SIP: Boosting Up Graph Computing by Separating the Irregular Property Data
GLSVLSI '20: Great Lakes Symposium on VLSI 2020 Virtual Event China September, 2020(2020)
摘要
Graph analytics is an important class of applications and is one of the cornerstone of big-data workloads. Unfortunately, due to poor data locality in most graph applications, conventional general-purpose computer architectures are unable to perform the best of their processing abilities. The main source of poor locality comes from accessing vertex properties. Upper-level caches cannot hold data blocks long enough due to their limited capacity and the long reuse distance of vertex properties. Moreover, accesses to properties can evict other useful data with good locality, which causes more conflicting misses. In this work, a small cache is added exclusively for the properties to solve this problem. We further enhance this structure with prefetchers to increase the hit rate of properties and improve performance of system. Experimental results show that compared to two state-of-the-art prefetcher and accelerator for graph computing, our proposed architecture achieves 1.13x-2.54x and 1.04x-1.27x performance improvements. In the meanwhile, the energy consumptions can be saved by 6.41%-13.43% and 34.67%-43.92% respectively.
更多查看译文
AI 理解论文
溯源树
样例
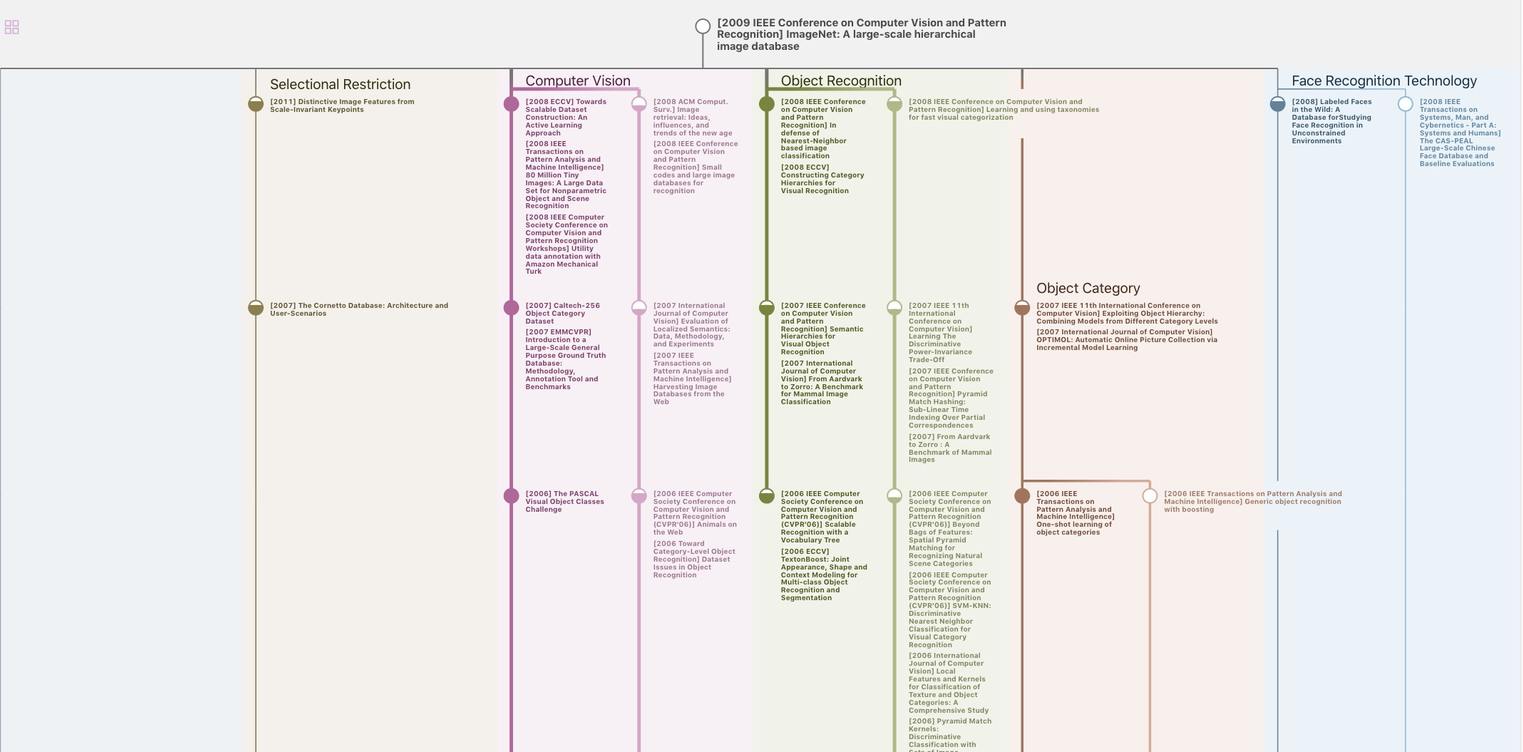
生成溯源树,研究论文发展脉络
Chat Paper
正在生成论文摘要