BlockMix: Meta Regularization and Self-Calibrated Inference for Metric-Based Meta-Learning
MM '20: The 28th ACM International Conference on Multimedia Seattle WA USA October, 2020(2020)
摘要
Most metric-based meta-learning methods learn only the sophisticated similarity metric for few-shot classification, which may lead to the feature deterioration and unreliable prediction. Toward this end, we propose new mechanisms to learn generalized and discriminative feature embeddings as well as improve the robustness of classifiers against prediction corruptions for meta-learning. For this purpose, a new generation operator BlockMix is proposed by integrating interpolation on the images and labels within metric learning. Based on the above BlockMix, we propose a novel regularization method Meta Regularization as an auxiliary task branch with its own classifier to better constraint the feature embedding module and stabilize the meta-learning process. Furthermore, a novel inference scheme Self-Calibrated Inference is proposed to alleviate the unreliable prediction problem by calibrating the prototype of each category with the confidence-weighted average of the support and generated samples. The proposed mechanisms can be used as supplementary techniques alongside standard metric-based meta-learning algorithms without any pre-training. Experimental results demonstrate the insights and the efficiency of the proposed mechanisms respectively, compared with the state-of-the-art methods on the prevalent few-shot benchmarks.
更多查看译文
关键词
meta regularization,self-calibrated,metric-based,meta-learning
AI 理解论文
溯源树
样例
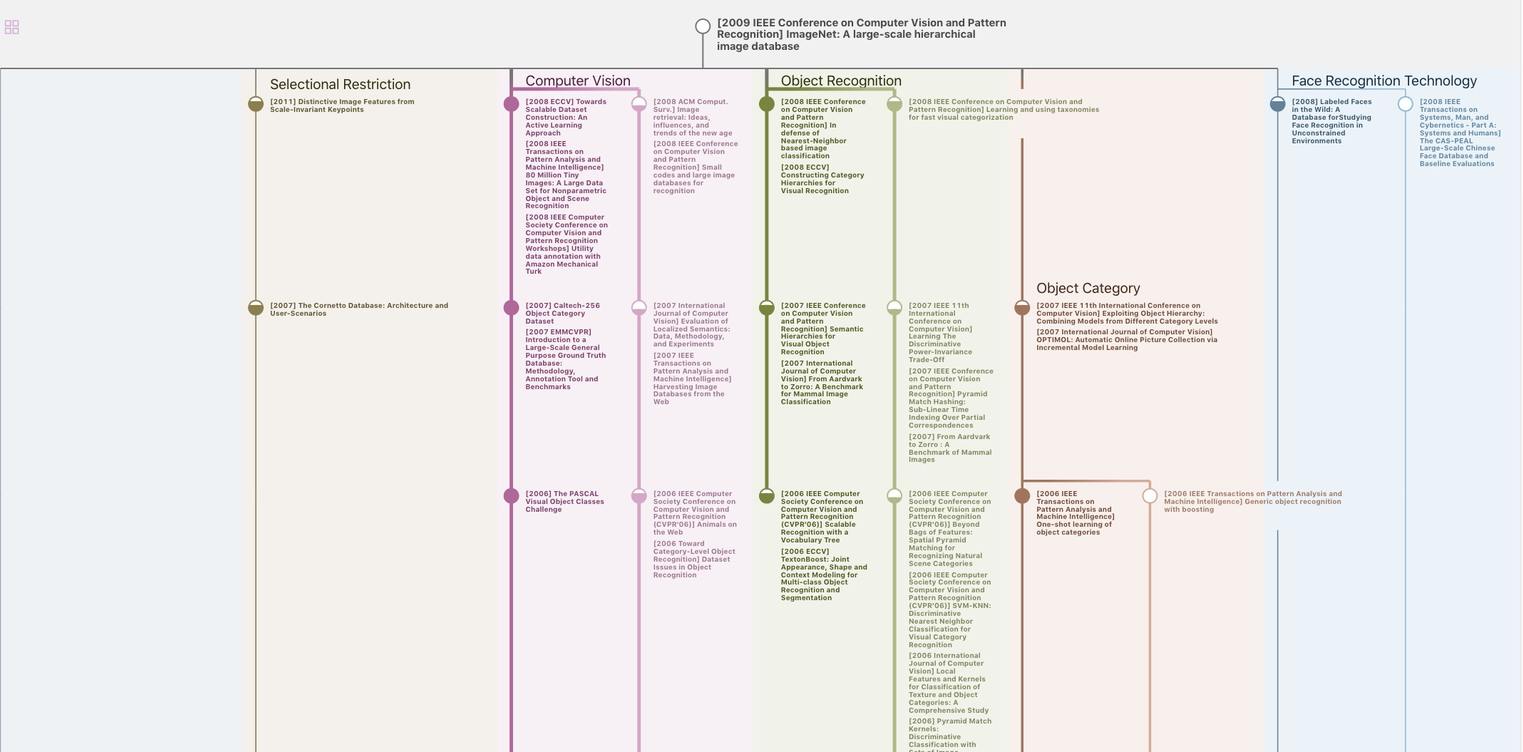
生成溯源树,研究论文发展脉络
Chat Paper
正在生成论文摘要