Using Class Based Document Frequency to Select Features in Text Classification
Communications in Computer and Information Science(2016)
摘要
Document Frequency (DF) is reported to be a simple yet quite effective measure for feature selection in text classification, which is a key step in processing big textual data collections. The calculation is based on how many documents in a collection contain a feature, which can be a word, a phrase, a n-gram, or a specially derived attribute. It is an unsupervised and class independent metric. Features of the same DF value may have quite different distribution over different categories, and thus have different discriminative power over categories. For example, in a binary classification problem, if feature A only appears in one category, but feature B, which has the same DF value as feature A, is evenly distributed in both categories. Then, feature A is obviously more effective than feature B for classification. To overcome this weakness of the original document frequency feature selection metric, we, therefore, propose a class based document frequency strategy to further refine the original DF to some extent. Extensive experiments on three text classification datasets demonstrate the effectiveness of the proposed measures.
更多查看译文
关键词
Document Frequency (DF), Text Categorization, Feature Selection Metrics, Independent Metrics, Text Classification Problem
AI 理解论文
溯源树
样例
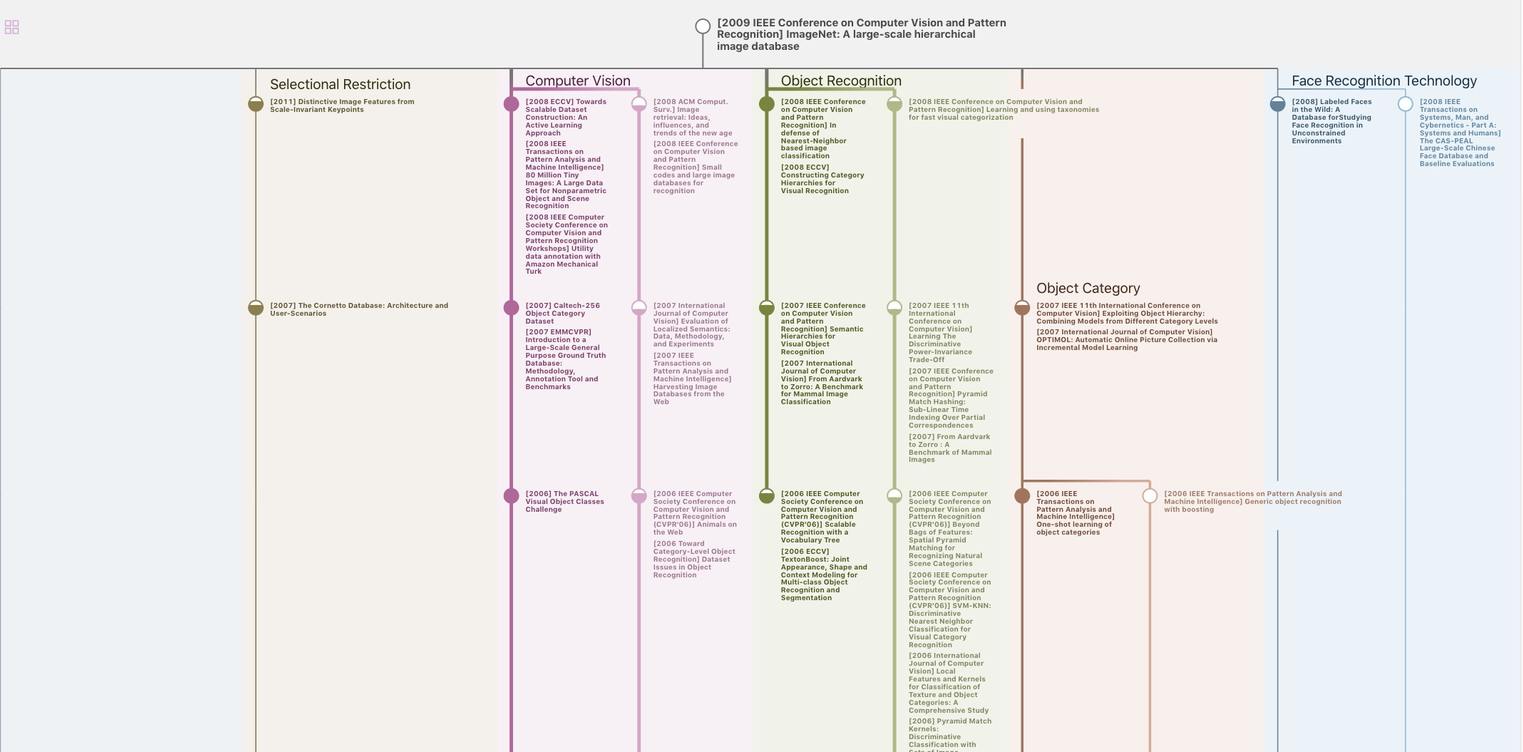
生成溯源树,研究论文发展脉络
Chat Paper
正在生成论文摘要