Self-supervised heterogeneous graph learning with iterative similarity distillation
Knowledge-Based Systems(2023)
摘要
This paper focuses on the self-supervised learning issue on heterogeneous graph. Currently, contrastive learning has become the dominant approach on various heterogeneous graph tasks. Its performance is highly dependent on the elaborate selection strategy of negative samples. However, it is difficult to design such an ideal strategy giving sufficient consideration to the characteristics of graph, such as structural similarity and feature similarity. To circumvent this problem, we propose a novel negative sample-free self-supervised framework named HGISD (Heterogeneous Graph learning based on Iterative Similarity Distillation), where the student improves itself by imitating the teacher’s behavior and the teacher updates itself as a slow-moving average of the student. Moreover, metapath-based HGNN is employed as the built-in information encoder. We argue that previous metapath-based HGNNs neglect the correlation among metapaths and the locality within metapaths. To tackle these issues, we respectively propose IMCD (Inter-Metapath Correlation Distillation) and IMSD (Intra-Metapath Similarity Distillation) within the self-distillation framework. Both IMCD and IMSD treat the embeddings in the shallow layers as the knowledge source, which well preserves the inter-metapath correlation and the intra-metapath locality. Finally, we conduct extensive experiments on several real-world heterogeneous graph datasets. The results show that the proposed HGISD achieves superior performance compared with the state-of-the-art methods.
更多查看译文
关键词
Heterogeneous graph neural network,Iterative similarity distillation,Self-distillation,Metapath correlation
AI 理解论文
溯源树
样例
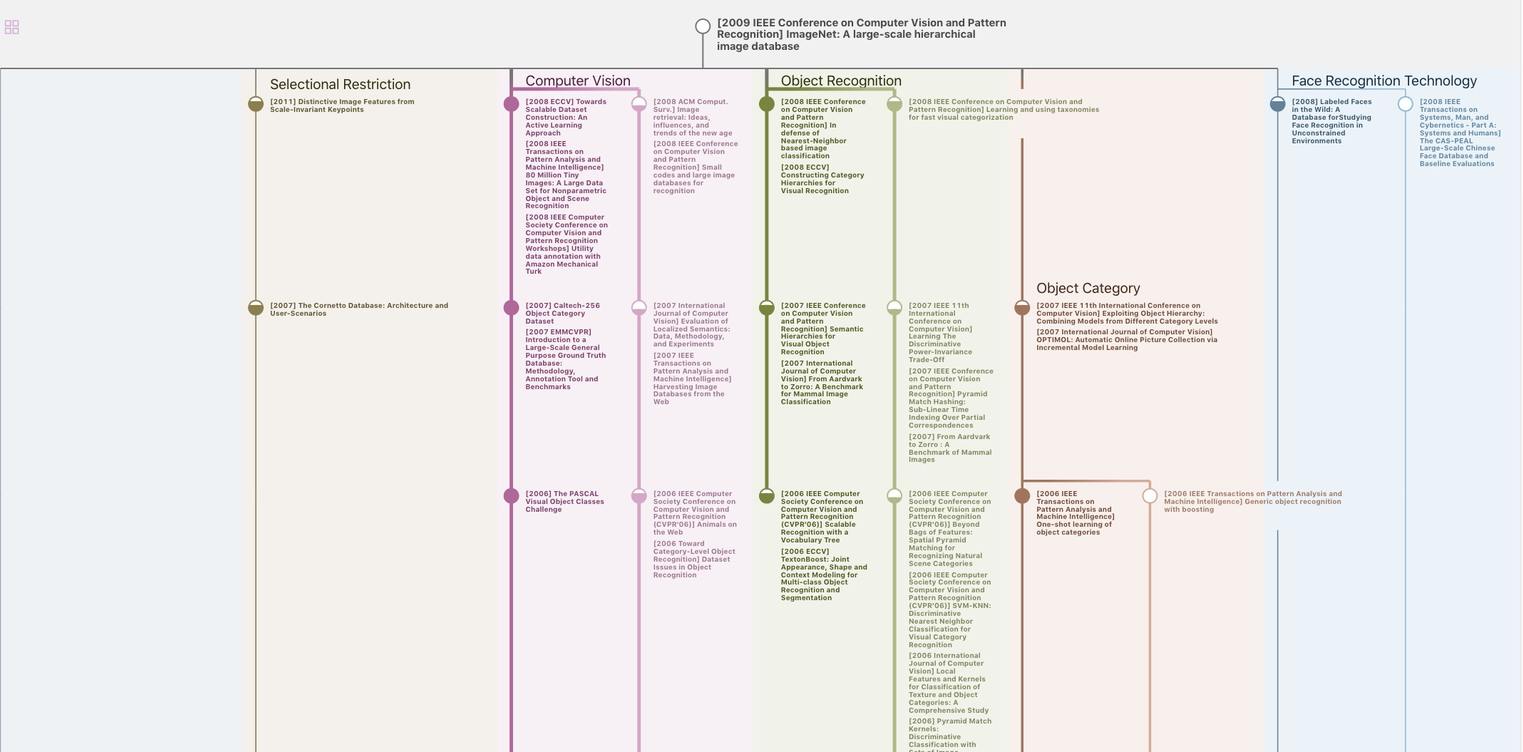
生成溯源树,研究论文发展脉络
Chat Paper
正在生成论文摘要