Equivariance For Deep Learning And Retinal Imaging
user-5f1696ff4c775ed682f5929f(2019)
摘要
Analysing and generalizing from small datasets is still a difficult challenge. However, the de facto model in computer vision, the convolutional neural network (CNN), is known to be data hungry and poorly interpretable. We investigate a new design principle called equivariance, which we demonstrate can both improve data efficiency and interpretability in CNNs. Intermediate layers of an equivariant CNN preserve information about local and global transformations of the input image. Moreover, we build specific equivariances into a CNN, such that they need not be learned from data. As a result, less data is needed, improving the network generalization. In this thesis, we explore three techniques to demonstrate the usefulness of equivariance. We start by considering equivariance to 3D right-angle rotations in the context of voxelized data. We show that this improves classification performance on the ModelNet10 dataset, among non-ensembled models. We then introduce Harmonic Networks, which are equivariant to continuous rotations in 2D. These exploit the fact that the Fourier transform naturally disentangles transformation and magnitude information. To the best of our knowledge, this is the first CNN architecture exhibiting equivariance to a continuous transformation. Lastly we consider learning equivariance from data, to model equivariances, which may not be tractably designed into a network. To validate our work, we harness a real-world case study by applying some of our developments to the difficult challenge of Retinopathy of Prematurity (ROP) detection. ROP is a sight-threatening disease, affecting 60% of premature babies below 32 …
更多查看译文
AI 理解论文
溯源树
样例
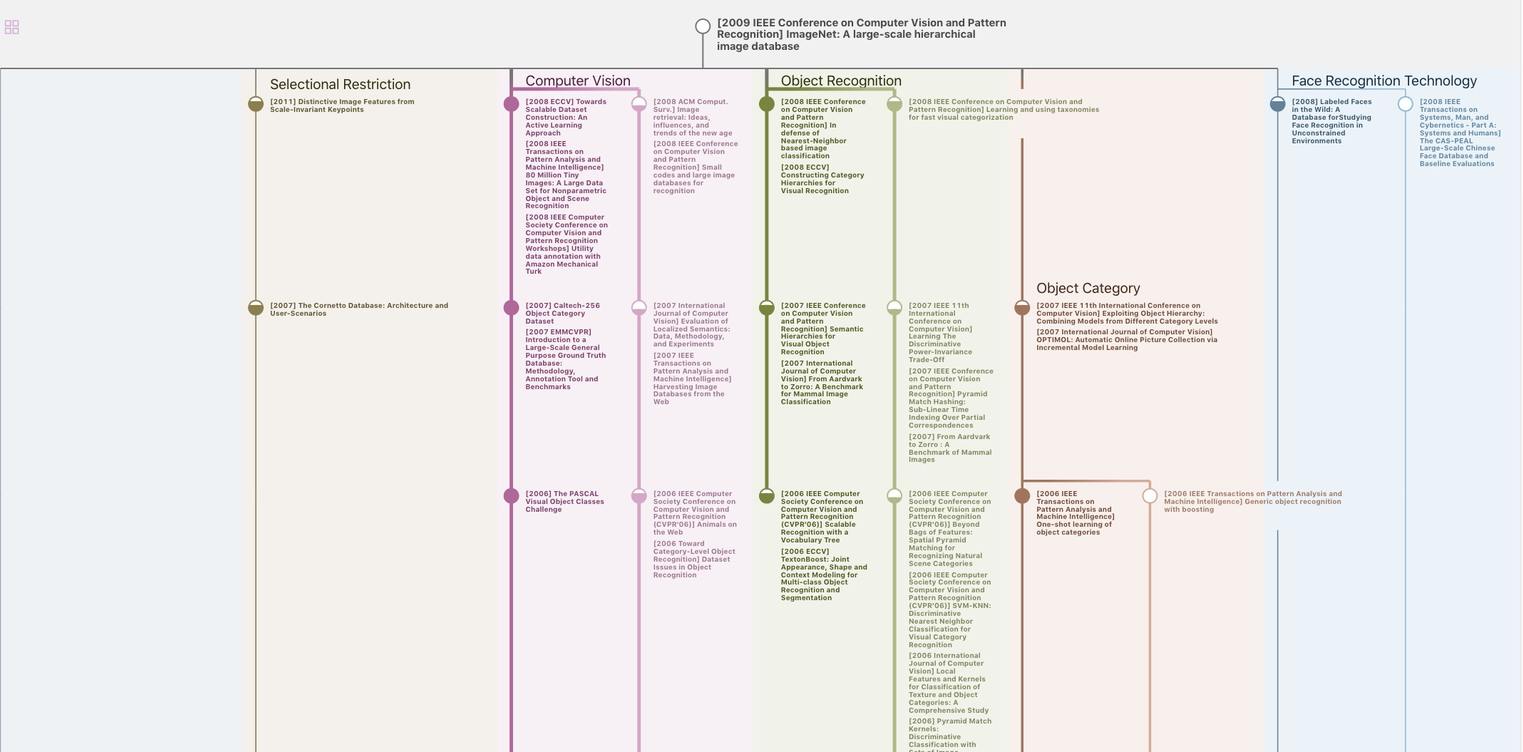
生成溯源树,研究论文发展脉络
Chat Paper
正在生成论文摘要