Analysis and classification of cardiac arrhythmia based on general sparsed neural network of ECG signals
SN Applied Sciences(2020)
摘要
In medical practices, the ECG plays an important role in diagnosing cardiac arrhythmia. In this paper efficient and most reliable technique is mentioned for the suitable classification of arrhythmia using a general sparsed neural network (GSNN). The sparsed neural network is used to extract the feature of ECG signals and then this feature is used in the neural network for processing to obtain the final classification result. The different class of ECG beat is chosen from MIT-BIH dataset. The signal to noise ratio has been calculated to filter the ECG signal. To evaluate the results, the MATLAB software is used. The main purpose of this paper is to design an efficient neural network and also to implement reliable techniques for the classification of various ECG arrhythmia conditions. The obtained accuracy level of arrhythmia detection is 98%, which is the highest rate of performance. The presented approach by GSNN to classify and predict arrhythmia will provide efficient arrhythmia detection as compared to other techniques. The suggested scheme will improve the prediction and classification efficiency.
更多查看译文
关键词
ECG signal, Feature extraction, General sparsed neural network (GSNN), Arrhythmia detection, Super vector machine
AI 理解论文
溯源树
样例
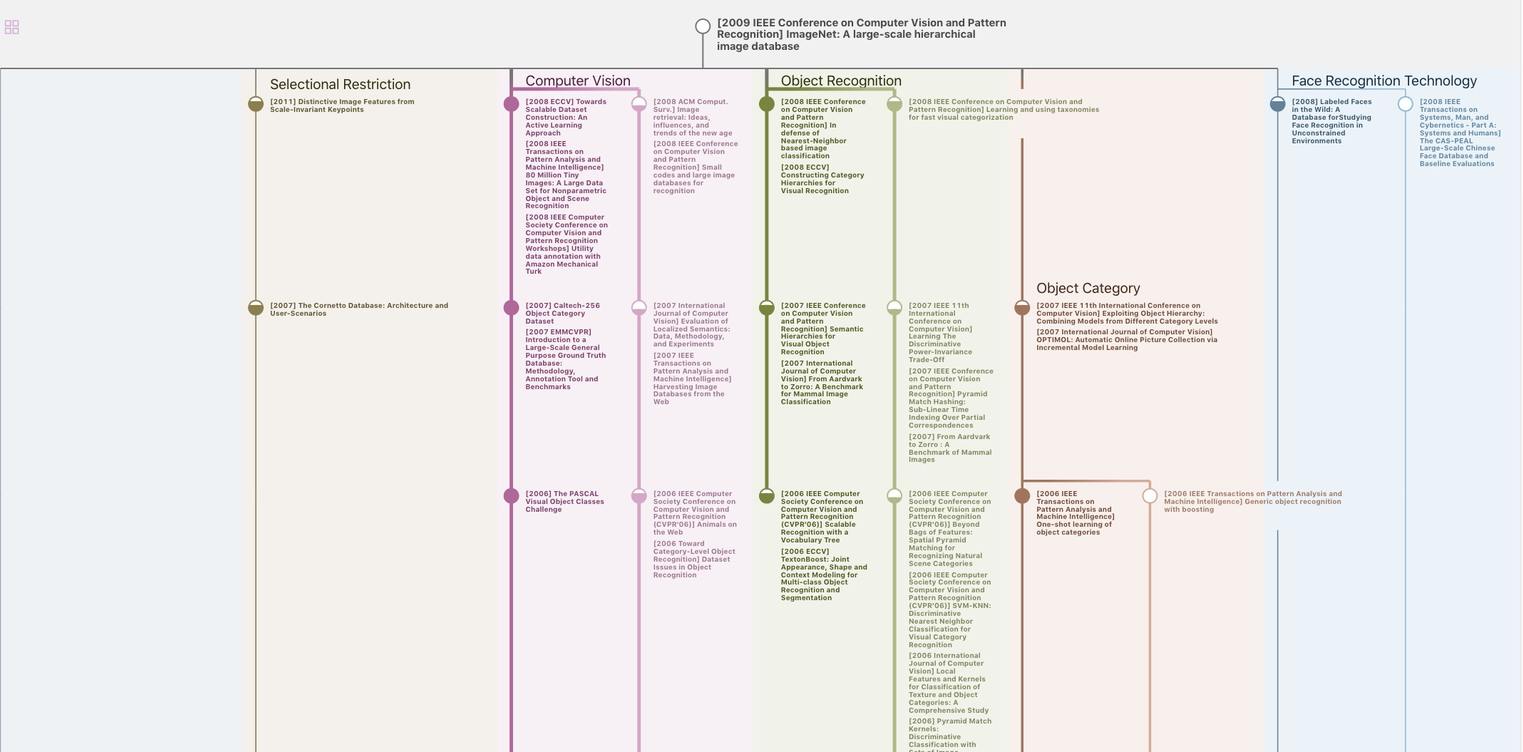
生成溯源树,研究论文发展脉络
Chat Paper
正在生成论文摘要