Improvements in the Large p , Small n Classification Issue
SN Computer Science(2020)
摘要
Classifying gene expression data is known to contain keys for solving the fundamental problems in cancer studies. However, this issue is a complex task because of the large p , small n issue on gene expression data analysis. In this paper, we propose the improvements in the large p , small n classification issue for the study of human cancer. First, a new enhancing sample size method with generative adversarial network is proposed to improve classification algorithms. Second, we suggest a classification approach with over-sampling technique using features extracted by deep convolutional neural network. Numerical test results on fifty very-high-dimensional and low-sample-size gene expression data datasets from the Kent Ridge Biomedical and Array Expression repositories illustrate that the proposed models are more accurate than state-of-the-art classifying models. In addition, we also have explored the performance of support vector machines, k nearest neighbors and random forests, which have improved when apply our approaches.
更多查看译文
关键词
Large p, small n classification issue, Synthetic over sampling, Enhancing data, Deep convolutional neural network, Generative adversarial network, Support vector machines, Gene expression data
AI 理解论文
溯源树
样例
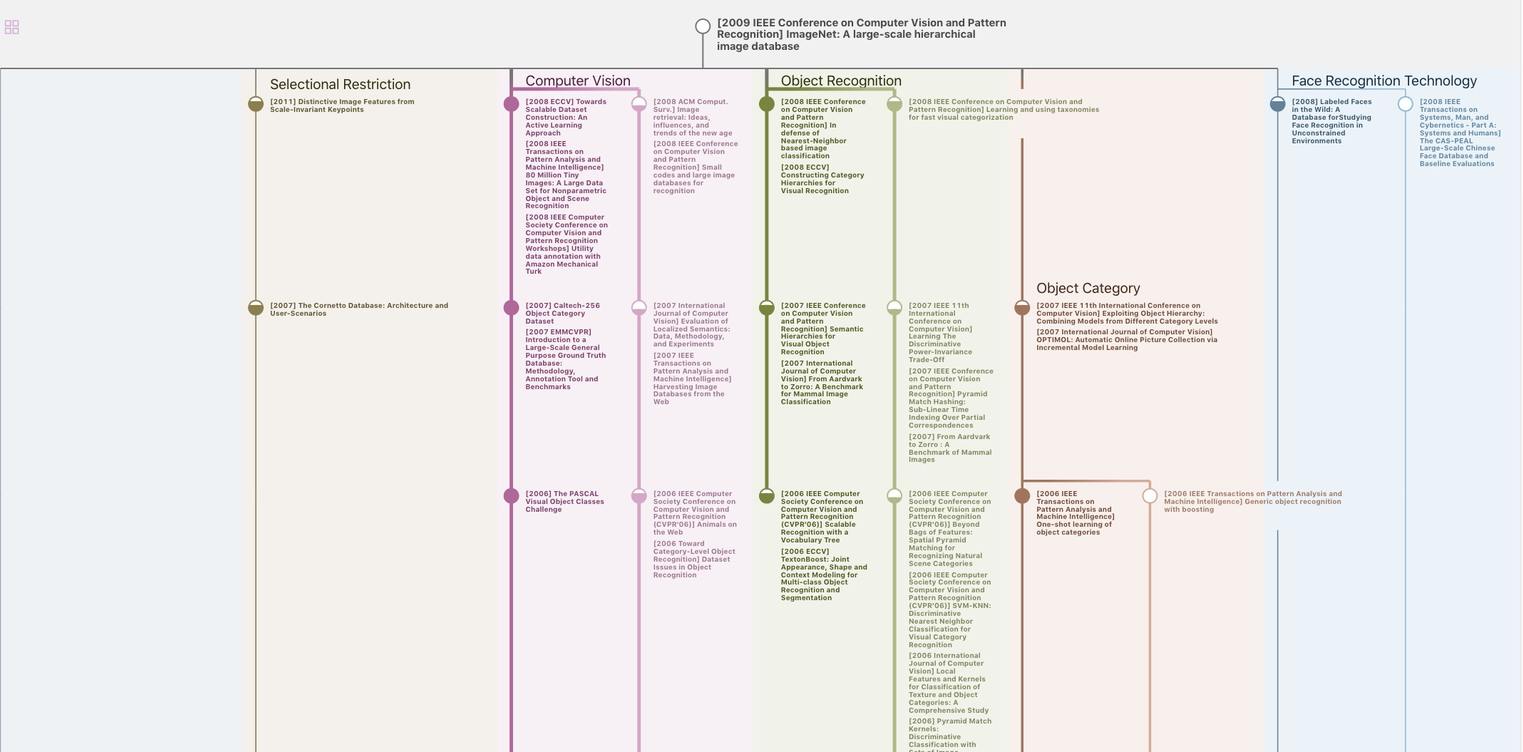
生成溯源树,研究论文发展脉络
Chat Paper
正在生成论文摘要