Learning Discriminative Neural Sentiment Units for Semi-supervised Target-Level Sentiment Classification.
PAKDD (2)(2020)
摘要
Target-level sentiment classification aims at assigning sentiment polarities to opinion targets in a sentence, for which it is significantly more challenging to obtain large-scale labeled data than sentence/document-level sentiment classification due to the intricate contexts and relations of the target words. To address this challenge, we propose a novel semi-supervised approach to learn sentiment-aware representations from easily accessible unlabeled data specifically for the fine-grained sentiment learning. This is very different from current popular semi-supervised solutions that use the unlabeled data via pretraining to generate generic representations for various types of downstream tasks. Particularly, we show for the first time that we can learn and detect some highly sentiment-discriminative neural units from the unsupervised pretrained model, termed neural sentiment units. Due to the discriminability, these sentiment units can be leveraged by downstream LSTM-based classifiers to generate sentiment-aware and context-dependent word representations to substantially improve their sentiment classification performance. Extensive empirical results on two benchmark datasets show that our approach (i) substantially outperforms state-of-the-art sentiment classifiers and (ii) achieves significantly better data efficiency.
更多查看译文
关键词
discriminative neural sentiment units,classification,learning,semi-supervised,target-level
AI 理解论文
溯源树
样例
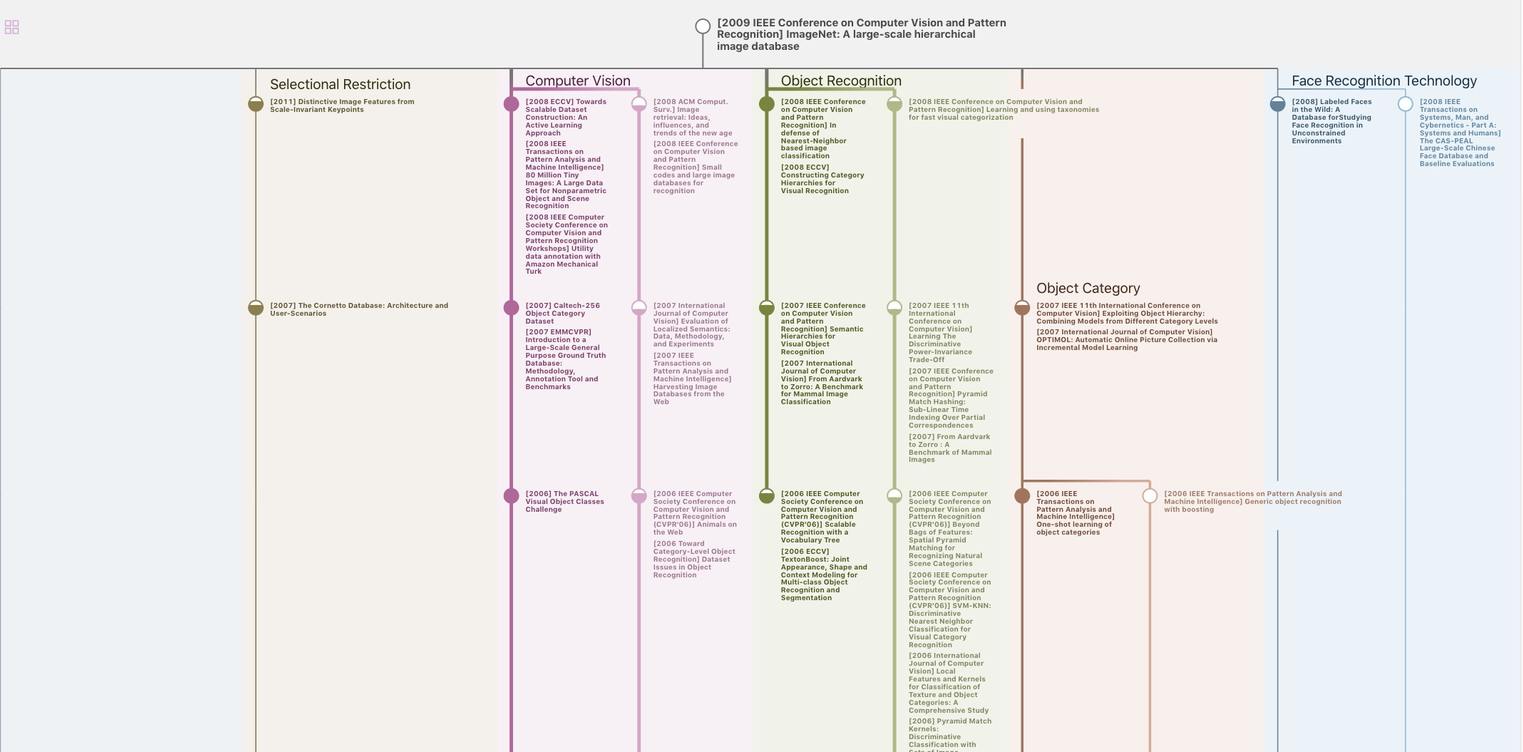
生成溯源树,研究论文发展脉络
Chat Paper
正在生成论文摘要