Discriminating tree species at different taxonomic levels using multi-temporal WorldView-3 imagery in Washington D.C., USA
Remote Sensing of Environment(2020)
摘要
Abstract With the promise of transformative changes for the management of rural and urban forests, the discrimination of tree species from satellite imagery has been a long-standing goal of remote sensing. For the species-rich urban setting of Washington, D.C. USA, we evaluate current prospects toward this goal by combining a Random Forest (RF) object-based tree species classification method with two large datasets 1) A suite of 12 very high resolution (VHR) WorldView-3 images (WV-3), whose image acquisition date cover each pheno-phase of the growing season from April to November; and 2) the 16,496 street trees from Washington D.C. Department of Transportation\u0027s (DDOT) field inventory. We classify the 19 most abundant tree species with an overall accuracy of 61.3% and classify the ten most abundant genera with an overall accuracy of 73.7%. We observe that (1) there are larger declines in accuracy when attempting to classify species in the same genus, and (2) the most valuable phenological period is fall senescence for classification at different taxonomic levels. Especially if satellite data can be matched to the key pheno-phases, our study highlights that current VHR satellite sensors now have the radiometric, spectral, and spatial resolution to potentially help manage species-rich urban forests.
更多查看译文
关键词
WorldView3,Tree species,Phenology,Washington D.C.,Street trees,Urban
AI 理解论文
溯源树
样例
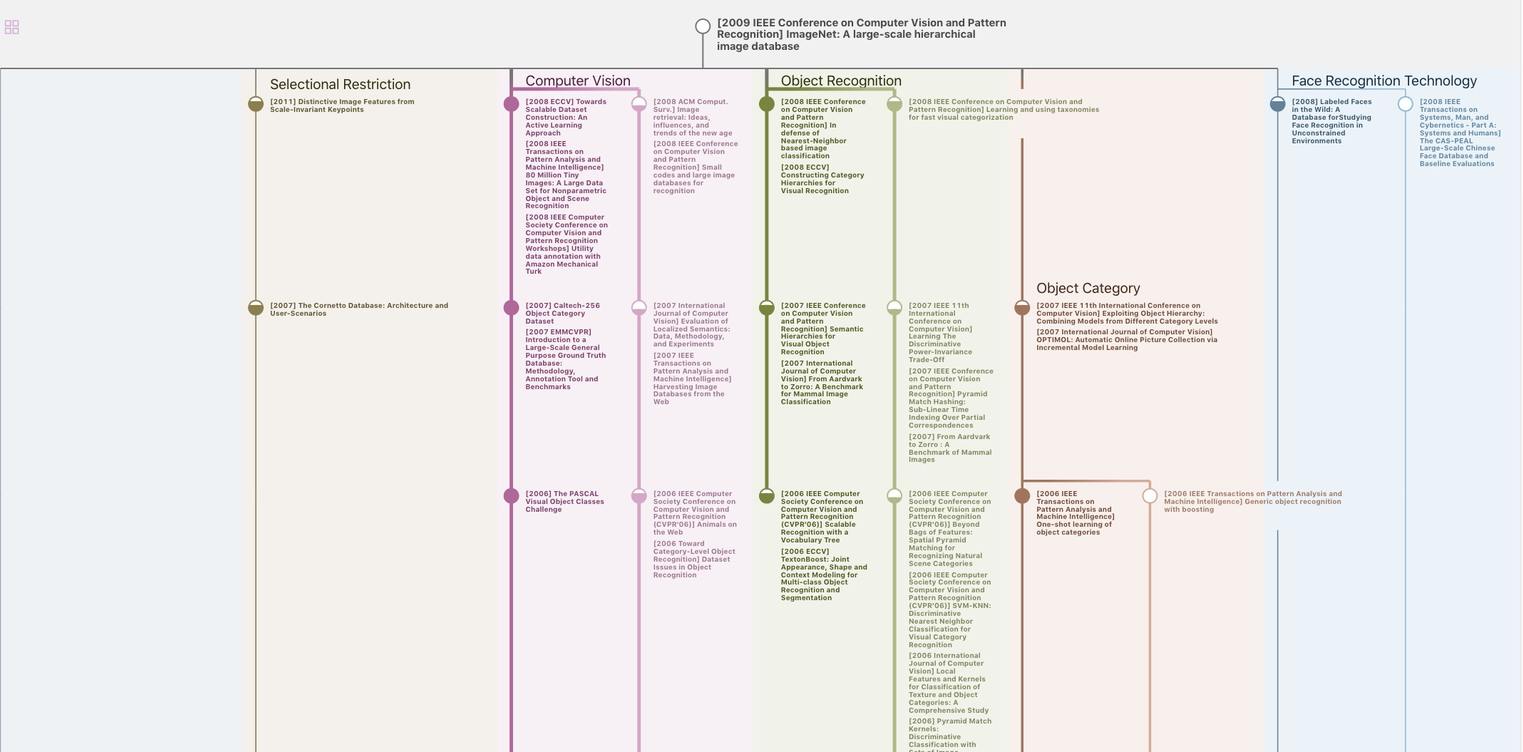
生成溯源树,研究论文发展脉络
Chat Paper
正在生成论文摘要