Lower Bounds for Policy Iteration on Multi-action MDPs
CDC(2020)
摘要
Policy Iteration (PI) is a classical family of algorithms to compute an optimal policy for any given Markov Decision Problem (MDP). The basic idea in PI is to begin with some initial policy and to repeatedly update the policy to one from an improving set, until an optimal policy is reached. Different variants of PI result from the (switching) rule used for improvement. An important theoretical question is how many iterations a specified PI variant will take to terminate as a function of the number of states n and the number of actions k in the input MDP. While there has been considerable progress towards upper-bounding this number, there are fewer results on lower bounds. In particular, existing lower bounds primarily focus on the special case of k = 2 actions. We devise lower bounds for k ≥ 3. Our main result is that a particular variant of PI can take Ω(k
n
/2) iterations to terminate. We also generalise existing constructions on 2-action MDPs to scale lower bounds by a factor of k for some common deterministic variants of PI, and by log(k) for corresponding randomised variants.
更多查看译文
关键词
policy iteration,multi-action
AI 理解论文
溯源树
样例
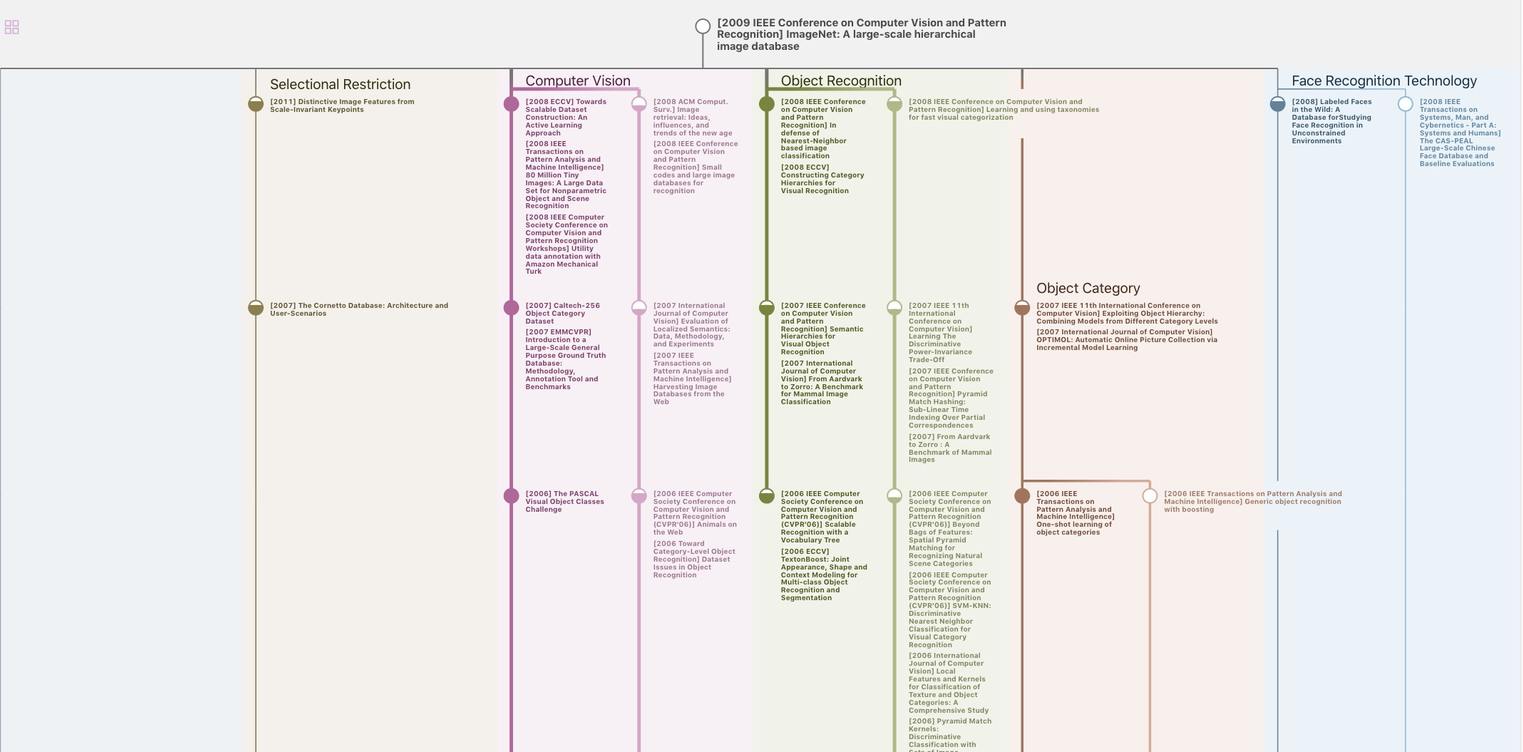
生成溯源树,研究论文发展脉络
Chat Paper
正在生成论文摘要