Site-scale modeling of surface ozone in Northern Bavaria using machine learning algorithms, regional dynamic models, and a hybrid model
Environmental Pollution(2021)
摘要
Ozone (O3) is a harmful pollutant when present in the lowermost layer of the atmosphere. Therefore, the European Commission formulated directives to regulate O3 concentrations in near-surface air. However, almost 50% of the 5068 air quality stations in Europe do not monitor O3 concentrations. This study aims to provide a hybrid modeling system that fills these gaps in the hourly surface O3 observations on a site scale with much higher accuracy than existing O3 models. This hybrid model was developed using estimations from multiple linear regression-based eXtreme Gradient Boosting Machines (MLR-XGBM) and O3 reanalysis from European regional air quality models (CAMS-EU). The binary classification of extremely high O3 events and the 1- and 24-h forecasts of hourly O3 were investigated as secondary aims. In this study thirteen stations in Northern Bavaria, out of which six do not monitor O3, were chosen as test sites. Considering the computational complexity of machine learning algorithms (MLAs), we also applied two recent MLA interpretation methods, namely SHapley Additive exPlanations (SHAP) and Local interpretable model-agnostic explanations (LIME).
更多查看译文
关键词
Downscaling,Surface ozone,Ensemble learning,Simulation interpretability
AI 理解论文
溯源树
样例
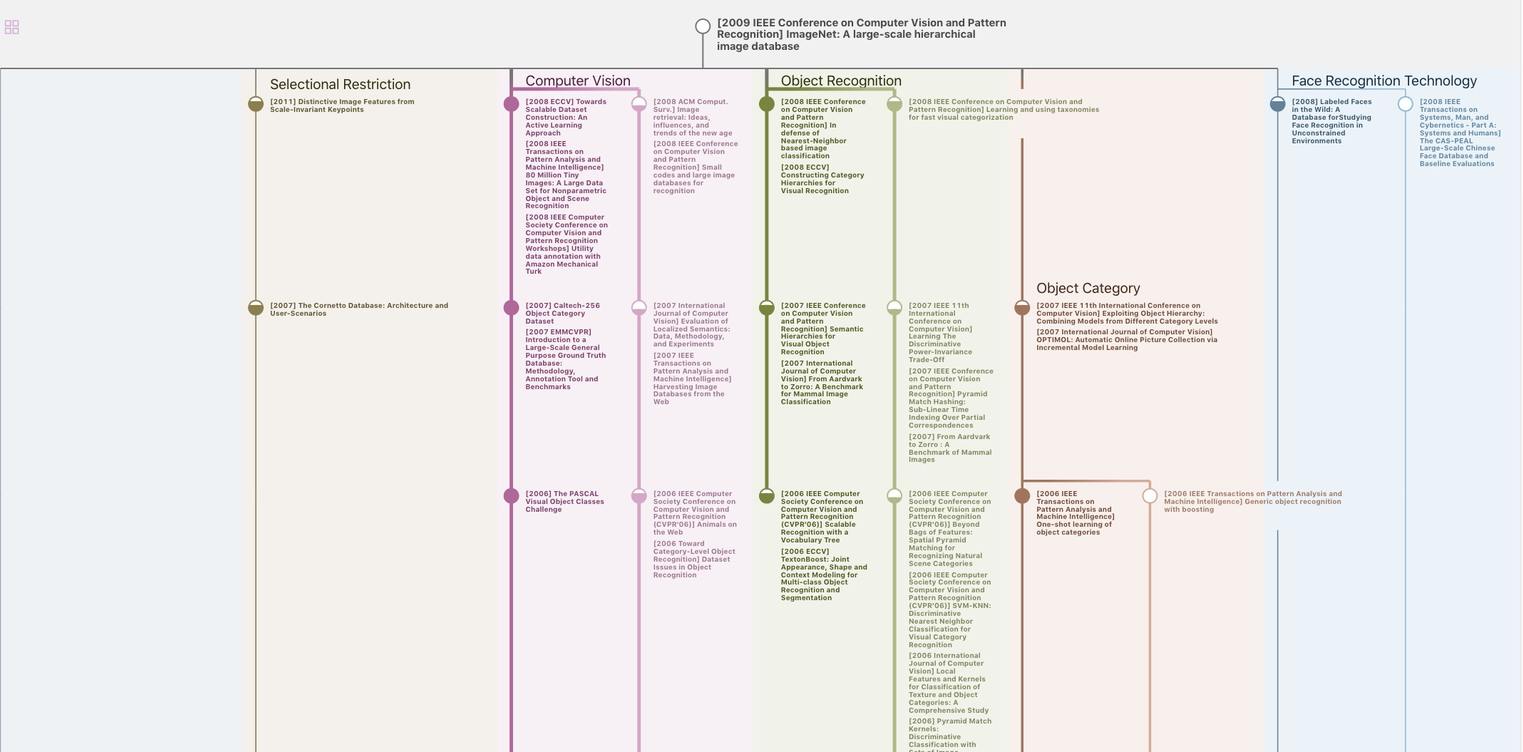
生成溯源树,研究论文发展脉络
Chat Paper
正在生成论文摘要