Box Guided Convolution for Pedestrian Detection
MM '20: The 28th ACM International Conference on Multimedia Seattle WA USA October, 2020(2020)
摘要
Occlusions, scale variation and numerous false positives still represent fundamental challenges in pedestrian detection. Intuitively, different sizes of receptive fields and more attention to the visible parts are required for detecting pedestrians with various scales and occlusion levels, respectively. However, these challenges have not been addressed well by existing pedestrian detectors. This paper presents a novel convolutional network, denoted as box guided convolution network (BGCNet), to tackle these challenges simultaneously in a unified framework. In particular, we proposed a box guided convolution (BGC) that can dynamically adjust the sizes of convolution kernels guided by the predicted bounding boxes. In this way, BGCNet provides position-aware receptive fields to address the challenge of large variations of scales. In addition, for the issue of heavy occlusion, the kernel parameters of BGC are spatially localized around the salient and mostly visible key points of a pedestrian, such as the head and foot, to effectively capture high-level semantic features to help detection. Furthermore, a local maximum (LM) loss is introduced to depress false positives and highlight true positives by forcing positives, rather than negatives, as local maximums, without any additional inference burden. We evaluate BGCNet on popular pedestrian detection benchmarks, and achieve the state-of-the-art results, with the significant performance improvement on heavily occluded and small-scale pedestrians.
更多查看译文
关键词
Pedestrian Detection, Box Guided Convolution, Receptive Fields, Scale Variation
AI 理解论文
溯源树
样例
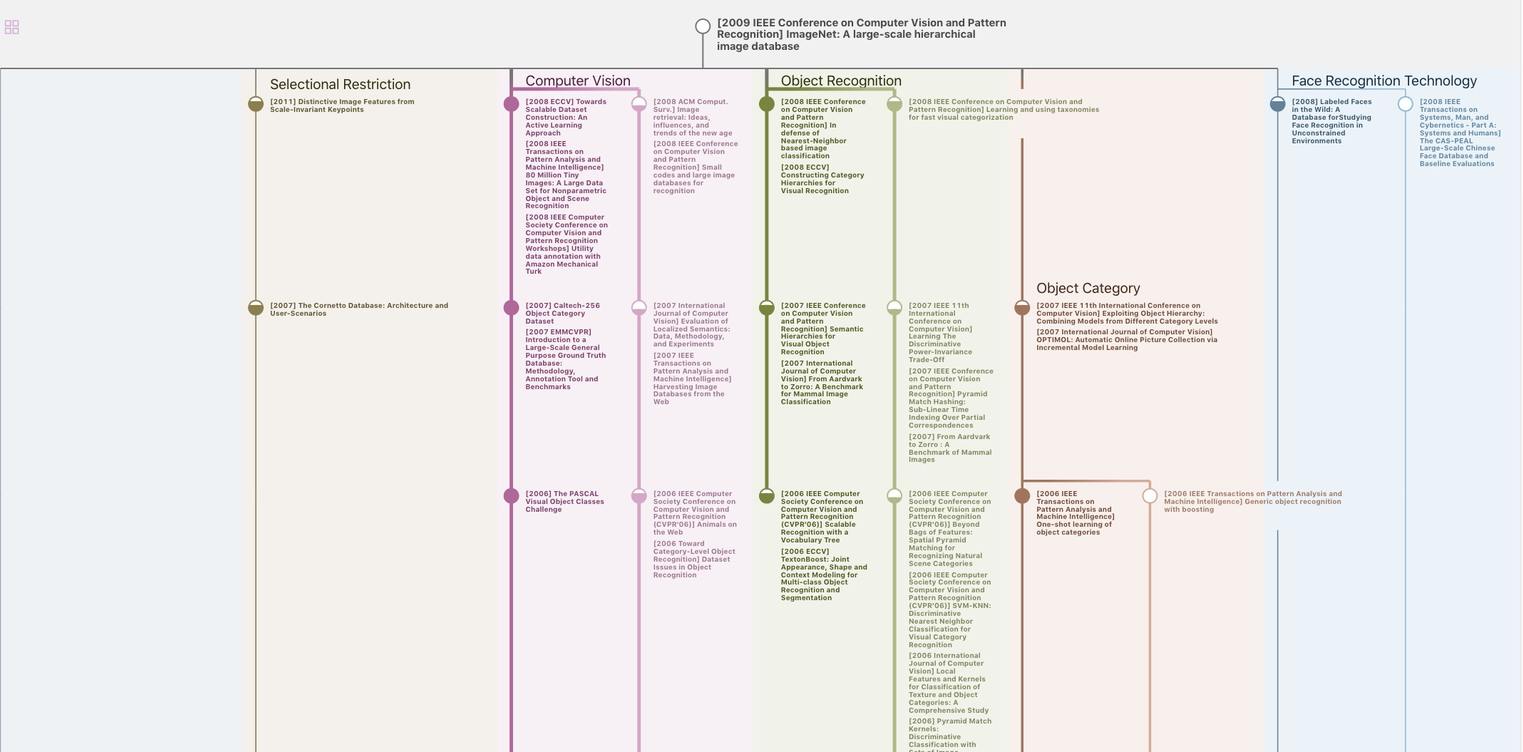
生成溯源树,研究论文发展脉络
Chat Paper
正在生成论文摘要