TPR: Text-aware Preference Ranking for Recommender Systems
CIKM '20: The 29th ACM International Conference on Information and Knowledge Management Virtual Event Ireland October, 2020(2020)
摘要
Textual data is common and informative auxiliary information for recommender systems. Most prior art utilizes text for rating prediction, but rare work connects it to top-recommendation. Moreover, although advanced recommendation models capable of incorporating auxiliary information have been developed, none of these are specifically designed to model textual information, yielding a limited usage scenario for typical user-to-item recommendation. In this work, we present a framework of text-aware preference ranking (TPR) for top- recommendation, in which we comprehensively model the joint association of user-item interaction and relations between items and associated text. Using the TPR framework, we construct a joint likelihood function that explicitly describes two ranking structures: 1) item preference ranking (IPR) and 2) word relatedness ranking (WRR), where the former captures the item preference of each user and the latter captures the word relatedness of each item. As these two explicit structures are by nature mutually dependent, we propose TPR-OPT, a simple yet effective learning criterion that additionally includes implicit structures, such as relatedness between items and relatedness between words for each user for model optimization. Such a design not only successfully describes the joint association among users, words, and text comprehensively but also naturally yields powerful representations that are suitable for a range of recommendation tasks, including user-to-item, item-to-item, and user-to-word recommendation, as well as item-to-word reconstruction. In this paper, extensive experiments have been conducted on eight recommendation datasets, the results of which demonstrate that by including textual information from item descriptions, the proposed TPR model consistently outperforms state-of-the-art baselines on various recommendation tasks.
更多查看译文
关键词
recommender systems, textual information, preference ranking
AI 理解论文
溯源树
样例
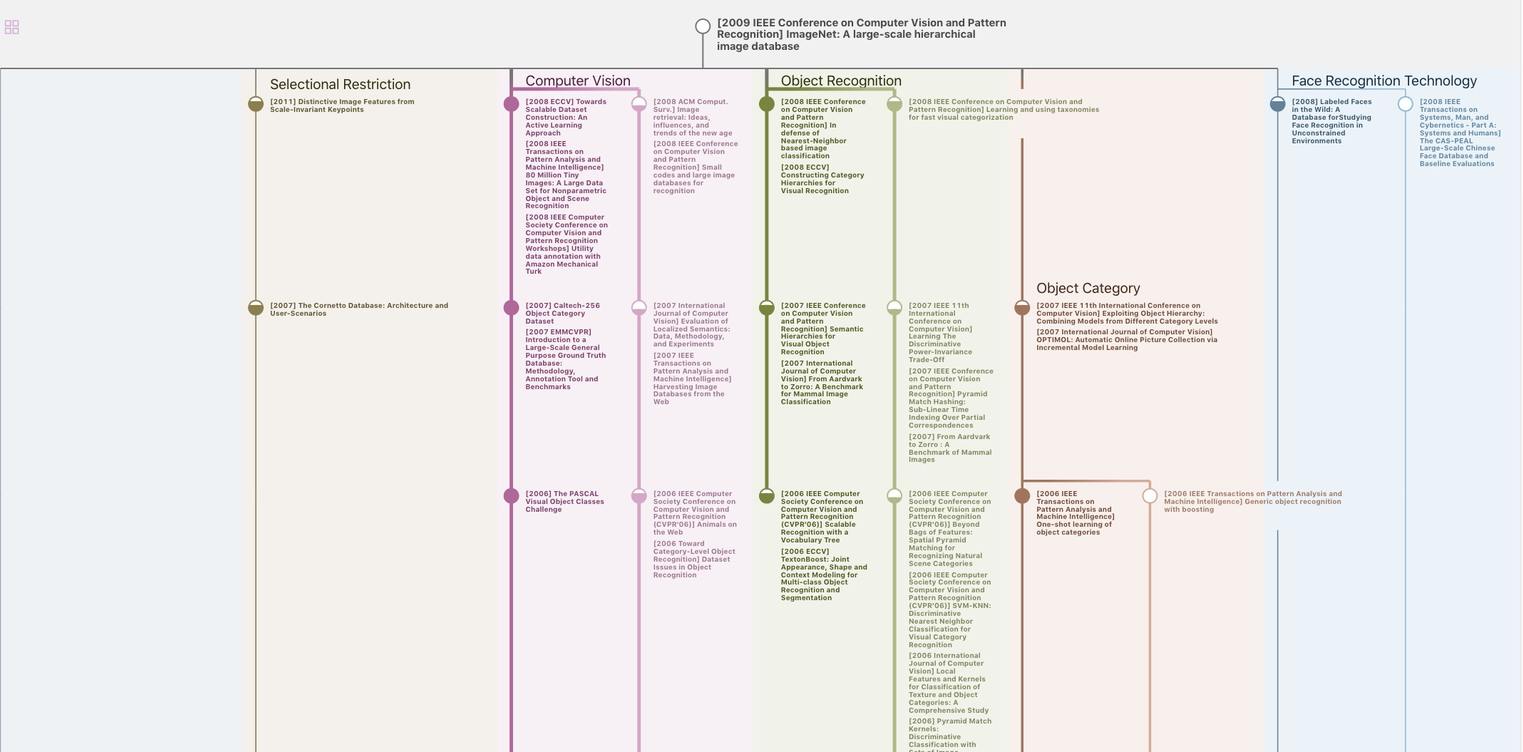
生成溯源树,研究论文发展脉络
Chat Paper
正在生成论文摘要