Autoencoder Based Local T Cell Repertoire Density Can Be Used To Classify Samples And T Cell Receptors
PLOS COMPUTATIONAL BIOLOGY(2021)
摘要
Author summary T cell repertoires contain a vast amount of information on the donors, and can be used to characterize the donor, and apply machine learning algorithms on such repertoires. A limiting factor in the analysis of such repertoire is the lack of a good representation of the T cell receptors. We here propose an autoencoder, named ELATE to present receptors as real vectors. We show that this encoder can be used to characterize both full donors and specific receptors using either supervised or unsupervised methods.Recent advances in T cell repertoire (TCR) sequencing allow for the characterization of repertoire properties, as well as the frequency and sharing of specific TCR. However, there is no efficient measure for the local density of a given TCR. TCRs are often described either through their Complementary Determining region 3 (CDR3) sequences, or theirV/J usage, or their clone size. We here show that the local repertoire density can be estimated using a combined representation of these components through distance conserving autoencoders and Kernel Density Estimates (KDE). We present ELATE-an Encoder-based LocAl Tcr dEnsity and show that the resulting density of a sample can be used as a novel measure to study repertoire properties. The cross-density between two samples can be used as a similarity matrix to fully characterize samples from the same host. Finally, the same projection in combination with machine learning algorithms can be used to predict TCR-peptide binding through the local density of known TCRs binding a specific target.
更多查看译文
关键词
T cell receptor,Local density,Autoencoder,Distance conserving,Machine learning
AI 理解论文
溯源树
样例
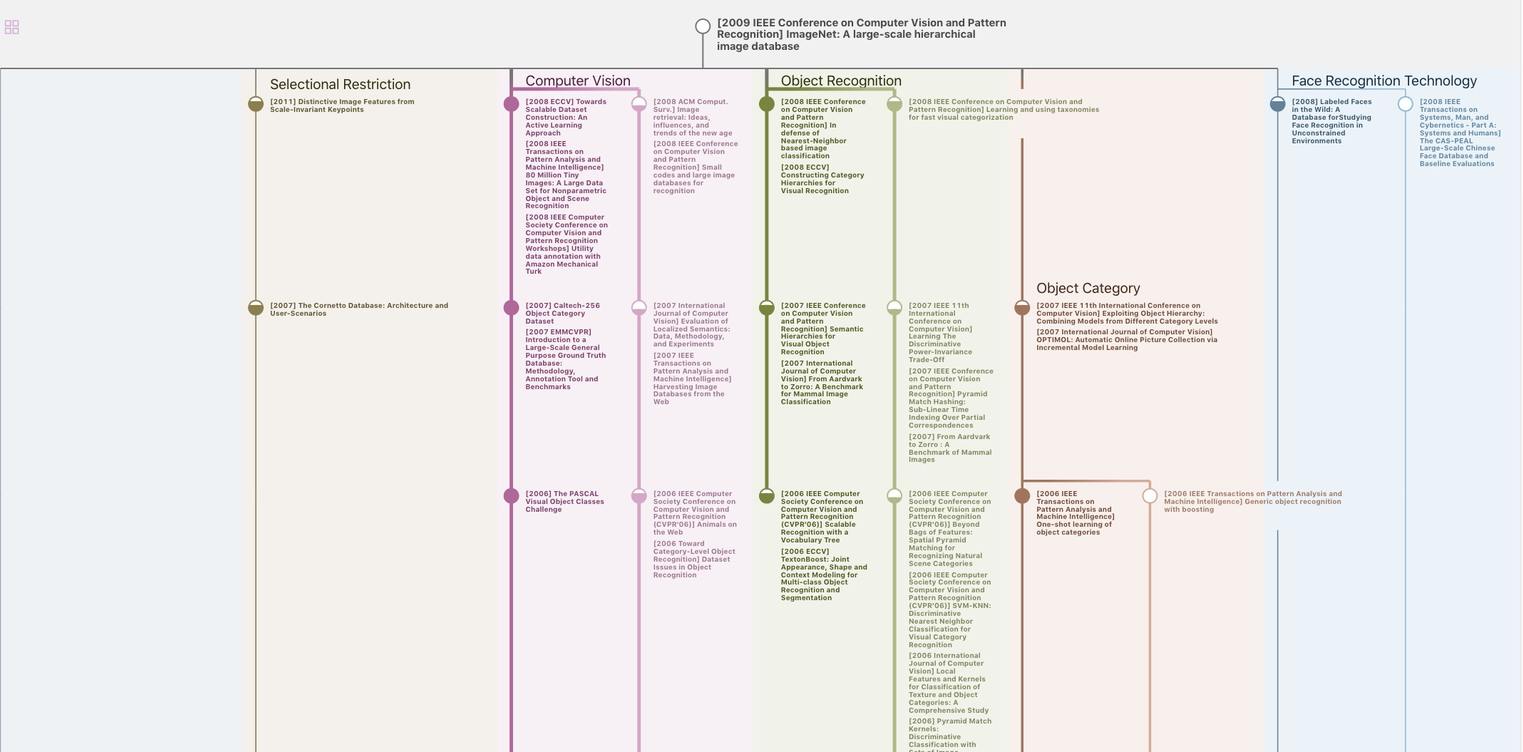
生成溯源树,研究论文发展脉络
Chat Paper
正在生成论文摘要