Exploring Aspects of Image Segmentation: Diversity, Global Reasoning, and Panoptic Formulation.
user-5ef9583f4c775ed682ecca0f(2018)
摘要
Image segmentation is the task of partitioning an image intomeaningful regions. It is a fundamental part of the visual scene understanding problem with many real-world applications, such as photo-editing, robotics, navigation, autonomous driving and bio-imaging. It has been extensively studied for several decades and has transformed into a set of problems which define meaningfulness of regions differently. The set includes two high-level tasks: semantic segmentation (each region assigned with a semantic label) and instance segmentation (each region representing object instance). Due to their practical importance, both tasks attract a lot of research attention. In this work we explore several aspects of these tasks and propose novel approaches and new paradigms. While most research efforts are directed at developing models that produce a single best segmentation, we consider the task of producing multiple diverse solutions given a single input image. This allows to hedge against the intrinsic ambiguity of segmentation task. We propose a new global model with multiple solutions for a trained segmentation model. This new model generalizes previously proposed approaches for the task. We present several approximate and exact inference techniques that suit a wide spectrum of possible applications and demonstrate superior performance comparing to previous methods. Then, we present a new bottom-up paradigm for the instance segmentation task. The new scheme is substantially different from the previous approaches that produce each instance independently. Our approach named InstanceCut reasons globally about the optimal …
更多查看译文
AI 理解论文
溯源树
样例
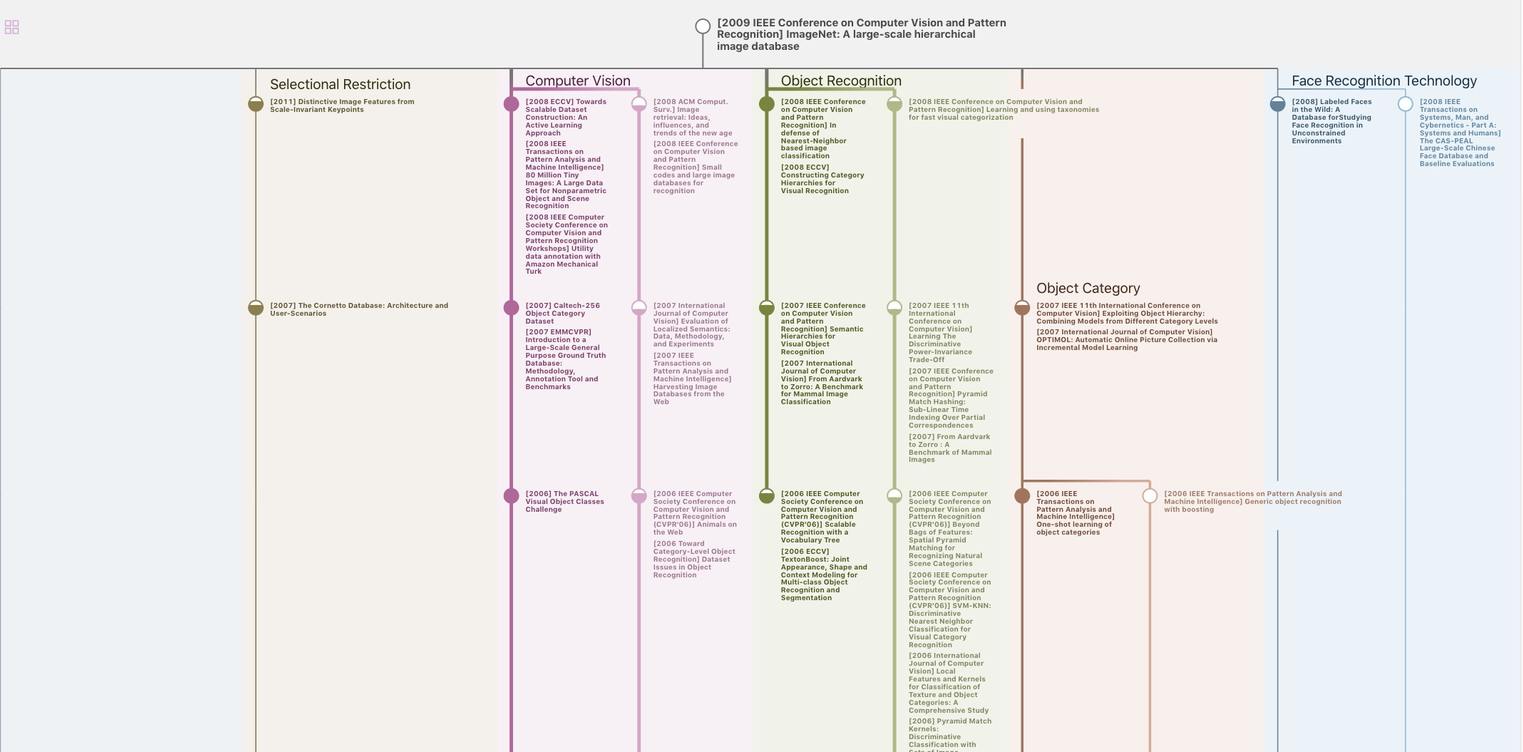
生成溯源树,研究论文发展脉络
Chat Paper
正在生成论文摘要