Performance and Robustness of Machine Learning based Radiomic COVID 19 Severity Prediction
medRxiv(2020)
摘要
Objectives This study investigated the performance and robustness of radiomics in predicting COVID-19 severity in a large public cohort.
Methods A public dataset of 1110 COVID-19 patients (1 CT/patient) was used. Using CTs and clinical data, each patient was classified into mild, moderate, and severe by two observers: (1) dataset provider and (2) a board-certified radiologist. For each CT, 107 radiomic features were extracted. The dataset was randomly divided into a training (60%) and holdout validation (40%) set. During training, features were selected and combined into a logistic regression model for predicting severe cases from mild and moderate cases. The models were trained and validated on the classifications by both observers. AUC quantified the predictive power of models. To determine model robustness, the trained models was cross-validated on the inter-observer’s classifications.
Results A single feature alone was sufficient to predict mild from severe COVID-19 with ![Graphic][1] and ![Graphic][2] (p<< 0.01). The most predictive features were the distribution of small size-zones (GLSZM-SmallAreaEmphasis) for provider’s classification and linear dependency of neighboring voxels (GLCM-Correlation) for radiologist’s classification. Cross-validation showed that both ![Graphic][3]. In predicting moderate from severe COVID-19 , first-order-Median alone had sufficient predictive power of ![Graphic][4]. For radiologist’s classification, the predictive power of the model increased to ![Graphic][5] as the number of features grew from 1 to 5. Cross-validation yielded ![Graphic][6] and ![Graphic][7].
Conclusions Radiomics significantly predicted different levels of COVID-19 severity. The prediction was moderately sensitive to inter-observer classifications, and thus need to be used with caution.
Key points
### Competing Interest Statement
S.S.F.Y. and R.J. of this manuscript declare relationships with AIQ Solutions Inc: S.S.F.Y. is an employee and a shareholder of AIQ Solutions, Inc. R.J. is a consultant and shareholder of AIQ Solutions, Inc.
### Funding Statement
The authors state that this work has not received any funding.
### Author Declarations
I confirm all relevant ethical guidelines have been followed, and any necessary IRB and/or ethics committee approvals have been obtained.
Yes
The details of the IRB/oversight body that provided approval or exemption for the research described are given below:
Institutional Review Board approval was not required because public MosMedData dataset was used. This public dataset is freely available for scientific research on https://mosmed.ai
All necessary patient/participant consent has been obtained and the appropriate institutional forms have been archived.
Yes
I understand that all clinical trials and any other prospective interventional studies must be registered with an ICMJE-approved registry, such as ClinicalTrials.gov. I confirm that any such study reported in the manuscript has been registered and the trial registration ID is provided (note: if posting a prospective study registered retrospectively, please provide a statement in the trial ID field explaining why the study was not registered in advance).
Yes
I have followed all appropriate research reporting guidelines and uploaded the relevant EQUATOR Network research reporting checklist(s) and other pertinent material as supplementary files, if applicable.
Yes
This public dataset is freely available for scientific research on https://mosmed.ai
* COVID-19
: Coronavirus disease 2019
GLCM
: Gray level co-occurrence matrix
GLDM
: Gray level dependence matrix
GLSZM
: Gray level size zone matrix
IMC2
: Informational measure of correlation 2
ML
: Machine learning
MRMR
: Maximum relevance and minimum redundancy
RFE
: Recursive feature elimination
[1]: /embed/inline-graphic-1.gif
[2]: /embed/inline-graphic-2.gif
[3]: /embed/inline-graphic-3.gif
[4]: /embed/inline-graphic-4.gif
[5]: /embed/inline-graphic-5.gif
[6]: /embed/inline-graphic-6.gif
[7]: /embed/inline-graphic-7.gif
更多查看译文
关键词
severity,prediction,robustness,learning-based
AI 理解论文
溯源树
样例
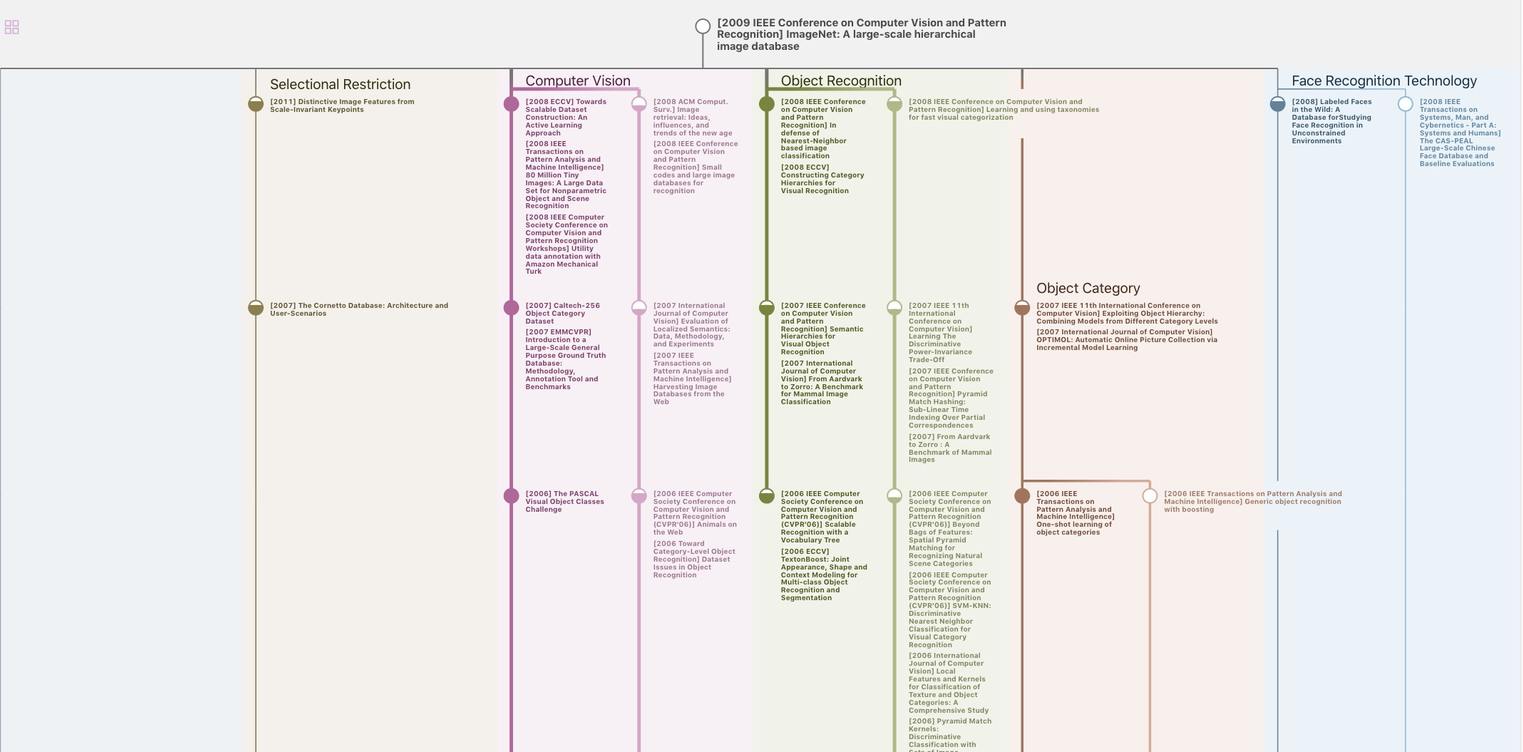
生成溯源树,研究论文发展脉络
Chat Paper
正在生成论文摘要