Human activity recognition using multi-input CNN model with FFT spectrograms
UbiComp/ISWC '20: 2020 ACM International Joint Conference on Pervasive and Ubiquitous Computing and 2020 ACM International Symposium on Wearable Computers Virtual Event Mexico September, 2020(2020)
摘要
An activity recognition method developed by Team DSML-TDU for the Sussex-Huawei Locomotion-Transportation (SHL) recognition challenge was descrived. Since the 2018 challenge, our team has been developing human activity recognition models based on a convolutional neural network (CNN) using Fast Fourier Transform (FFT) spectrograms from mobile sensors. In the 2020 challenge, we developed our model to fit various users equipped with sensors in specific positions. Nine modalities of FFT spectrograms generated from the three axes of the linear accelerometer, gyroscope, and magnetic sensor data were used as input data for our model. First, we created a CNN model to estimate four retention positions (Bag, Hand, Hips, and Torso) from the training data and validation data. The provided test data was expected to from Hips. Next, we created another (pre-trained) CNN model to estimate eight activities from a large amount of user 1 training data (Hips). Then, this model was fine-tuned for different users by using the small amount of validation data for users 2 and 3 (Hips). Finally, an F-measure of 96.7% was obtained as a result of 5-fold-cross validation.
更多查看译文
关键词
Human activity recognition, SHL dataset, CNN, FFT spectrogram
AI 理解论文
溯源树
样例
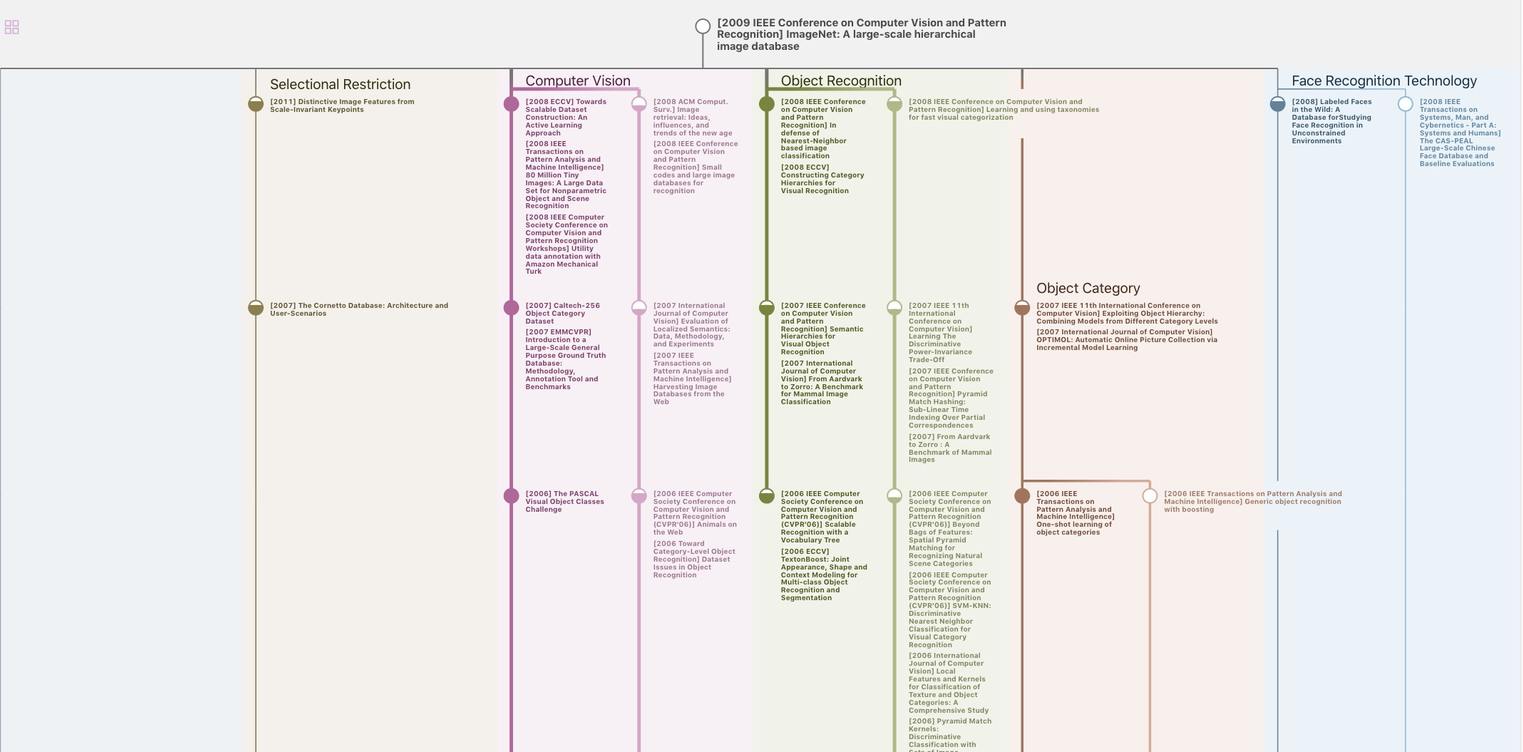
生成溯源树,研究论文发展脉络
Chat Paper
正在生成论文摘要