Large-scale scene image categorisation with deep learning-based model
International Journal of Computational Vision and Robotics(2020)
摘要
Increasing depth of convolutional neural networks (CNNs) is a highly promising method of increasing the accuracy of the (CNNs). Increased CNN depth will also result in increased layer count (parameters), leading to a slow backpropagation convergence prone to overfitting. We trained our model (residual-CNDS) to classify very large-scale scene datasets. The outcome result from the two datasets proved our proposed model effectively handled the slow convergence, overfitting, and degradation. Our approach overcomes degradation in the very deep network. We have built two models (residual-CNDS 8), and (residual-CNDS 10). Moreover, we tested our models on two large-scale datasets, and we compared our results with other recently introduced cutting-edge networks in the domain of top-1 and top-5 classification accuracy. As a result, both of models have shown good improvement, which supports the assertion that the addition of residual connections enhances network CNDS accuracy without adding any computation complexity.
更多查看译文
关键词
residual-CNDS,scene classification,residual learning convolutional neural networks to residual learning,convolutional networks with deep supervision
AI 理解论文
溯源树
样例
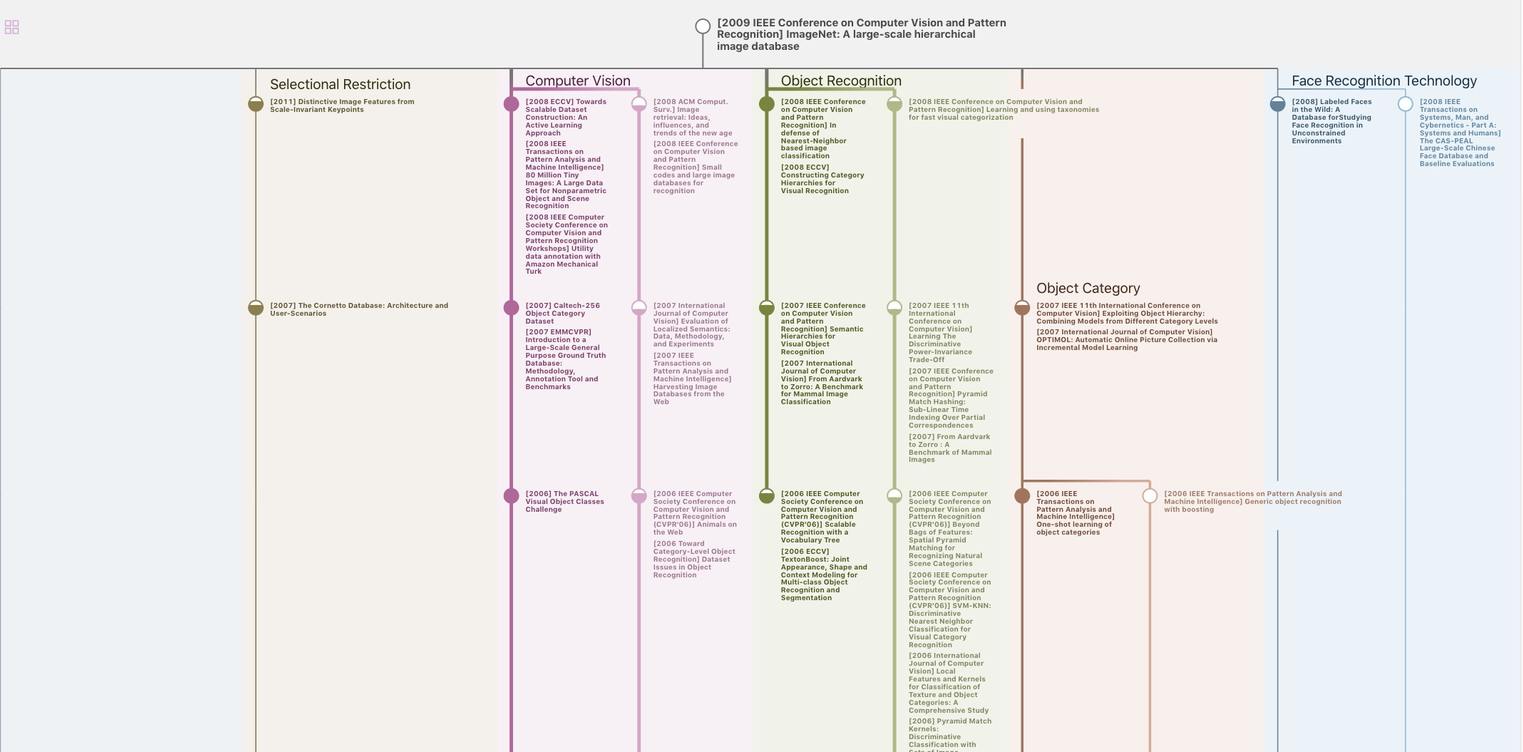
生成溯源树,研究论文发展脉络
Chat Paper
正在生成论文摘要