Innovative Deep Neural Network Modeling for Fine-Grained Chinese Entity Recognition
ELECTRONICS(2020)
摘要
Named entity recognition (NER) is a basic but crucial task in the field of natural language processing (NLP) and big data analysis. The recognition of named entities based on Chinese is more complicated and difficult than English, which makes the task of NER in Chinese more challenging. In particular, fine-grained named entity recognition is more challenging than traditional named entity recognition tasks, mainly because fine-grained tasks have higher requirements for the ability of automatic feature extraction and information representation of deep neural models. In this paper, we propose an innovative neural network model named En2BiLSTM-CRF to improve the effect of fine-grained Chinese entity recognition tasks. This proposed model including the initial encoding layer, the enhanced encoding layer, and the decoding layer combines the advantages of pre-training model encoding, dual bidirectional long short-term memory (BiLSTM) networks, and a residual connection mechanism. Hence, it can encode information multiple times and extract contextual features hierarchically. We conducted sufficient experiments on two representative datasets using multiple important metrics and compared them with other advanced baselines. We present promising results showing that our proposed En2BiLSTM-CRF has better performance as well as better generalization ability in both fine-grained and coarse-grained Chinese entity recognition tasks.
更多查看译文
关键词
neural network,information extraction,deep learning,fine-grained,NER,NLP
AI 理解论文
溯源树
样例
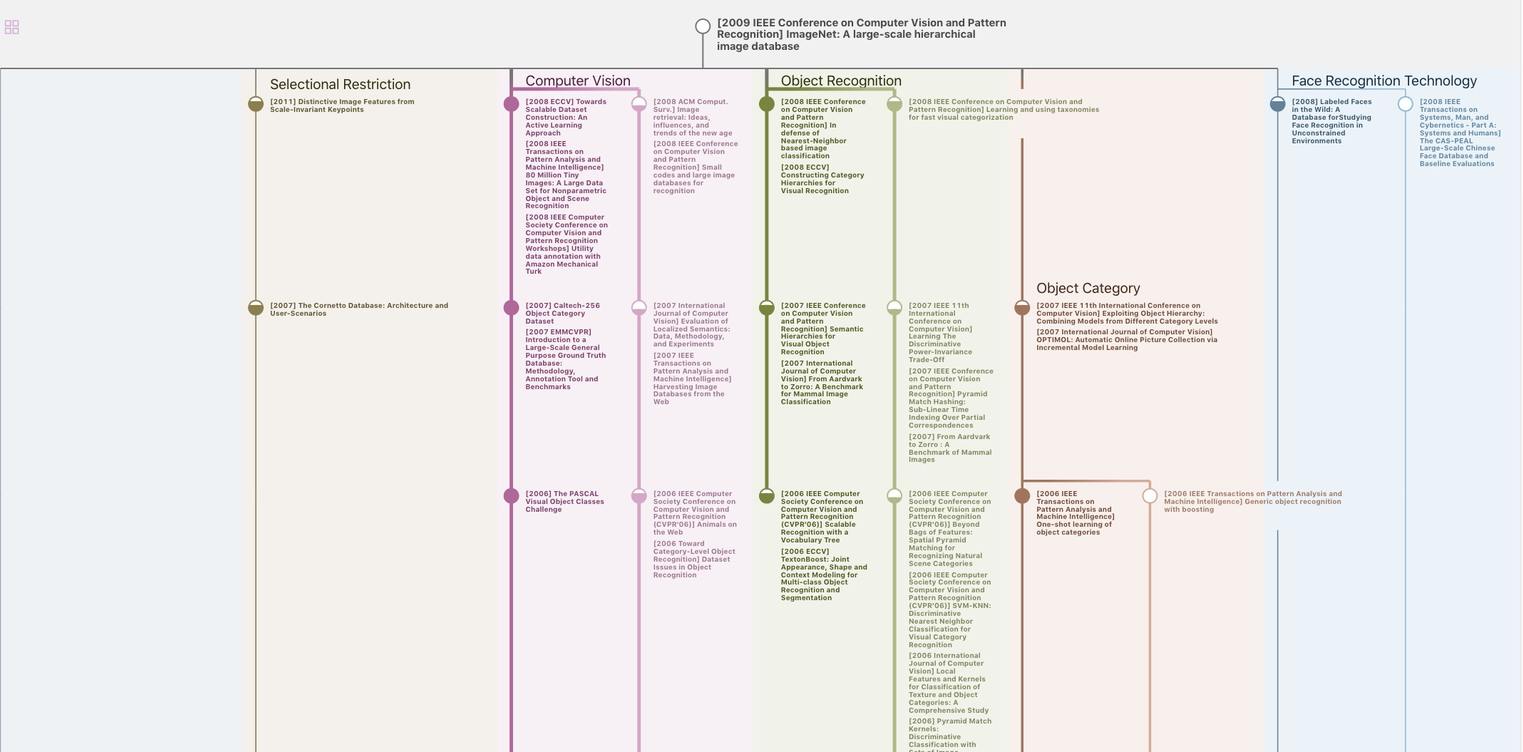
生成溯源树,研究论文发展脉络
Chat Paper
正在生成论文摘要