Trading places–simulating goalkeeper performance using spatial & body pose data
user-5ef9583f4c775ed682ecca0f(2019)
摘要
Late in the 2018 Champions League Final between Real Madrid and Liverpool with the score 2-1 in favor of the Spaniards, in the 83rd minute Real Madrid’s substitute Gareth Bale took aim at goalkeeper Loris Karius from 35 yards with a powerful yet straight strike. The rest is history, as the ball ended up sailing through Karius’ hands effectively giving Real Madrid their third straight title. The reaction to the loss was immediate by Liverpool, with the club breaking the world record fee for a goalkeeper by purchasing Brazilian Alisson for£ 67m from AS Roma from the Serie A.While this transfer triggered a flurry of other high-priced goalkeeper transfers between the top European leagues putting the cost of goalkeepers at an all-time high–it begs the questions: i) how can you compare the performance of different goalkeepers across teams and leagues?, and ii) how can you approximate whether or not a goalkeeper will be a success in your specific team? Currently goalkeepers are assessed using coarse metrics, such as “clean-sheets”,“total goals conceded” or “shots saved to goals conceded” ratio. More recently “expected metrics” such as expected saves (xS)[1] have been introduced to compare performance to the league average. Problems arise with these methods because goalkeepers could have different types of saves to make depending on the style of the team and the opponents they face.
更多查看译文
AI 理解论文
溯源树
样例
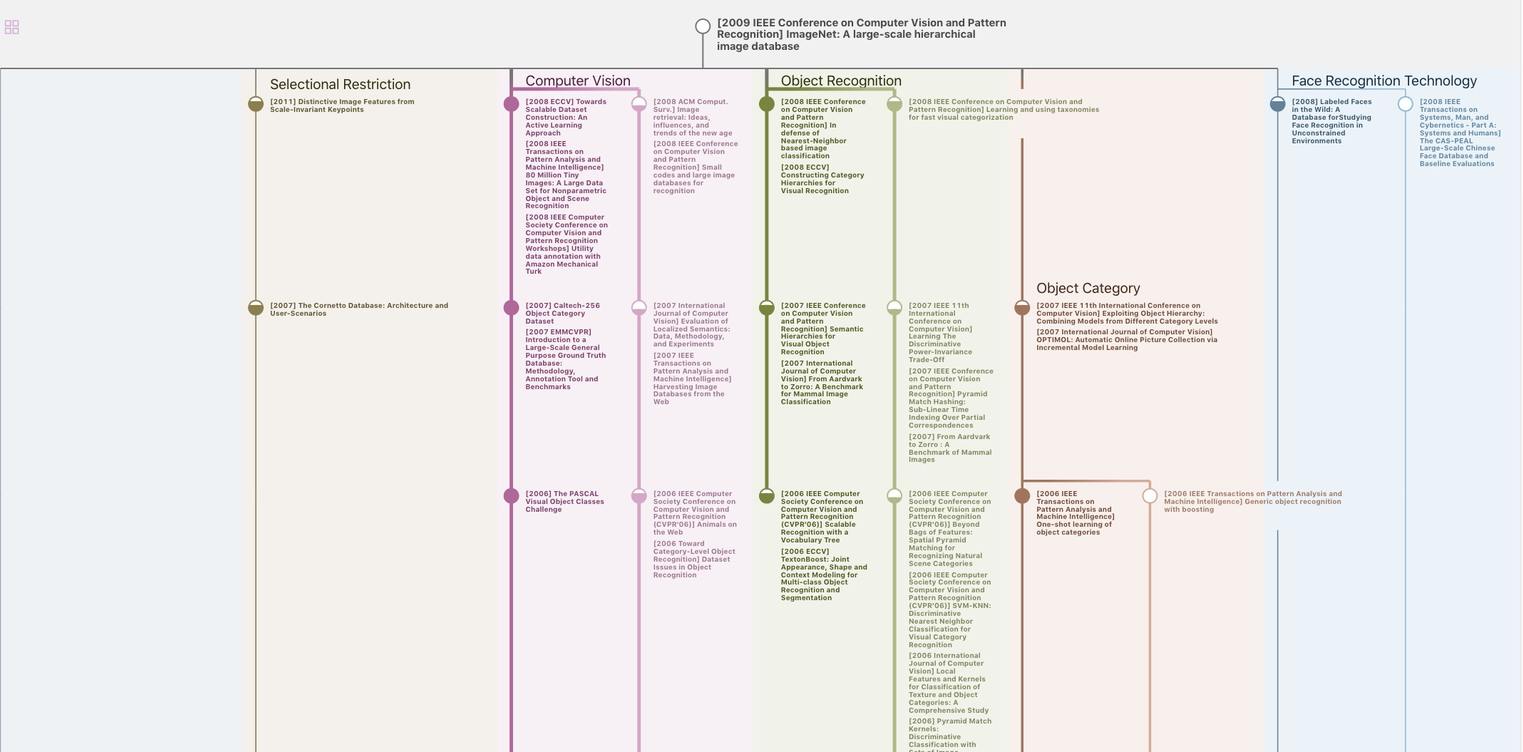
生成溯源树,研究论文发展脉络
Chat Paper
正在生成论文摘要