State-Machine-Based Dialogue Agents with Few-Shot Contextual Semantic Parsers
arxiv(2020)
摘要
This paper presents a methodology and toolkit for creating a rule-based multi-domain conversational agent for transactions from (1) language annotations of the domains' database schemas and APIs and (2) a couple of hundreds of annotated human dialogues. There is no need for a large annotated training set, which is expensive to acquire. The toolkit uses a pre-defined abstract dialogue state machine to synthesize millions of dialogues based on the domains' information. The annotated and synthesized data are used to train a contextual semantic parser that interprets the user's latest utterance in the context of a formal representation of the conversation up to that point. Developers can refine the state machine to achieve higher accuracy. On the MultiWOZ benchmark, we achieve over 71% turn-by-turn slot accuracy on a cleaned, reannotated test set, without using any of the original training data. Our state machine can model 96% of the human agent turns. Our training strategy improves by 9% over a baseline that uses the same amount of hand-labeled data, showing the benefit of synthesizing data using the state machine.
更多查看译文
关键词
dialogue agents,state-machine-based,few-shot
AI 理解论文
溯源树
样例
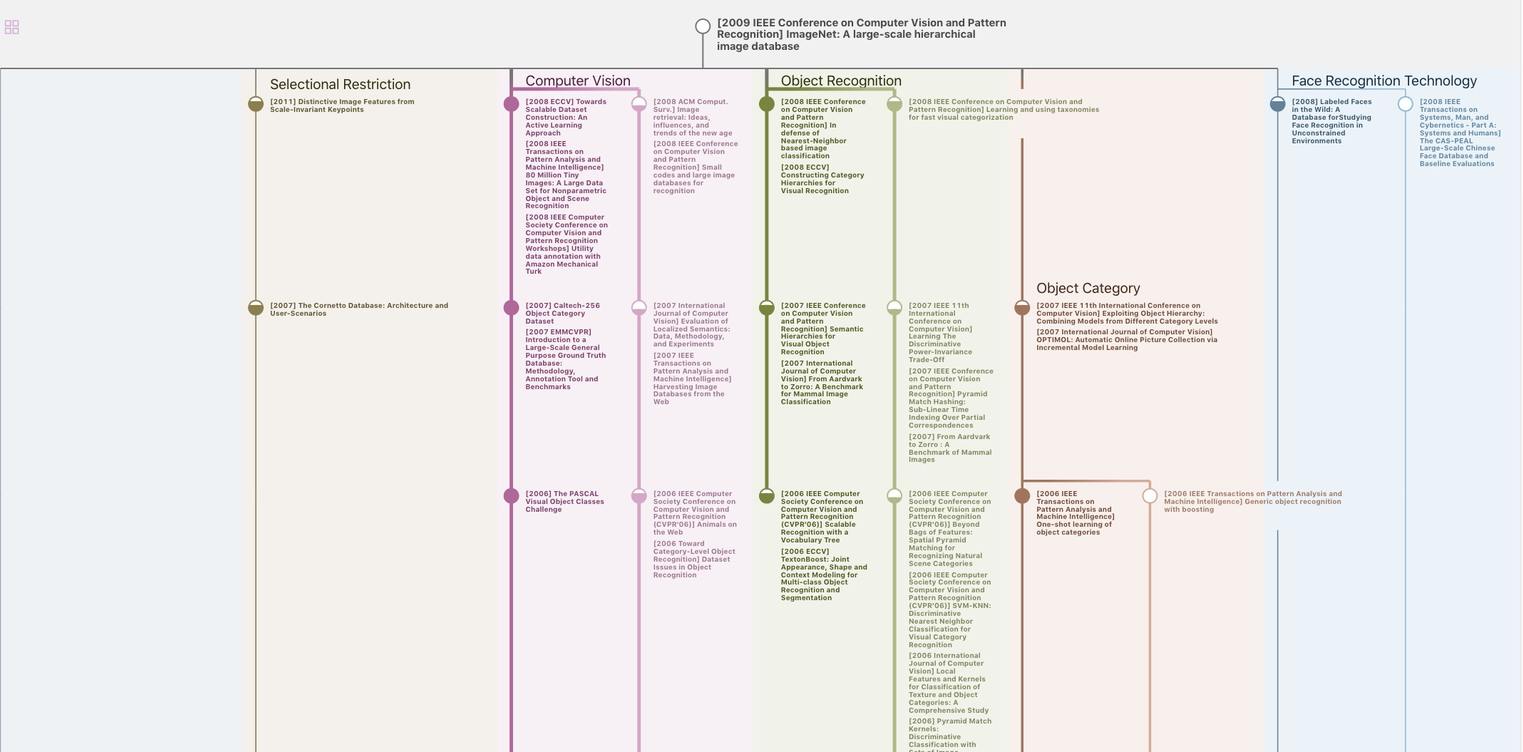
生成溯源树,研究论文发展脉络
Chat Paper
正在生成论文摘要