Integrated Double Estimator Architecture for Reinforcement Learning
IEEE Transactions on Cybernetics(2022)
摘要
Estimation bias is an important index for evaluating the performance of reinforcement learning (RL) algorithms. The popular RL algorithms, such as
$Q$
-learning and deep
$Q$
-network (DQN), often suffer overestimation due to the maximum operation in estimating the maximum expected action values of the next states, while double
$Q$
-learning (DQ) and double DQN may fall into underestimation by using a double estimator (DE) to avoid overestimation. To keep the balance between overestimation and underestimation, we propose a novel integrated DE (IDE) architecture by combining the maximum operation and DE operation to estimate the maximum expected action value. Based on IDE, two RL algorithms: 1) integrated DQ (IDQ) and 2) its deep network version, that is, integrated double DQN (IDDQN), are proposed. The main idea of the proposed RL algorithms is that the maximum and DE operations are integrated to eliminate the estimation bias, where one estimator is stochastically used to perform action selection based on the maximum operation, and the convex combination of two estimators is used to carry out action evaluation. We theoretically analyze the reason of estimation bias caused by using nonmaximum operation to estimate the maximum expected value and investigate the possible reasons of underestimation existence in DQ. We also prove the unbiasedness of IDE and convergence of IDQ. Experiments on the grid world and Atari 2600 games indicate that IDQ and IDDQN can reduce or even eliminate estimation bias effectively, enable the learning to be more stable and balanced, and improve the performance effectively.
更多查看译文
关键词
Algorithms,Learning,Reinforcement, Psychology
AI 理解论文
溯源树
样例
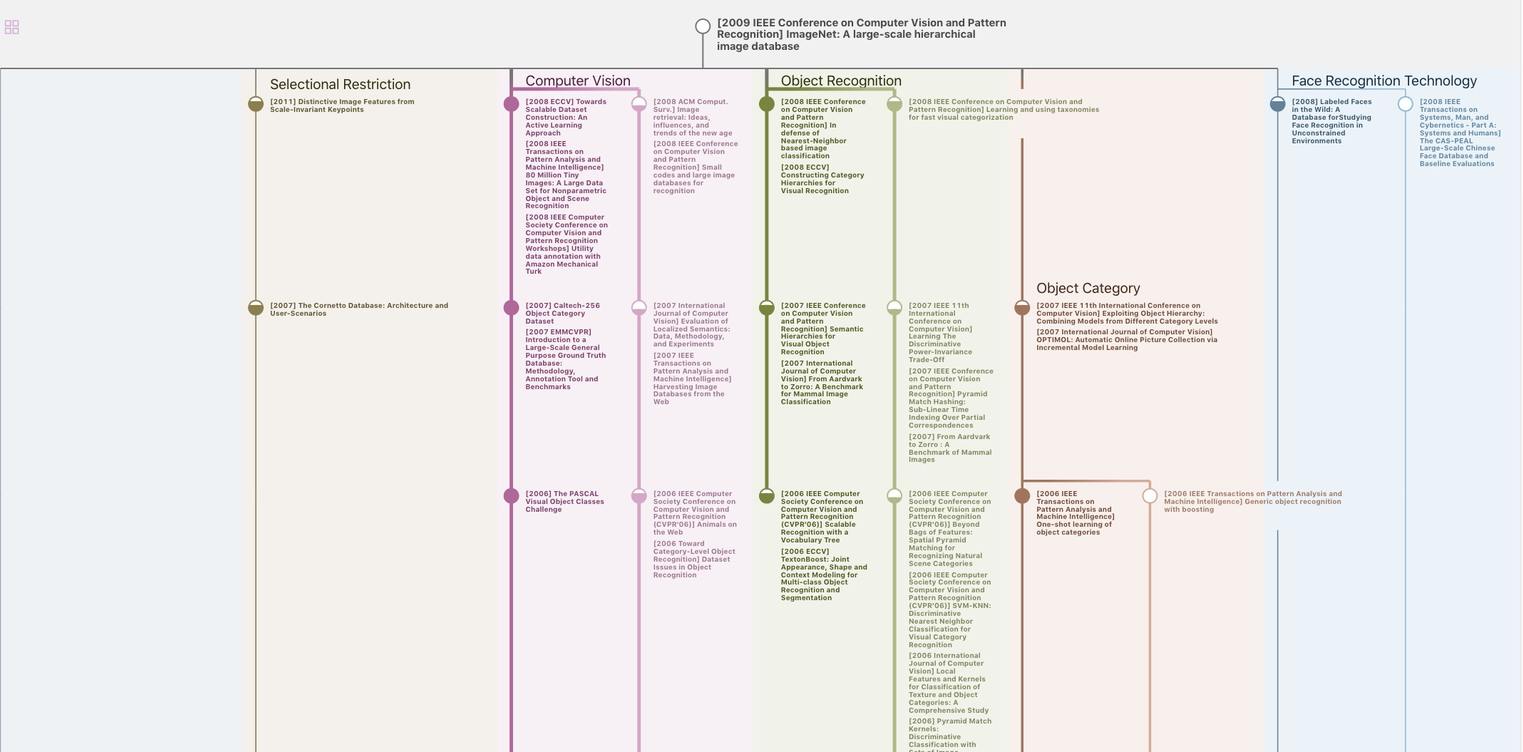
生成溯源树,研究论文发展脉络
Chat Paper
正在生成论文摘要