Solving the where problem in neuroanatomy: a generative framework with learned mappings to register multimodal, incomplete data into a reference brain
biorxiv(2024)
摘要
A current focus of research in neuroscience is to enumerate, map and annotate neuronal cell types in whole vertebrate brains using different modalities of data acquisition. Mapping these molecular and anatomical datasets into a common reference space remains a key challenge. While several brain-to-atlas mapping workflows exist, they do not adequately address challenges of modern high throughput neuroimaging, including multimodal and multiscale signals, missing data or non reference signals, and geometric quantification of individual variation. Our solution is to implement a generative statistical model that describes the likelihood of imaging data given a sequence of transforms of an atlas image, and a framework for maximum a posteriori estimation of unknown parameters capturing the issues listed above. The key idea in our approach is to minimize the difference between synthetic image volumes and real data over these parameter. Rather than merely using mappings as a "normalization" step, we implement tools for using their local metric changes as an opportunity for geometric quantification of technical and biological sources of variation in an unprecedented manner. While the framework is used to compute pairwise mappings, our approach particularly allows for easy compositions across chains of multimodality datasets. We apply these methods across a broad range of datasets including various combinations of in-vivo and ex-vivo MRI, 3D STP and fMOST data sets, 2D serial histology sections, and brains processed for snRNAseq with tissue partially removed. We show biological utility by quantifying cell density and diffeomorphic characterization of brain shape fluctuations across biological covariates. We note that the magnitude of individual variation is often greater than differences between different sample preparation techniques. To facilitate community accessibility, we implement our algorithm as open source, include a web based framework, and implement input and output dataset standards. Our work establishes a quantitative, scalable and streamlined workflow for unifying a broad spectrum of multi-modal whole-brain light microscopic data volumes into a coordinate-based atlas framework. This work enables large scale integration of whole brain data sets that are essential in modern neuroscience.
### Competing Interest Statement
The authors have declared no competing interest.
更多查看译文
关键词
generative framework,brain,multimodal,mappings,neuroanatomy
AI 理解论文
溯源树
样例
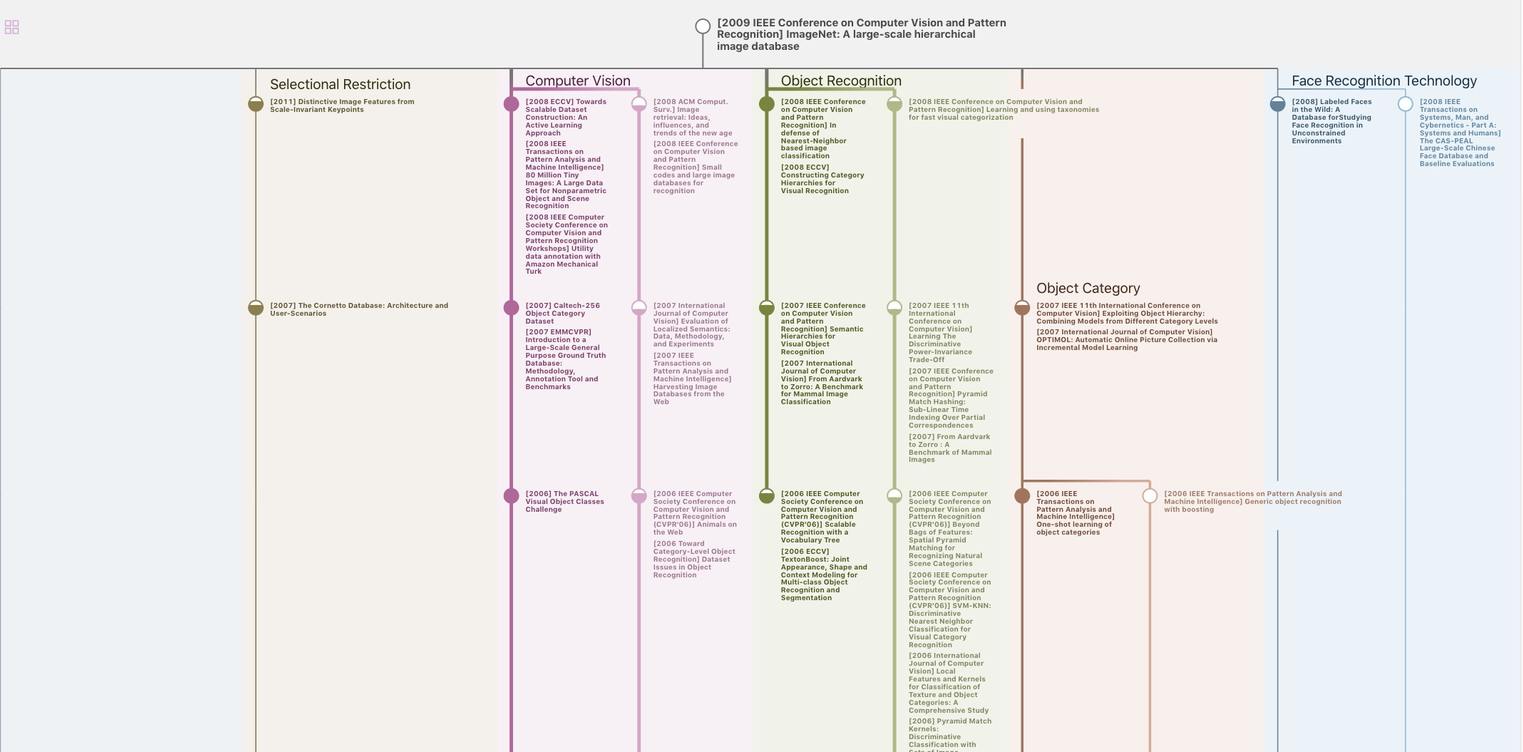
生成溯源树,研究论文发展脉络
Chat Paper
正在生成论文摘要