Knowledge Discovery from Temporal Social Networks
Intelligent Informatics(2018)
摘要
Extracting knowledge from network data is a complex task. It requires the use of appropriate tools and techniques, especially in scenarios that take into account the volume and evolving aspects of the network. There is a vast literature on how to collect, process, and model social media data in the form of networks, as well as key metrics of centrality. However, there is still much to be discussed in relation to the analysis of the underlying huge networks. In this article the goal is to discuss briefly different techniques in the process of gaining knowledge from networked data, especially considering time perspective. Firstly, we presented some techniques for online sampling of temporally ordered massive networked data which can be efficiently plugged in for a further network mining task. Next are discussed approximate mechanisms for high speed network change detection using centrality measures. Hand in hand we also presented, the ways of processing temporal network streams, applications with real data and illustrations using network visualizations. In the end are discussed concepts related to temporal ordering of links and paths in temporal networks.
更多查看译文
AI 理解论文
溯源树
样例
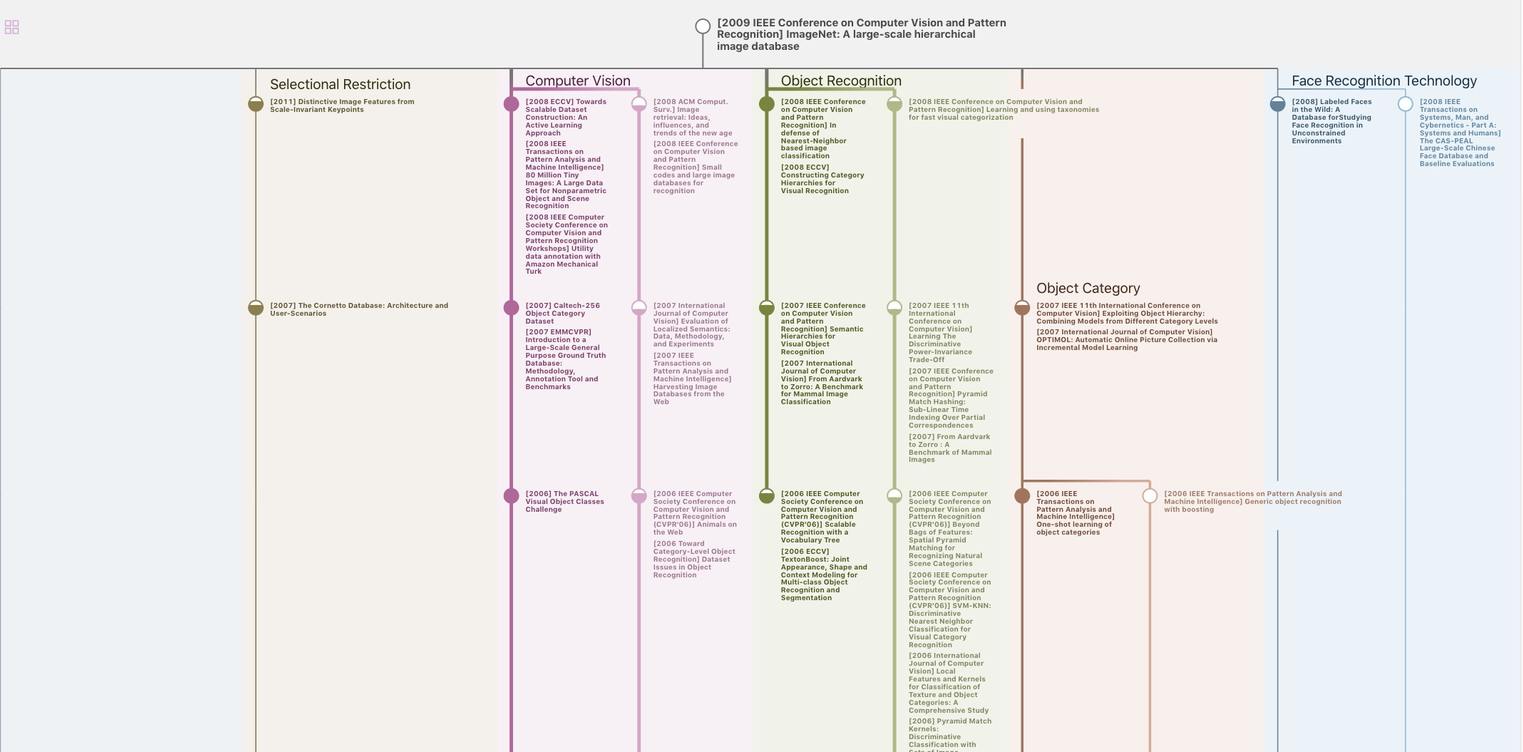
生成溯源树,研究论文发展脉络
Chat Paper
正在生成论文摘要