Benchmarking Q-Learning Methods for Intelligent Network Orchestration in the Edge
2020 2nd 6G Wireless Summit (6G SUMMIT)(2020)
摘要
We benchmark Q-learning methods, with various action selection strategies, in intelligent orchestration of the network edge. Q-learning is a reinforcement learning technique that aims to find optimal action policies by taking advantage of the experiences in the past without utilizing a model that describes the dynamics of the environment. With experiences, we refer to the observed causality between the action and the corresponding impact to the environment. In this paper, the environment for Q-learning is composed of virtualized networking resources along with their dynamics that are monitored with Spindump, an in-network latency measurement tool with support for QUIC and TCP. We optimize the orchestration of these networking resources by introducing Q-learning as part of the machine learning driven, intelligent orchestration that is applicable in the edge. Based on the benchmarking results, we identify which action selection strategies support network orchestration that provides low latency and packet loss by considering network resource allocation in the edge.
更多查看译文
关键词
Q-learning,edge,intelligent orchestration
AI 理解论文
溯源树
样例
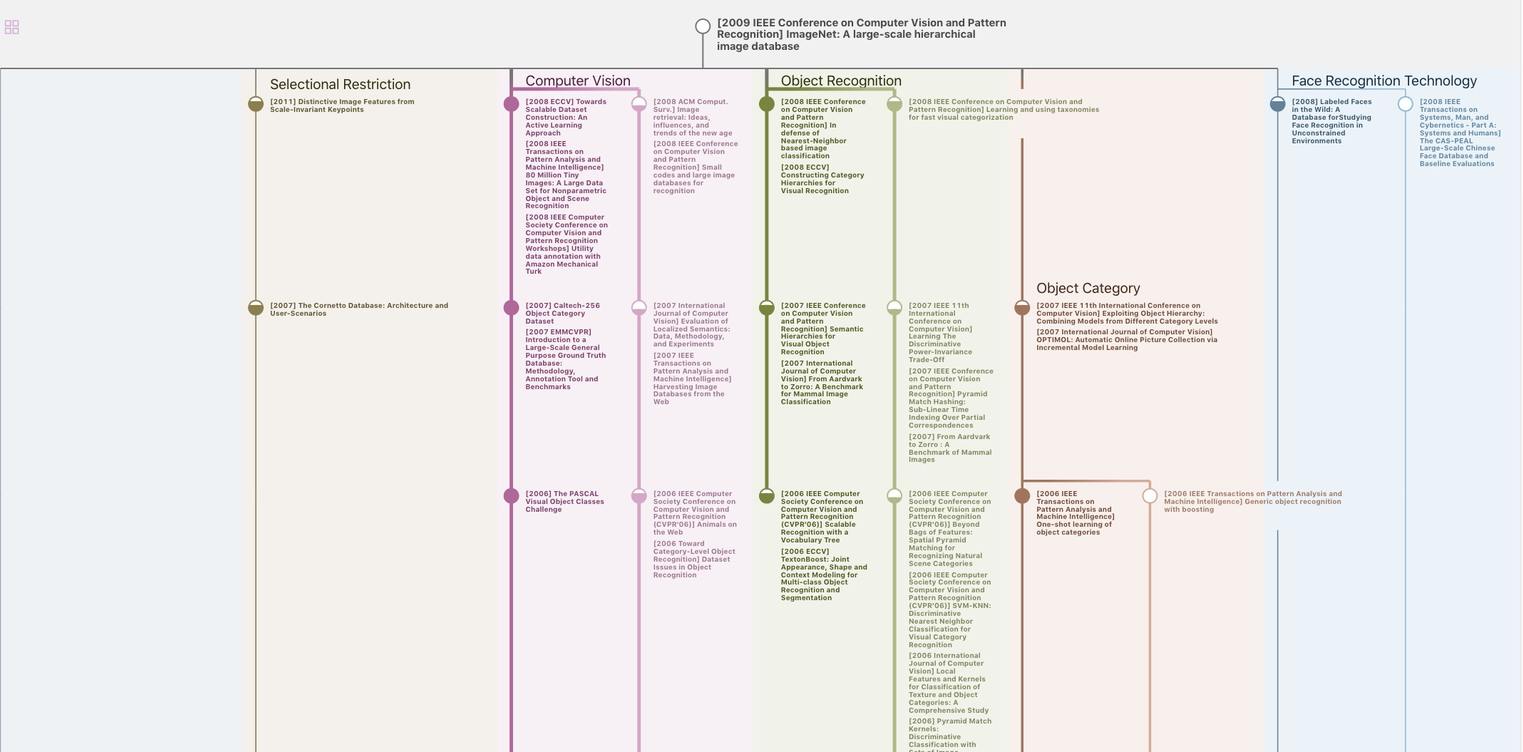
生成溯源树,研究论文发展脉络
Chat Paper
正在生成论文摘要