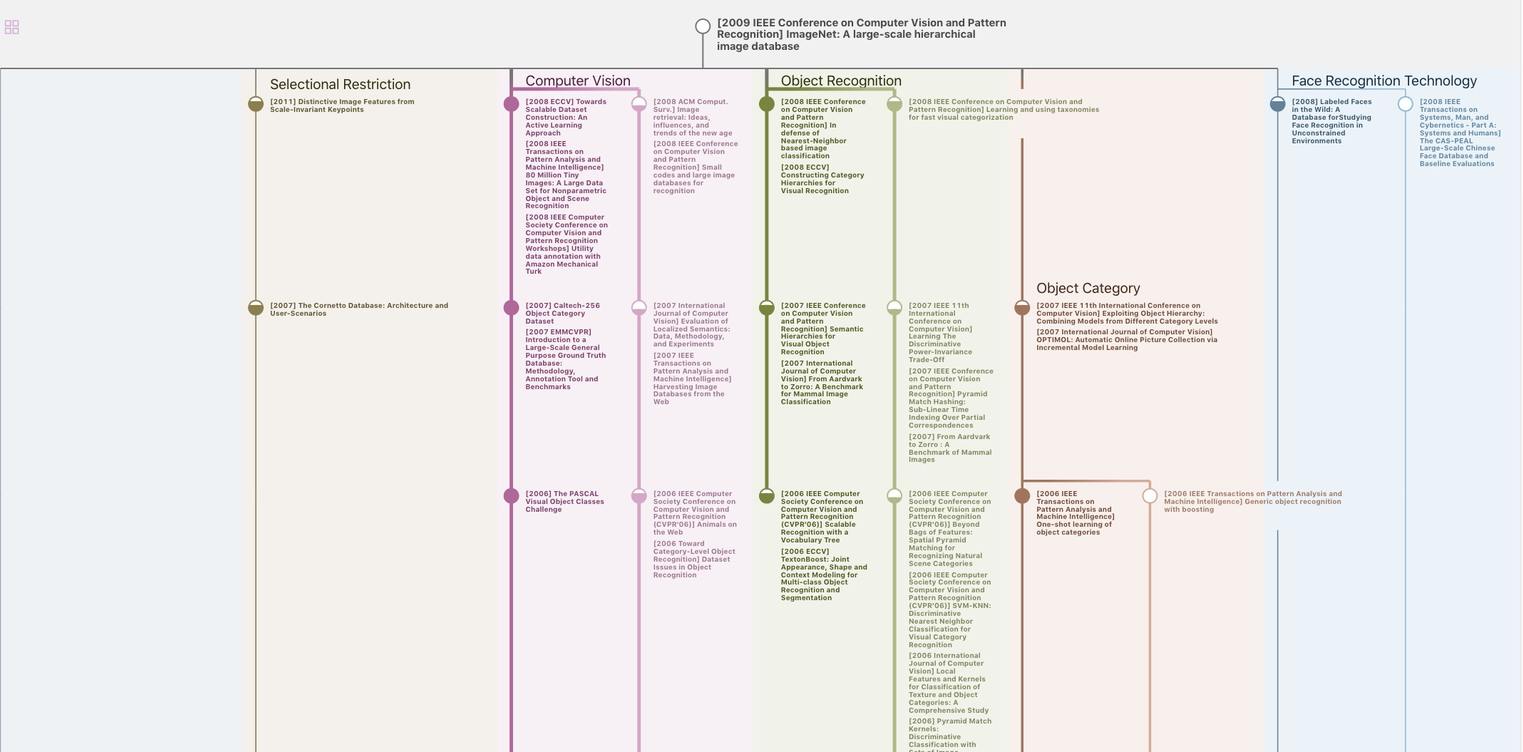
生成溯源树,研究论文发展脉络
Chat Paper
正在生成论文摘要