Two-Dimensional Semi-Supervised Feature Selection
2020 10th International Conference on Information Science and Technology (ICIST)(2020)
摘要
Data with a few labels are common in reality and corresponding semi-supervised learning methods have good application percepts such as image process and computer vision. However, most of conventional semi-supervised feature selection methods, they first convert the image data into corresponding vectors and then utilize the vectors for feature selection. However, these vector-based methods fail to consider the intrinsic location information of the matrix data resulting in degrading performance. To overcome these problems, in this paper, we propose a two-dimensional semi-supervised feature selection model, which directly selects the features from the matrix data by using both labeled and unlabeled data. Specifically, our proposed method unifies the sparse matrix regression and label prediction procedures to conduct feature selection. To solve our model, an alternating optimization algorithm is also proposed. Furthermore, we provide analysis about our proposed model including convergence analysis and computational complexity. Extensive experimental results confirm the effectiveness of the proposed model.
更多查看译文
关键词
two-dimensional data,sparse matrix regression,feature selection,semi-supervised learning
AI 理解论文
溯源树
样例
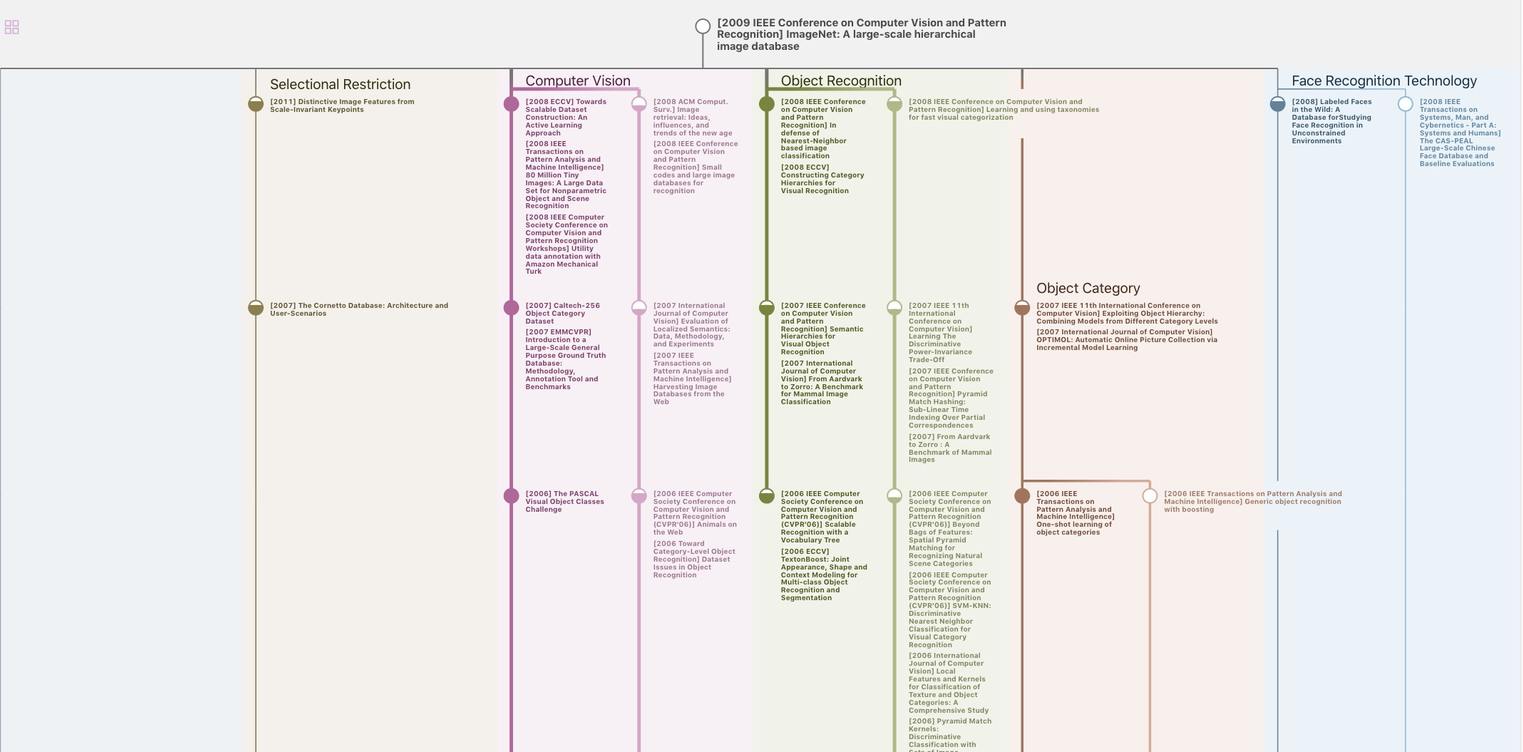
生成溯源树,研究论文发展脉络
Chat Paper
正在生成论文摘要