Deep quantised portrait matting
IET Computer Vision(2020)
摘要
Portrait matting is of vital importance for many applications such as portrait editing, background replacement, ecommerce demonstration, and augmented reality. The portrait matt can be accessed by predicting the α value of the original picture. Previous deep matting methods usually adopt a segmentation network to tackle portrait matting tasks. However, these traditional methods will introduce unpleasant blemishes in the matting results sometimes. The authors find that the key factor behind this phenomenon is how they model the matting problem. On the one hand, α value predicting can be modelled as a regression task. On the other hand, it can be viewed as a classification task of predicting background or foreground. To solve this problem, they explore different methods to model the nature of the α matting problem and propose a novel quantisation-based adaption. Their method comes up with an α quantisation loss to achieve multi-threshold filtering. Furthermore, they apply an α merging block to improve conventional regression methods. With their method, the gradient loss is reduced by 7.53% relatively, with mean square error and sum of absolute difference decreased by 14.7% relatively, leading to a more visually pleasant α matt in several segmentation backbones.
更多查看译文
关键词
augmented reality,image colour analysis,regression analysis,image filtering,mean square error methods,image classification
AI 理解论文
溯源树
样例
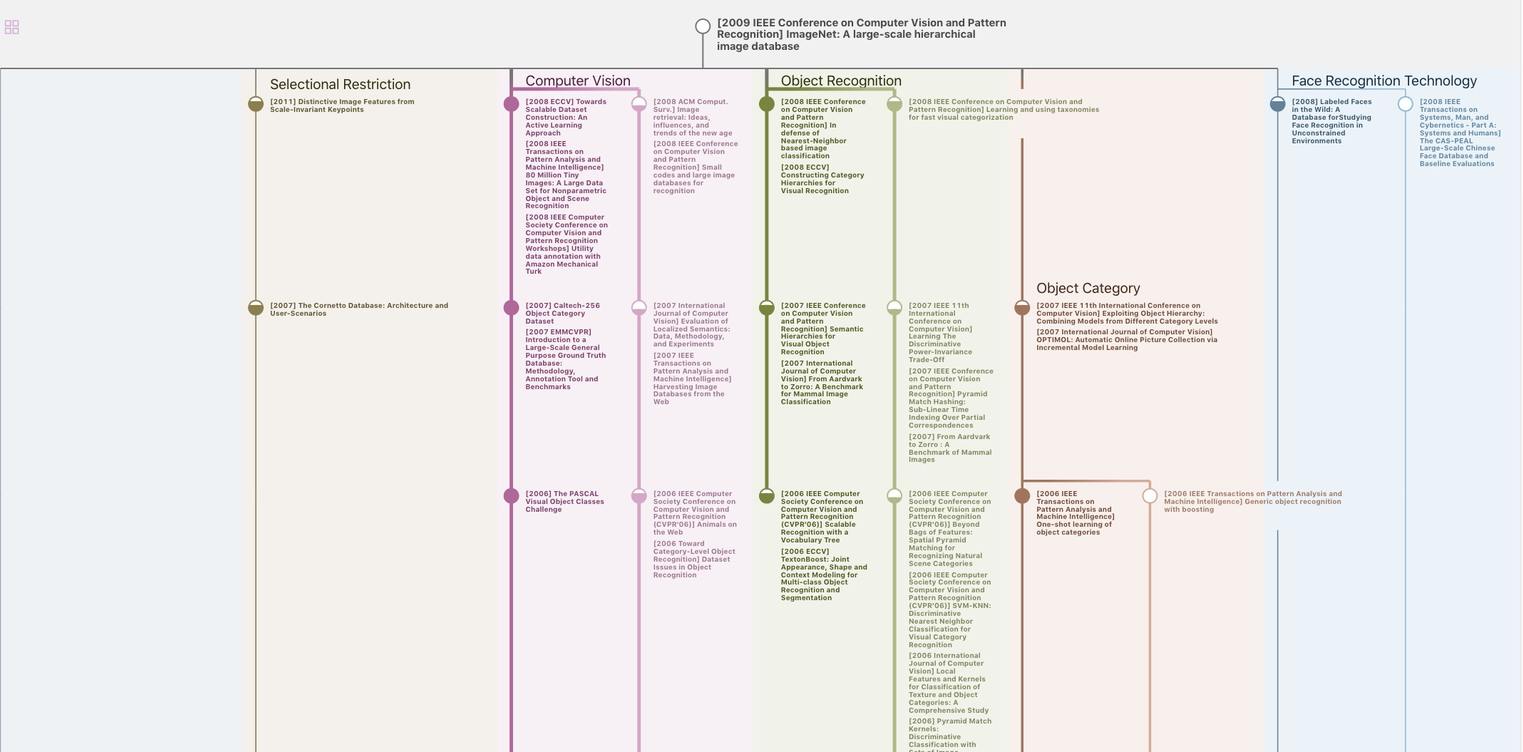
生成溯源树,研究论文发展脉络
Chat Paper
正在生成论文摘要