Utilizing Direct Photocurrent Computation and 2D Kernel Scheduling to Improve In-Sensor-Processing Efficiency
2020 57th ACM/IEEE Design Automation Conference (DAC)(2020)
摘要
Deploying intelligent visual algorithms in terminal devices for always-on sensing is an attractive trend in the IoT era. In-sensor-processing architecture is proposed to reduce power consumption on A/D conversion and data transmission, which performs pre-processing and only converting low-throughput features. However, current designs still require high energy consumption on photoelectric conversion and analog data movement. In this paper, two methods are proposed to improve the energy efficiency of in-sensor-processing architecture, including direct photocurrent computation and 2D kernel scheduling. Photocurrents are directly involved in computation to avoid data conversion; thus the indispensable imaging power is also utilized for computing. Since the location of the pixel data is fixed, data scheduling is conducted on digital weights to eliminate analog data storage and movement. We implement a prototype chip with an array of 32 × 32 units to calculate the first layer of binarized LeNet-5. The post-simulation shows that the proposed architecture reaches the energy efficiency of 11.49TOPs/W, about 14.8x higher than previous works.
更多查看译文
关键词
In-sensor-processing,BNN,photocurrent computation,kernel scheduling
AI 理解论文
溯源树
样例
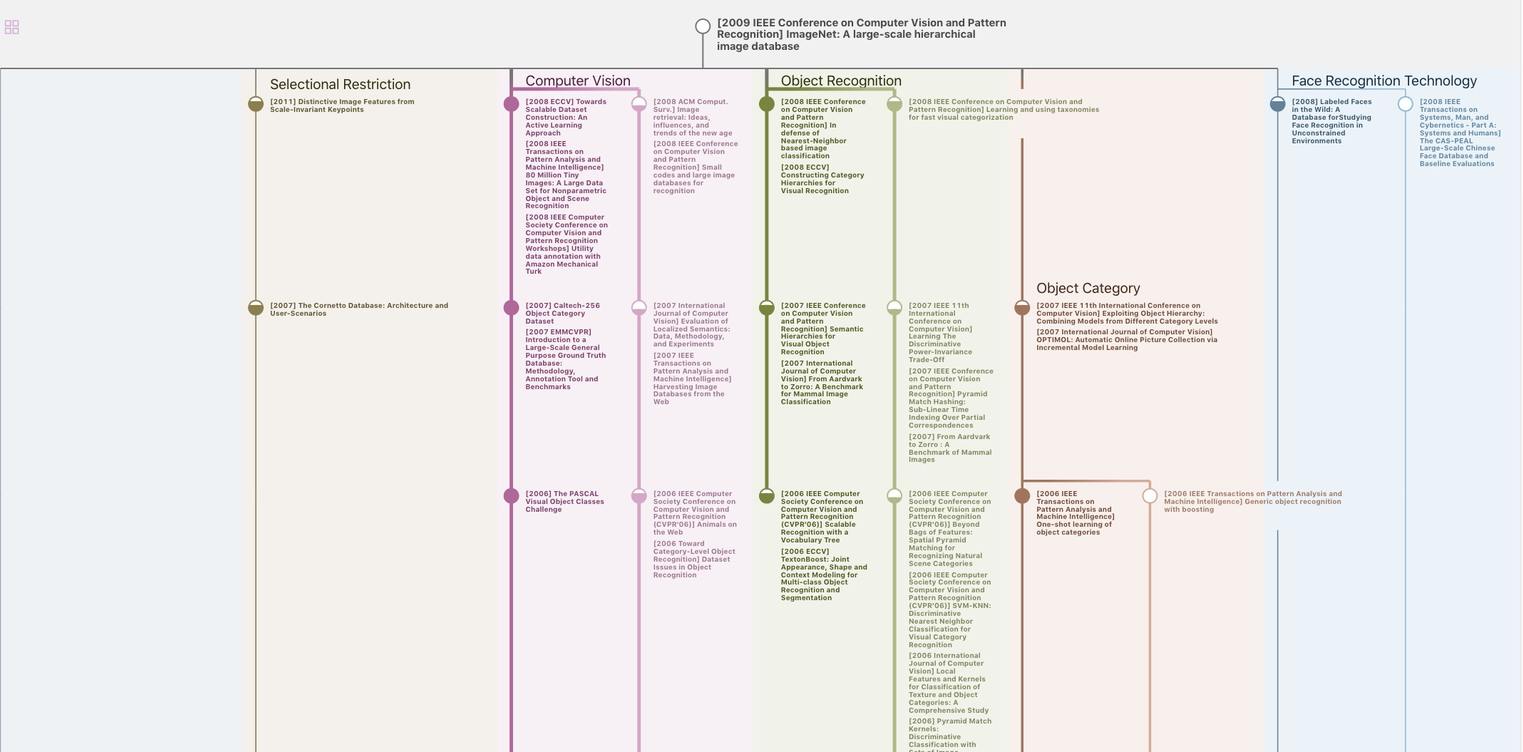
生成溯源树,研究论文发展脉络
Chat Paper
正在生成论文摘要