Fri0044 robust analyses for radiographic progression in rheumatoid arthritis
Annals of the Rheumatic Diseases(2020)
摘要
Background: Reducing structural damage is an important treatment goal for rheumatoid arthritis (RA). Demonstrating a clinically meaningful, statistically significant difference in radiographic progression (assessed by van der Heijde modified total Sharp score, mTSS) is a common objective in trials for RA treatments. Complete collection of radiographic data is challenging, especially in long term follow-up and pediatric studies. Therefore, scores for individual joints or entire patients are regularly missing. A frequently used analysis method for mTSS is the analysis of covariance model, in which missing data are imputed using linear extrapolation (ANCOVA+LE). However, other ways to deal with missing information have also been proposed. Objectives: To evaluate robust analysis methods for mTSS data. Methods: Simulated data were used to compare a random coefficient model (RC) without imputation, ANCOVA+LE and ANCOVA with last observation carry forward imputation (LOCF). A log-normal distribution was used to generate baseline patient level data to simulate a 2-arm clinical trial using baseline mTSS and rate of change in mTSS from recently completed trials. Changes in mTSS (12, 28 and 44 week timepoints) were generated under linear, concave quadratic (fast progression then slow progression), and convex quadratic (slow progression then fast progression) assumptions, with the proportion of change forced to be 0 (a proportion of simulated patients do not have progression). A monotone missing pattern was assumed to generate a data set with missing data (the ‘observed’ dataset). ANCOVA analyses were performed using baseline and treatment as predictors. The RC model was applied using baseline, treatment, time, and time-by-treatment interactions as fixed effect and time as a random effect. Bias (difference between average of simulation sample mean and true value, the smaller the better), root mean square error (RMSE, a measure of variation among simulation samples, the smaller the better), power and type I error rate were compared between methods. Results: The random coefficient model provided better or at least similar results in bias, RMSE, power and type I error rate as ANCOVA+LE under evaluated scenarios (Table 1). Conclusion: RC is a robust analysis method for mTSS. We recommend its use in primary analyses, especially for long-term extension and pediatric studies with a higher likelihood of missing data. This method can also provide reference for time points when no data are collected via estimated slope. ANCOVA+LE can be used for sensitivity analysis. References: None. Disclosure of Interests: Robert B.M. Landewe Consultant of: AbbVie; AstraZeneca; Bristol-Myers Squibb; Eli Lilly & Co.; Galapagos NV; Novartis; Pfizer; UCB Pharma, Luna Sun Shareholder of: Eli Lilly and Company, Employee of: Eli Lilly and Company, Yun-Fei Chen Shareholder of: Own shares in Eli Lilly and Company., Employee of: Employee of Eli Lilly and Company, Douglas Schlichting Shareholder of: Eli Lilly and Company, Employee of: Eli Lilly and Company, Desiree van der Heijde Consultant of: AbbVie, Amgen, Astellas, AstraZeneca, BMS, Boehringer Ingelheim, Celgene, Cyxone, Daiichi, Eisai, Eli-Lilly, Galapagos, Gilead Sciences, Inc., Glaxo-Smith-Kline, Janssen, Merck, Novartis, Pfizer, Regeneron, Roche, Sanofi, Takeda, UCB Pharma; Director of Imaging Rheumatology BV
更多查看译文
AI 理解论文
溯源树
样例
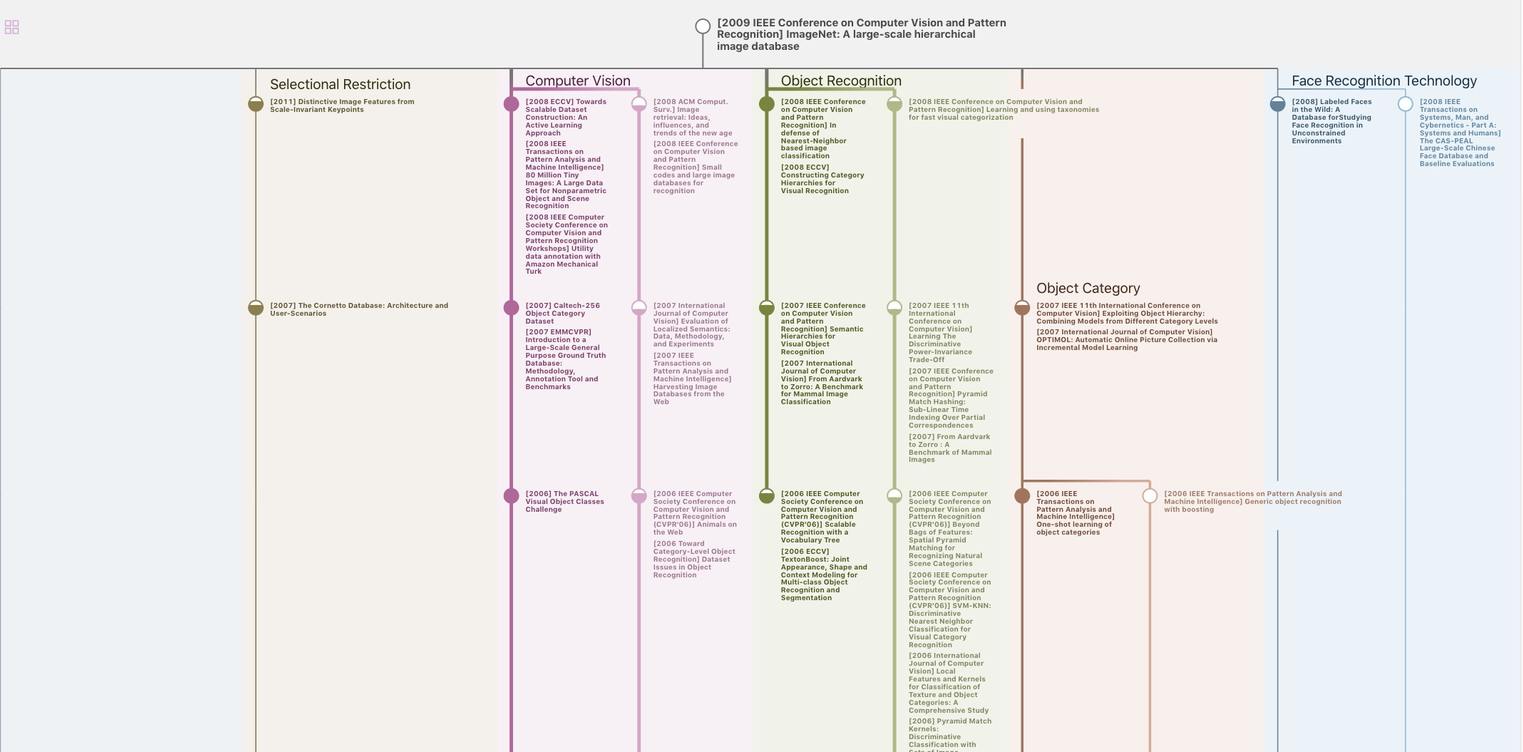
生成溯源树,研究论文发展脉络
Chat Paper
正在生成论文摘要