Two-Way Neural Machine Translation: A Proof Of Concept For Bidirectional Translation Modeling Using A Two-Dimensional Grid
2021 IEEE SPOKEN LANGUAGE TECHNOLOGY WORKSHOP (SLT)(2021)
Abstract
Neural translation models have proven to be effective in capturing sufficient information from a source sentence and generating a high-quality target sentence. However, it is not easy to get the best effect for bidirectional translation, i.e., both source-to-target and target-to-source translation using a single model. If we exclude some pioneering attempts, such as multilingual systems, all other bidirectional translation approaches are required to train two individual models. This paper proposes to build a single end-to-end bidirectional translation model using a two-dimensional grid, where the left-to-right decoding generates source-to-target, and the bottom-to-up decoding creates target-to-source output. Instead of training two models independently, our approach encourages a single network to jointly learn to translate in both directions. Experiments on theWMT2018 German <-> English and Turkish <-> English translation tasks show that the proposed model is capable of generating a good translation quality and has sufficient potential to direct the research.
MoreTranslated text
Key words
Bidirectional translation, Two-way translation, 2D sequence-to-sequence modeling
AI Read Science
Must-Reading Tree
Example
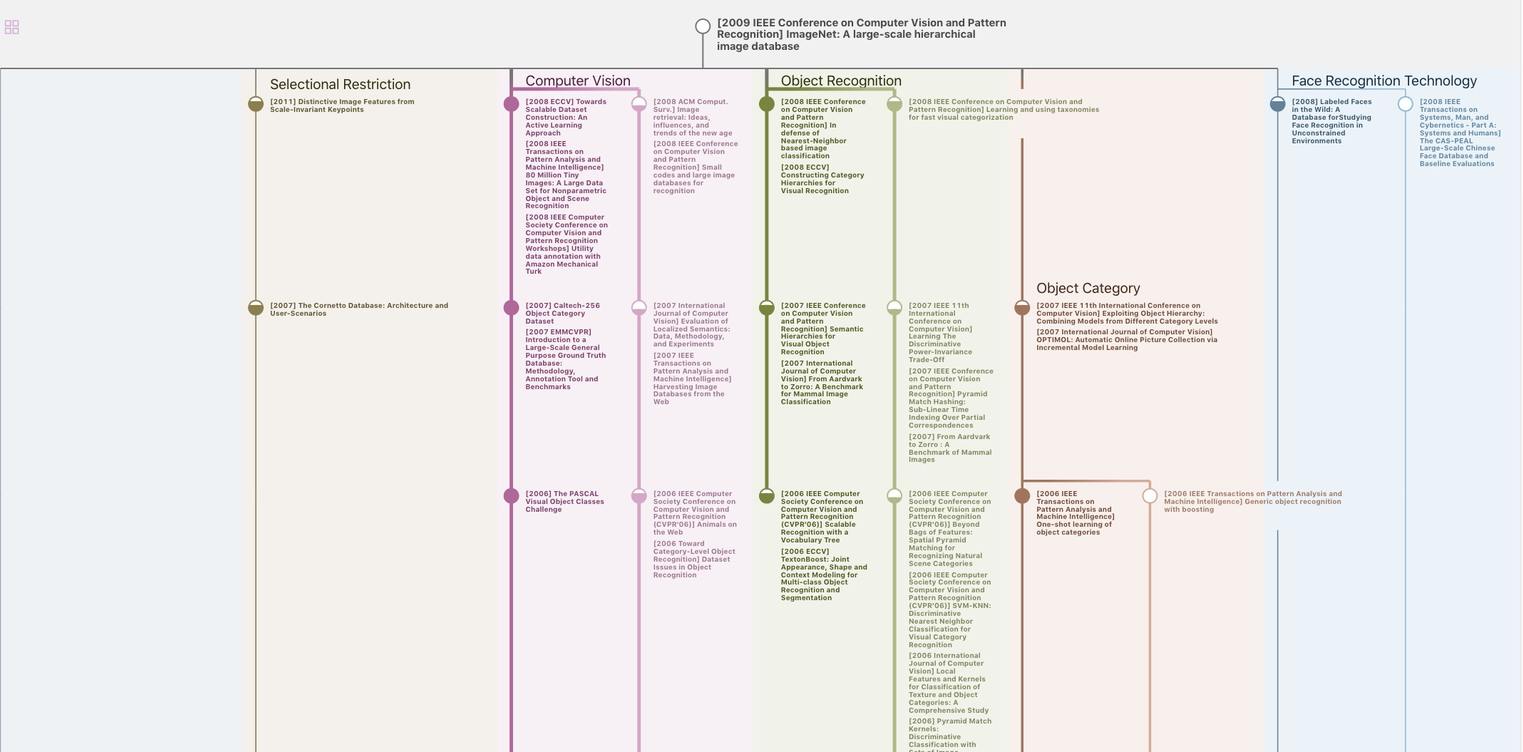
Generate MRT to find the research sequence of this paper
Chat Paper
Summary is being generated by the instructions you defined