Interactive and Explainable Point-of-Interest Recommendation using Look-alike Groups
SIGSPATIAL '20: 28th International Conference on Advances in Geographic Information Systems Seattle WA USA November, 2020(2020)
摘要
Recommending Points-of-Interest (POIs) is surfacing in many location-based applications. The literature contains personalized and socialized POI recommendation approaches which employ historical check-ins and social links to make recommendations. However these systems still lack customizability and contextuality particularly in cold start situations. In this paper, we propose LikeMind, a POI recommendation system which tackles the challenges of cold start, customizability, contextuality, and explainability by exploiting look-alike groups mined in public POI datasets. LikeMind reformulates the problem of POI recommendation, as recommending explainable look-alike groups (and their POIs) which are in line with user's interests. LikeMind frames the task of POI recommendation as an exploratory process where users interact with the system by expressing their favorite POIs, and their interactions impact the way look-alike groups are selected out. Moreover, LikeMind employs "mindsets", which capture actual situation and intent of the user, and enforce the semantics of POI interestingness. In an extensive set of experiments, we show the quality of our approach in recommending relevant look-alike groups and their POIs, in terms of efficiency and effectiveness.
更多查看译文
关键词
point-of-interest,look-alike
AI 理解论文
溯源树
样例
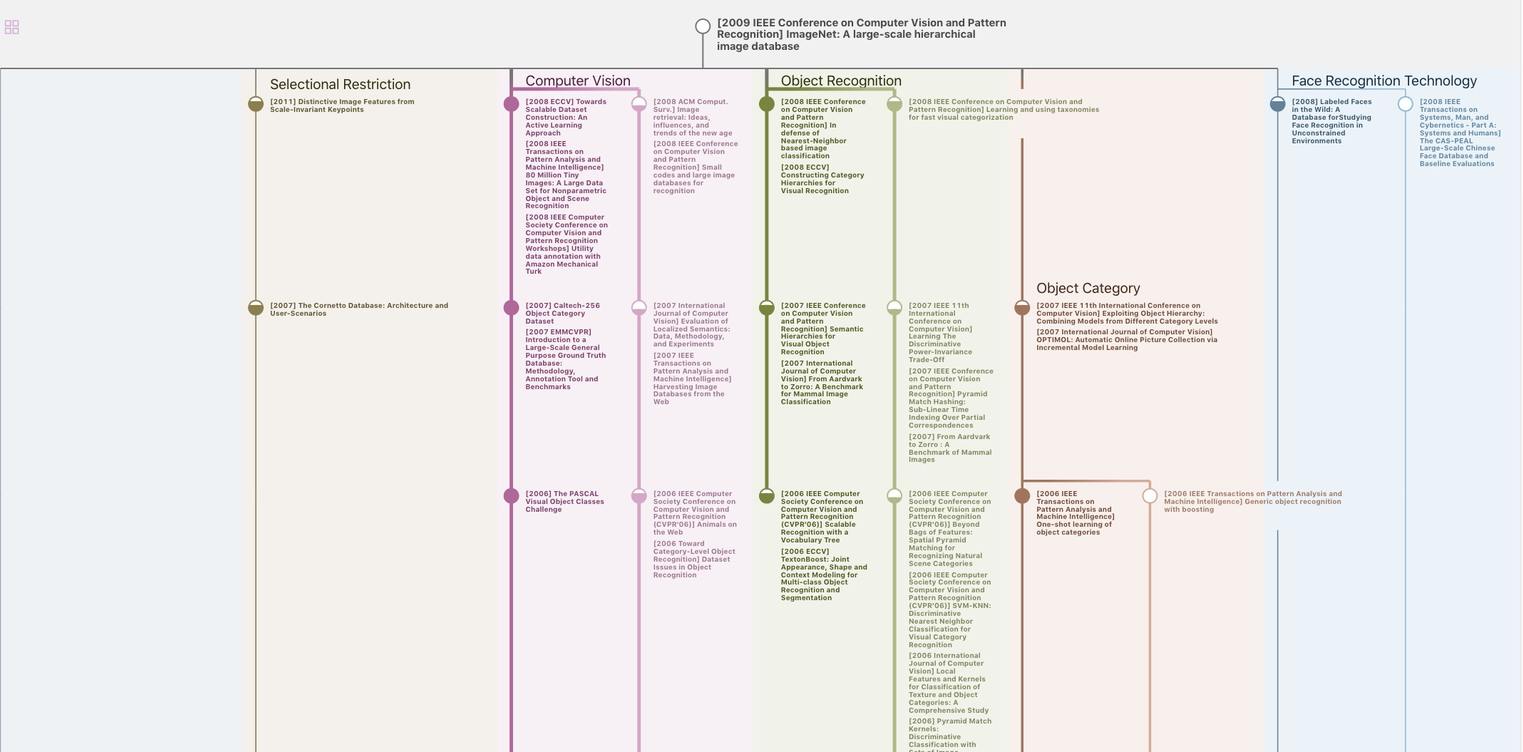
生成溯源树,研究论文发展脉络
Chat Paper
正在生成论文摘要