March on Data Imperfections: Domain Division and Domain Generalization for Semantic Segmentation
MM '20: The 28th ACM International Conference on Multimedia Seattle WA USA October, 2020(2020)
摘要
Significant progress has been made in semantic segmentation by deep neural networks, most of which concentrate on discriminative representation learning. However, model performances suffer from deterioration when the training process is optimized without awareness of data imperfections (e.g., data imbalance and label noise). In contrast to previous works, we present a novel model-agnostic training optimization algorithm which has two prominent components: Domain Division and Domain Generalization. Rather than sampling all pixels uniformly, an uncertainty-based Domain Division method is proposed to deal with data imbalance, which dynamically decomposes the pixels into meta-train and meta-test domains according to whether they lie near the classification boundary. The meta-train domain corresponds to highly-uncertain but more informative pixels and determines the current main update direction. Furthermore, to alleviate the degradation caused by label noise, we propose a Domain Generalization technique with a meta-optimization objective which ensures that update on the meta-train domain should generalize to the meta-test domain. Comprehensive experimental results on three public benchmarks across multi-modalities show that the proposed optimization algorithm is superior to other segmentation optimization methods and significantly outperforms conventional methods without introducing additional model parameters.
更多查看译文
关键词
semantic segmentation, domain division, domain generalization, meta-learning
AI 理解论文
溯源树
样例
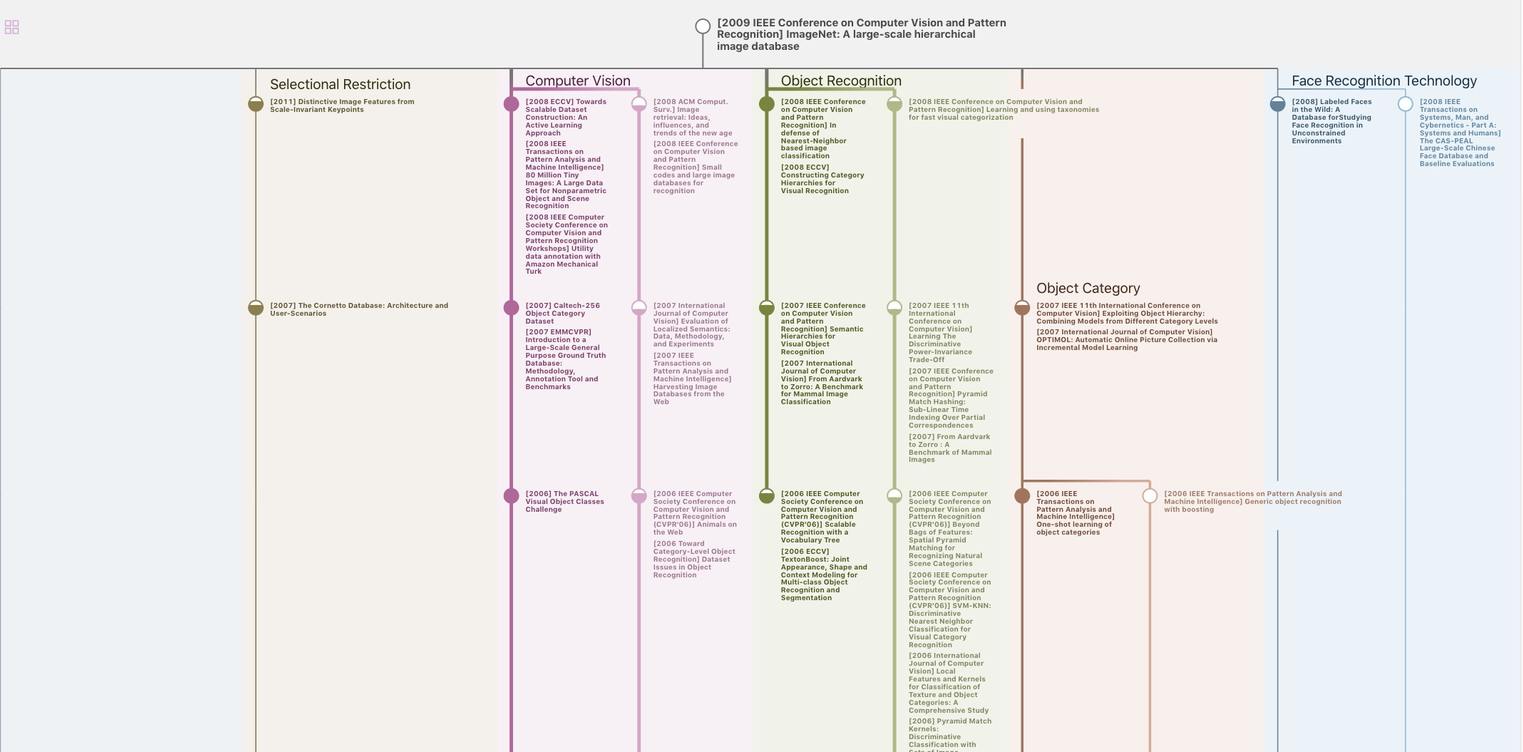
生成溯源树,研究论文发展脉络
Chat Paper
正在生成论文摘要