Practical Bayesian Optimization over Sets
arXiv preprint arXiv:1905.09780(2019)
摘要
We propose a practical Bayesian optimization method over sets, to minimize a black-box function that can take a set as a single input. Because set inputs are permutation-invariant and variable-length, traditional Gaussian process-based Bayesian optimization strategies which assume vector inputs can fall short. To address this, we develop a Bayesian optimization method with\emph {set kernel} that is used to build surrogate functions. This kernel accumulates similarity over set elements to enforce permutation-invariance and permit sets of variable size, but this comes at a greater computational cost. To reduce this burden, we propose two key components:(i) a more efficient probabilistic approximation which is still positive-definite and is an unbiased estimator of the true set kernel with upper-bounded variance in terms of the number of subsamples,(ii) a constrained acquisition function optimization over sets, which uses symmetry of the feasible region that defines a set input. Finally, we present several numerical experiments which demonstrate that our method outperforms other methods in various applications.
更多查看译文
AI 理解论文
溯源树
样例
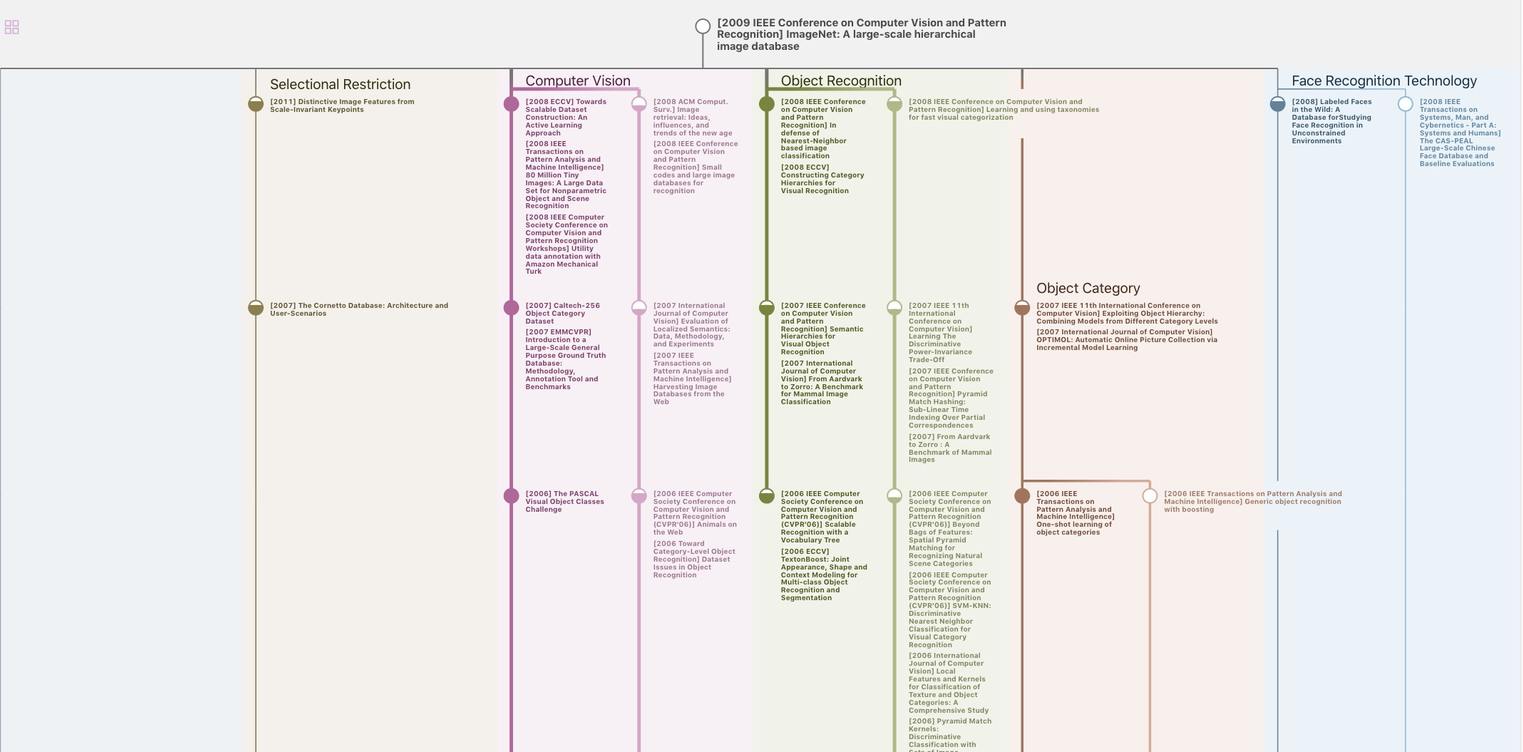
生成溯源树,研究论文发展脉络
Chat Paper
正在生成论文摘要