Machine Vision based Automation to Improve Process and Quality Control in Rolling Mills §
2020 25th IEEE International Conference on Emerging Technologies and Factory Automation (ETFA)(2020)
摘要
Steel manufacturing industries take advantage of growing infrastructure market by producing high volumes of rolling products like rebars. However, in the face of competition, better cost effective automation solutions are needed to run rolling mills at a high speed which would guarantee a high return of investment. In this paper, automatic, visual inspection methods are proposed to investigate rebar falling patterns and rebar surface properties at very early stage during the production of rolling stock. We propose a multi-modal adaptive control approach for rolling processes with camera, process, and material data to improve process and quality control in rolling mills.Image processing methods are proposed to detect and identify abnormalities in rolling stock and communicate visual information with a feedback mechanism to a control system and/or a manual operator. The visual information is transformed into sequential data with exponential weighted mean statistics and symbolic aggregate approximation techniques. We demonstrate, on real video streams, deviation statistics and identify set point corrections to improve time to deliver the stock; generate interpretable labels to quantify material loss and identify extent of deficiencies in rolling stock for assistance to manual operators.
更多查看译文
关键词
Quality Inspection,Process Control,Machine Vision,Image Processing,Industrial Automation,Rolling Mills
AI 理解论文
溯源树
样例
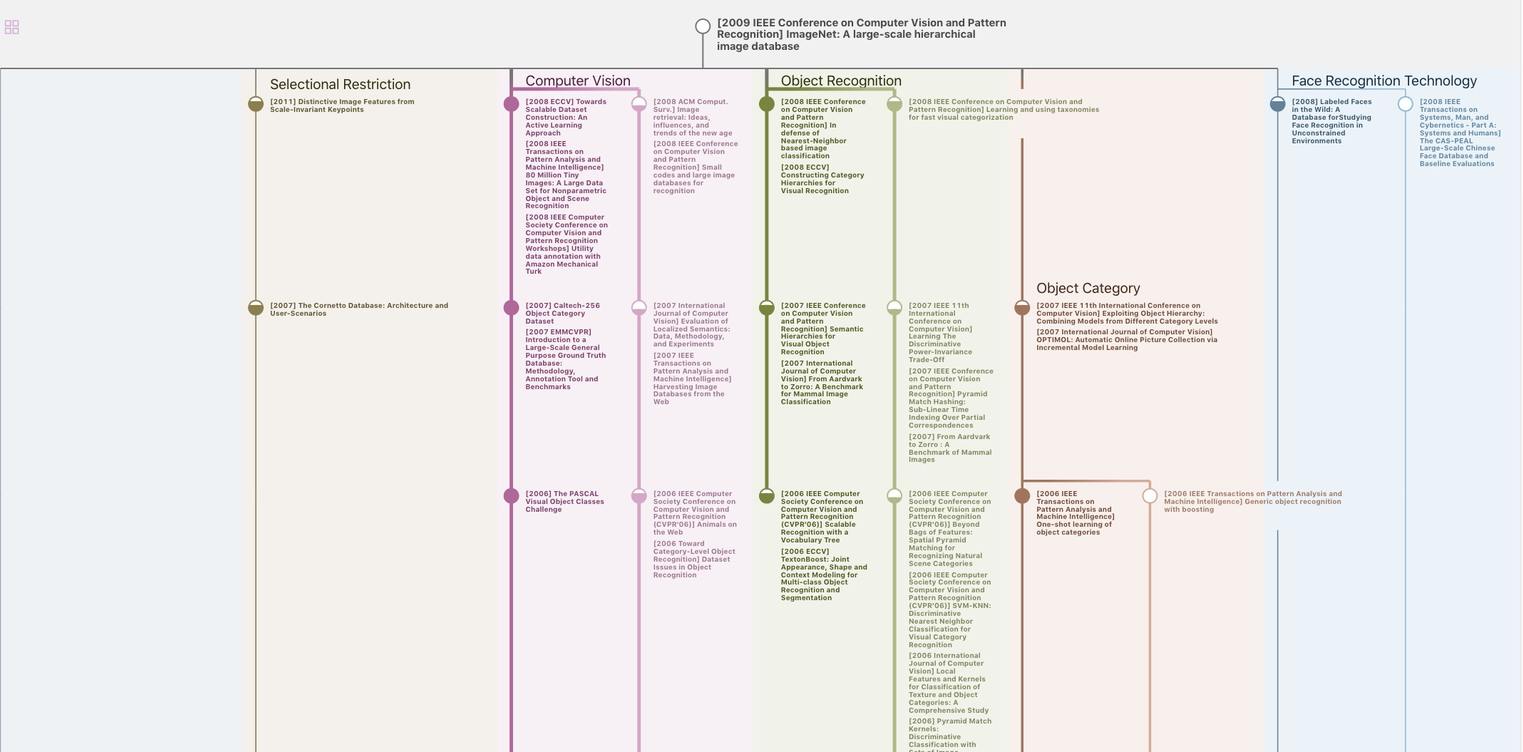
生成溯源树,研究论文发展脉络
Chat Paper
正在生成论文摘要