Self-Mimic Learning for Small-scale Pedestrian Detection
MM '20: The 28th ACM International Conference on Multimedia Seattle WA USA October, 2020(2020)
摘要
Detecting small-scale pedestrians is one of the most challenging problems in pedestrian detection. Due to the lack of visual details, the representations of small-scale pedestrians tend to be weak to be distinguished from background clutters. In this paper, we conduct an in-depth analysis of the small-scale pedestrian detection problem, which reveals that weak representations of small-scale pedestrians are the main cause for a classifier to miss them. To address this issue, we propose a novel Self-Mimic Learning (SML) method to improve the detection performance on small-scale pedestrians. We enhance the representations of small-scale pedestrians by mimicking the rich representations from large-scale pedestrians. Specifically, we design a mimic loss to force the feature representations of small-scale pedestrians to approach those of large-scale pedestrians. The proposed SML is a general component that can be readily incorporated into both one-stage and two-stage detectors, with no additional network layers and incurring no extra computational cost during inference. Extensive experiments on both the CityPersons and Caltech datasets show that the detector trained with the mimic loss is significantly effective for small-scale pedestrian detection and achieves state-of-the-art results on CityPersons and Caltech, respectively.
更多查看译文
关键词
pedestrian detection, small-scale object detection, mimic learning
AI 理解论文
溯源树
样例
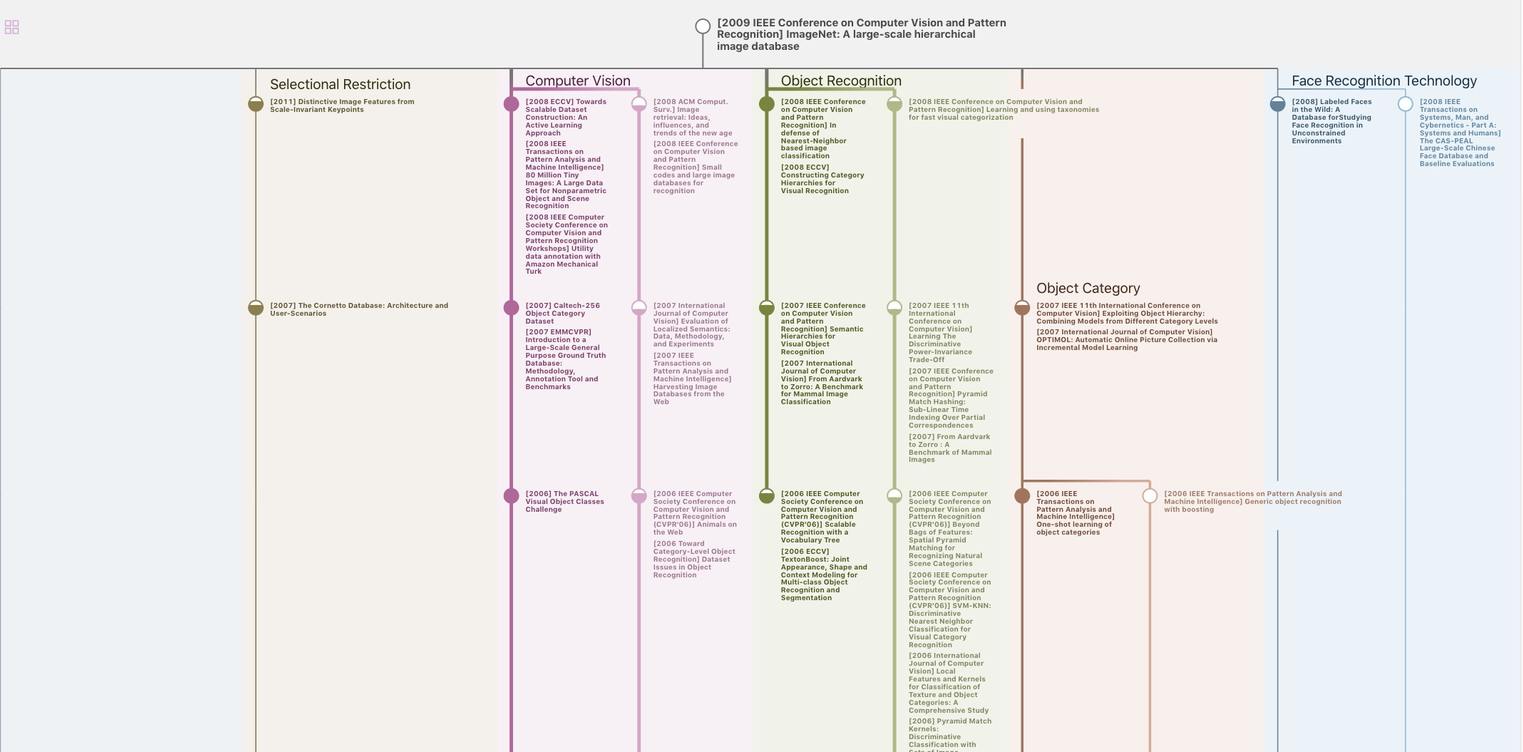
生成溯源树,研究论文发展脉络
Chat Paper
正在生成论文摘要