A General Approach for Supporting Time Series Matching Using Multiple-Warped Distances
IEEE Transactions on Knowledge and Data Engineering(2022)
摘要
Time series are generated at an unprecedented rate in domains ranging from finance, medicine to education. Collections composed of heterogeneous, variable-length and misaligned times series are best explored using a plethora of dynamic time warping distances. However, the computational costs of using such elastic distances result in unacceptable response times. We thus design the first practical solution for the efficient
GEN
eral
EX
ploration of time series leveraging multiple warped distances. GENEX pre-processes time series data in metric point-wise distance spaces, while providing bounds for the accuracy of corresponding analytics derived in non-metric warped distance spaces. Our empirical evaluation on 66 benchmark datasets provides a comparative study of the accuracy and response times of diverse warped distances. We show that GENEX is a versatile yet highly efficient solution for processing expensive-to-compute warped distances over large datasets, with response times 3 to 5 orders of magnitude faster than state-of-art systems.
更多查看译文
关键词
Time series mining,dynamic time warping,similarity exploration
AI 理解论文
溯源树
样例
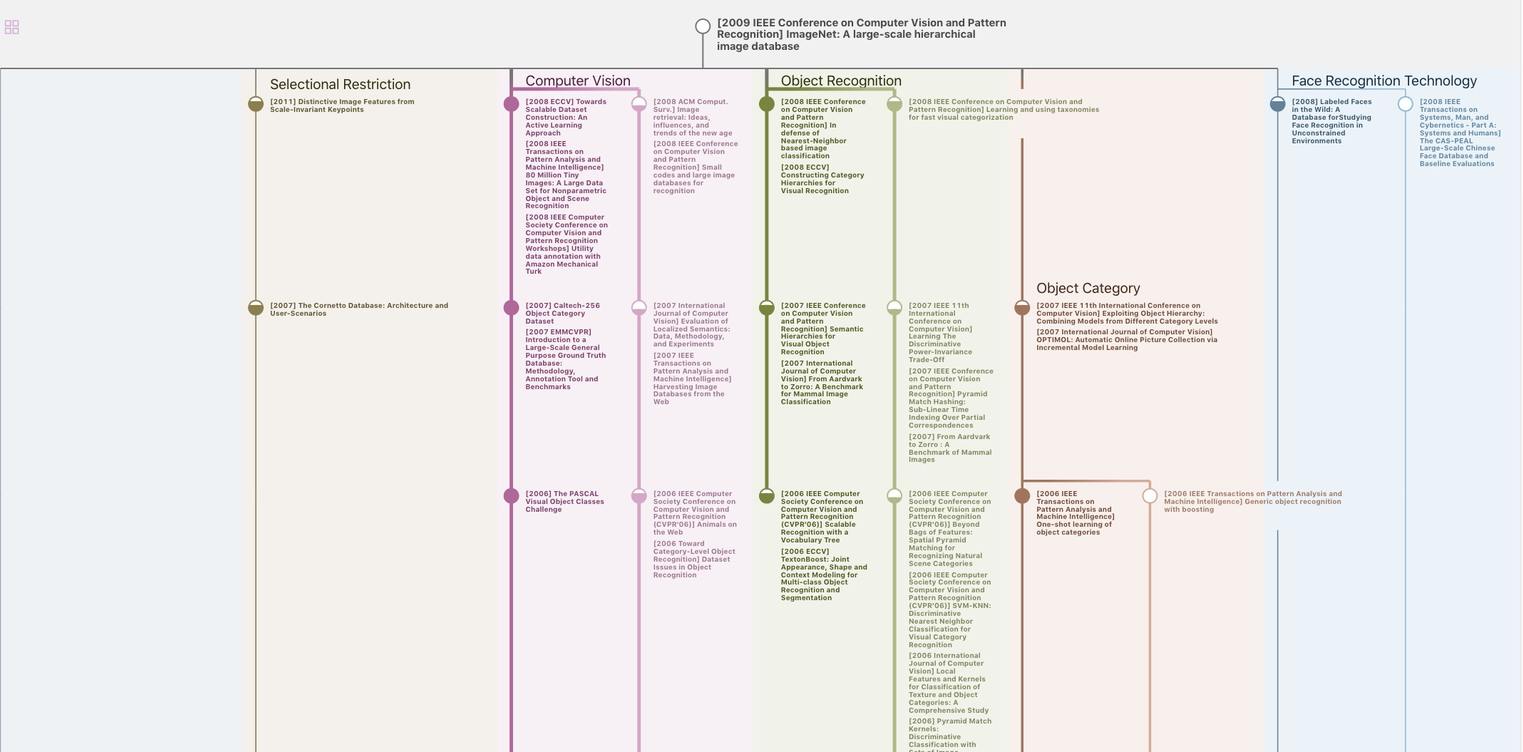
生成溯源树,研究论文发展脉络
Chat Paper
正在生成论文摘要