Spatiotemporal Feature Selection Improves Prediction Accuracy of Multi-Voxel Pattern Classification
biorxiv(2019)
摘要
The importance of spatiotemporal feature selection in fMRI decoding studies has not been studied exhaustively. Temporal embedding of features allows the incorporation of brain activity dynamics into multivariate pattern classification, and may provide enriched information about stimulus-specific response patterns and potentially improve prediction accuracy. This study investigates the possibility of enhancing the classification performance by exploring spatial and temporal (spatiotemporal) domain, to identify the optimum combination of the spatiotemporal features based on the classification performance. We investigated the importance of spatiotemporal feature selection using a slow event-related design adapted from the classic study. Data were collected using a multiband fMRI sequence with temporal resolution of 0.568 seconds. A wide range of spatiotemporal observations was created as various combinations of spatiotemporal features. Using both random forest, and support vector machine, classifiers, prediction accuracies for these combinations were then compared with the single time-point spatial multivariate pattern approach that uses only a single temporal observation. The results showed that on average spatiotemporal feature selection improved prediction accuracy. Moreover, the random forest algorithm outperformed the support vector machine and benefitted from temporal information to a greater extent. As expected, the most influential temporal durations were found to be around the peak of the hemodynamic response function, a few seconds after the stimuli onset until ∼4 seconds after the peak of the hemodynamic response function. The superiority of spatiotemporal feature selection over single time-point spatial approaches invites future work to design systematic and optimal approaches to the incorporation of spatiotemporal dependencies into feature selection for decoding.
更多查看译文
关键词
fMRI,Multi-Variate Pattern Classification,Spatiotemporal Feature Selection,Multi-band EPI,Random Forest,Support Vector Machine
AI 理解论文
溯源树
样例
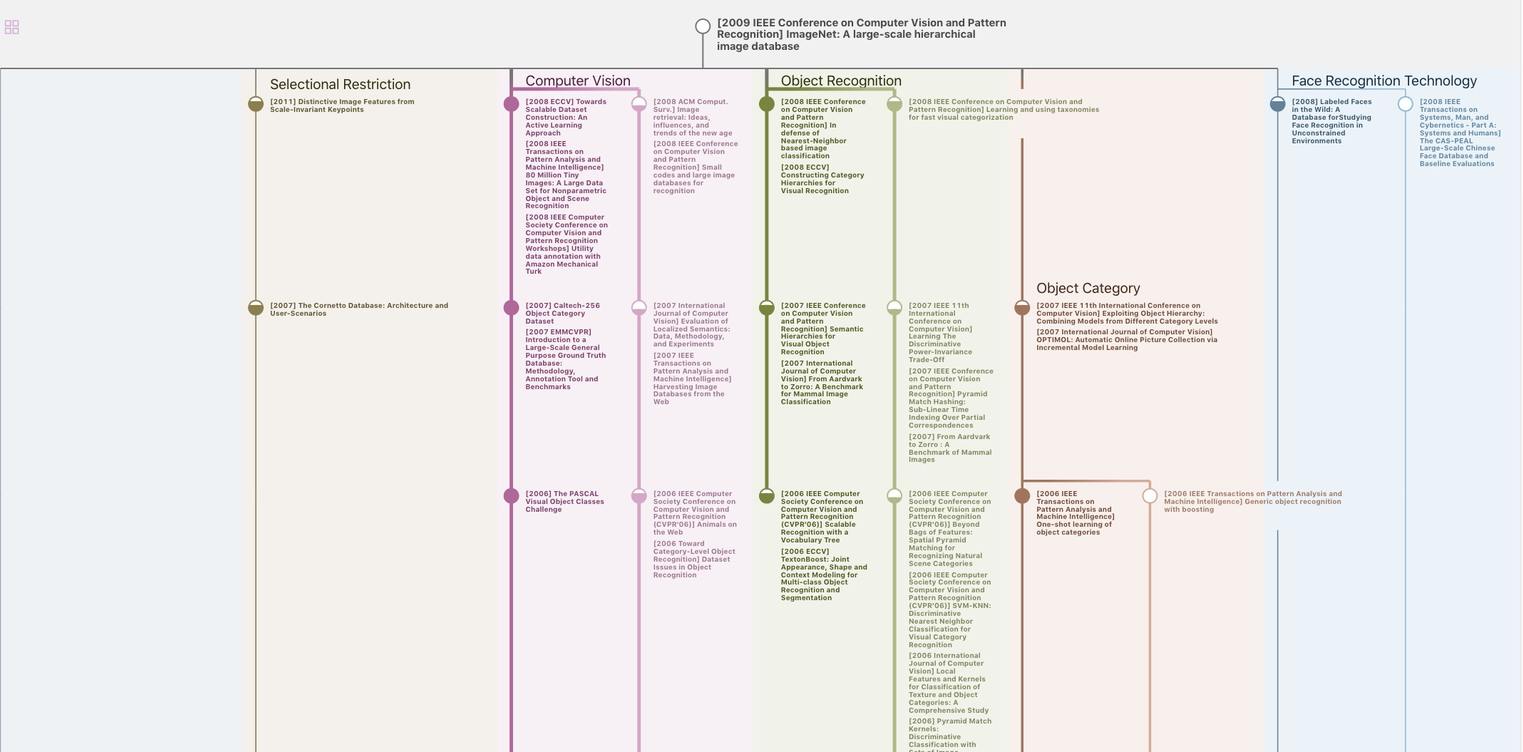
生成溯源树,研究论文发展脉络
Chat Paper
正在生成论文摘要