Speculative Text Mining for Document-level Sentiment Classification
Neurocomputing(2020)
摘要
Many existing solutions perform document-level sentiment classification based on local document only, ignoring other texts that might contribute to better classification accuracy. In this paper, we propose a novel speculative sentiment classification model named SSC. In SSC, we speculate that users with similar rating behaviours are more likely to write documents of similar sentiments toward a product. The motivation of SSC, therefore, is to exploit those speculative similar documents for improving classification accuracy. The proposed SSC model consists of three main components, namely, user-product interaction (UPI) component, document encoding (DE) component, and speculative similar document (SSD) component. The UPI component models user-product interactions, and encodes user/product ratings behaviours into user/product embeddings. The DE component utilizes learned user/product embeddings to capture the informative word vectors for comprising more accurate document representations. The SSD component aggregates documents written by similar users toward the same product for speculative sentiment classification. Because the user similarities are calculated based on user embeddings that encode user rating behaviours, the aggregated documents are more likely to have similar sentiments. The three components are seamlessly integrated into a unified model. In the unified manner, these three components are jointly optimized, and they mutually complement each other to enhance sentiment classification. We conduct extensive experiments on three public datasets, and demonstrate the advantage of the proposed SSC model over state-of-the-art baselines.
更多查看译文
关键词
Speculative sentiment classification,Speculative similar document
AI 理解论文
溯源树
样例
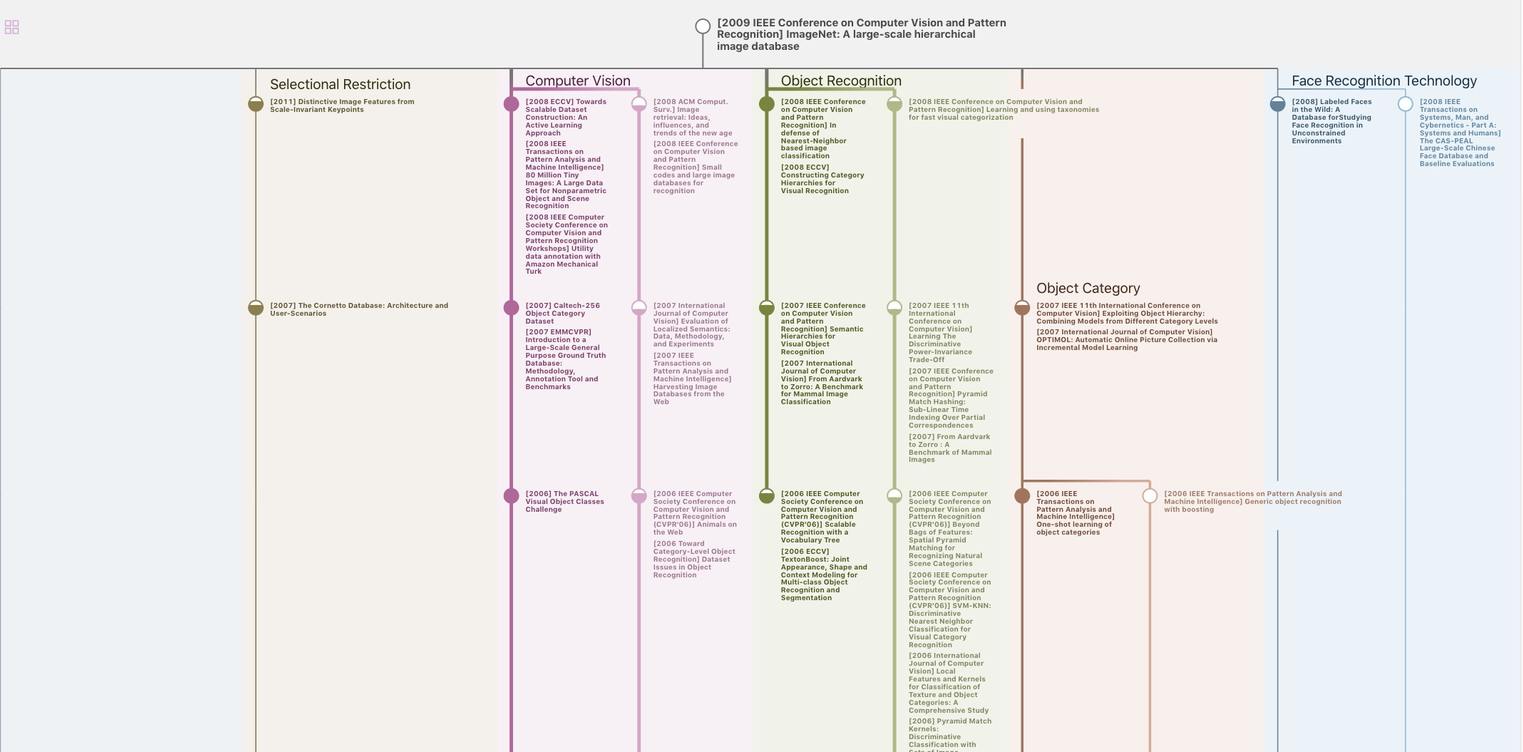
生成溯源树,研究论文发展脉络
Chat Paper
正在生成论文摘要