Visual Sentiment Analysis With Social Relations-Guided Multiattention Networks
IEEE Transactions on Cybernetics(2022)
摘要
These days, social media users tend to express their feelings through sharing images online. Capturing the emotions embedded in these social images involves great research challenges and practical values. Most existing works concentrate on extracting the visual feature from a global view, while ignoring the fact that visual objects are also rich in emotion. How to leverage the multilevel visual features to improve the sentiment analysis performance is important yet challenging. Besides, existing works view each social image as an independent sample while ignoring the rich correlations among social images, which may be helpful in detecting visual emotion. In this article, we propose a novel model called social relations-guided multiattention networks (SRGMANs) to incorporate both the multilevel (region-level and object-level) visual features of a single image and the correlations among multiple social images to conduct visual sentiment analysis. Specifically, we first construct a heterogeneous network consisting of various types of social relations and introduce a heterogeneous network embedding method to learn the network representation for each image. Then, two visual attention branches (region attention network and object attention network) are devised to extract emotional and discriminative visual features. For each branch, we design a self-attention module to capture the emotional dependencies among visual parts. Besides, a network-guided attention module is also designed in each branch to focus on more network-related emotional visual parts with the guidance of the topology information. Finally, the attended visual features from the two attention models, together with network representation features, are combined within a holistic framework to predict the sentiment of social images. Extensive experiments demonstrate the superiority of our model on three benchmark datasets.
更多查看译文
关键词
Emotions,Humans,Learning,Sentiment Analysis,Social Media,Social Networking
AI 理解论文
溯源树
样例
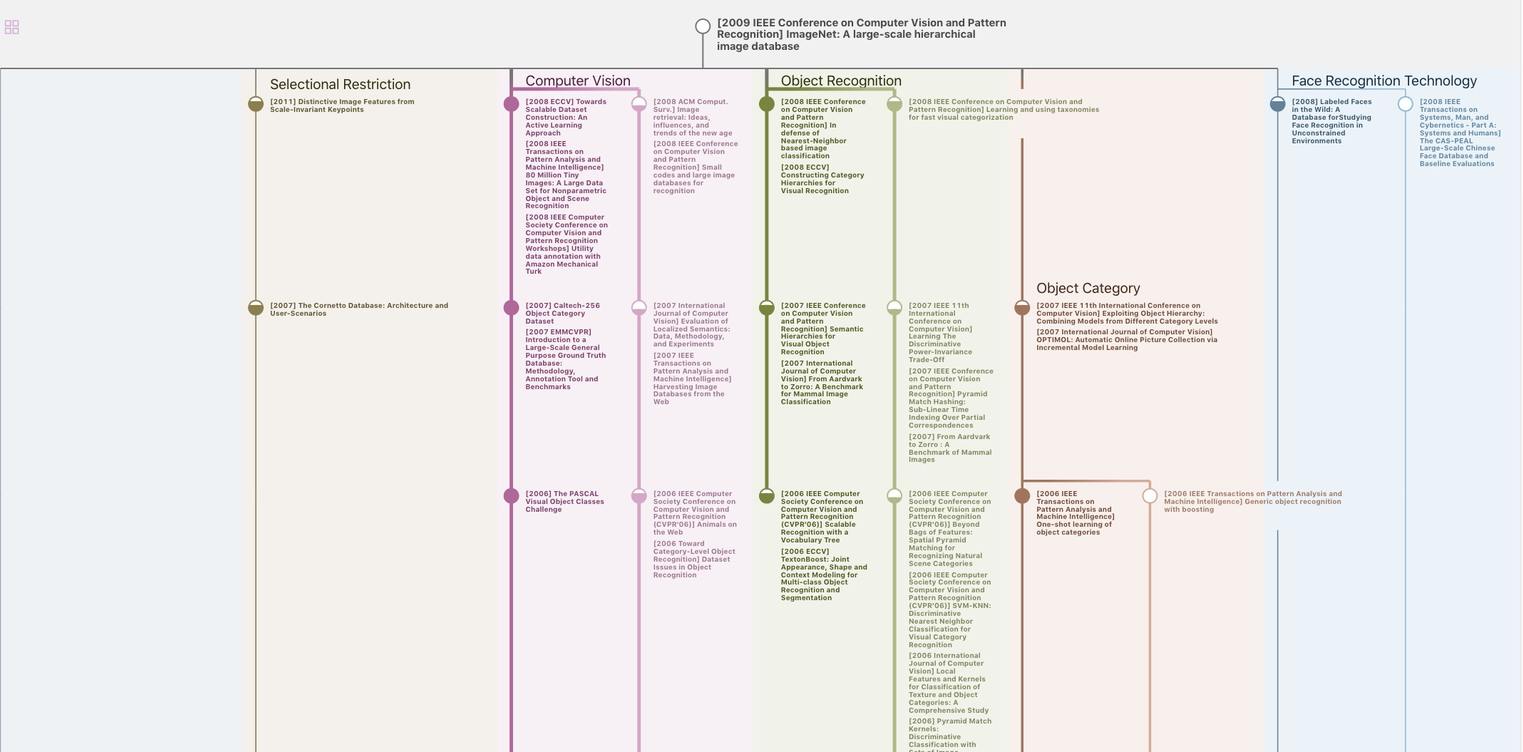
生成溯源树,研究论文发展脉络
Chat Paper
正在生成论文摘要