Do Syntax Trees Help Pre-trained Transformers Extract Information?
EACL(2021)
摘要
Much recent work suggests that incorporating syntax information from dependency trees can improve task-specific transformer models. However, the effect of incorporating dependency tree information into pre-trained transformer models (e.g., BERT) remains unclear, especially given recent studies highlighting how these models implicitly encode syntax. In this work, we systematically study the utility of incorporating dependency trees into pre-trained transformers on three representative information extraction tasks: semantic role labeling (SRL), named entity recognition, and relation extraction. We propose and investigate two distinct strategies for incorporating dependency structure: a late fusion approach, which applies a graph neural network on the output of a transformer, and a joint fusion approach, which infuses syntax structure into the transformer attention layers. These strategies are representative of prior work, but we introduce essential design decisions that are necessary for strong performance. Our empirical analysis demonstrates that these syntax-infused transformers obtain state-of-the-art results on SRL and relation extraction tasks. However, our analysis also reveals a critical shortcoming of these models: we find that their performance gains are highly contingent on the availability of human-annotated dependency parses, which raises important questions regarding the viability of syntax-augmented transformers in real-world applications.
更多查看译文
关键词
syntax trees,transformers extract information,pre-trained
AI 理解论文
溯源树
样例
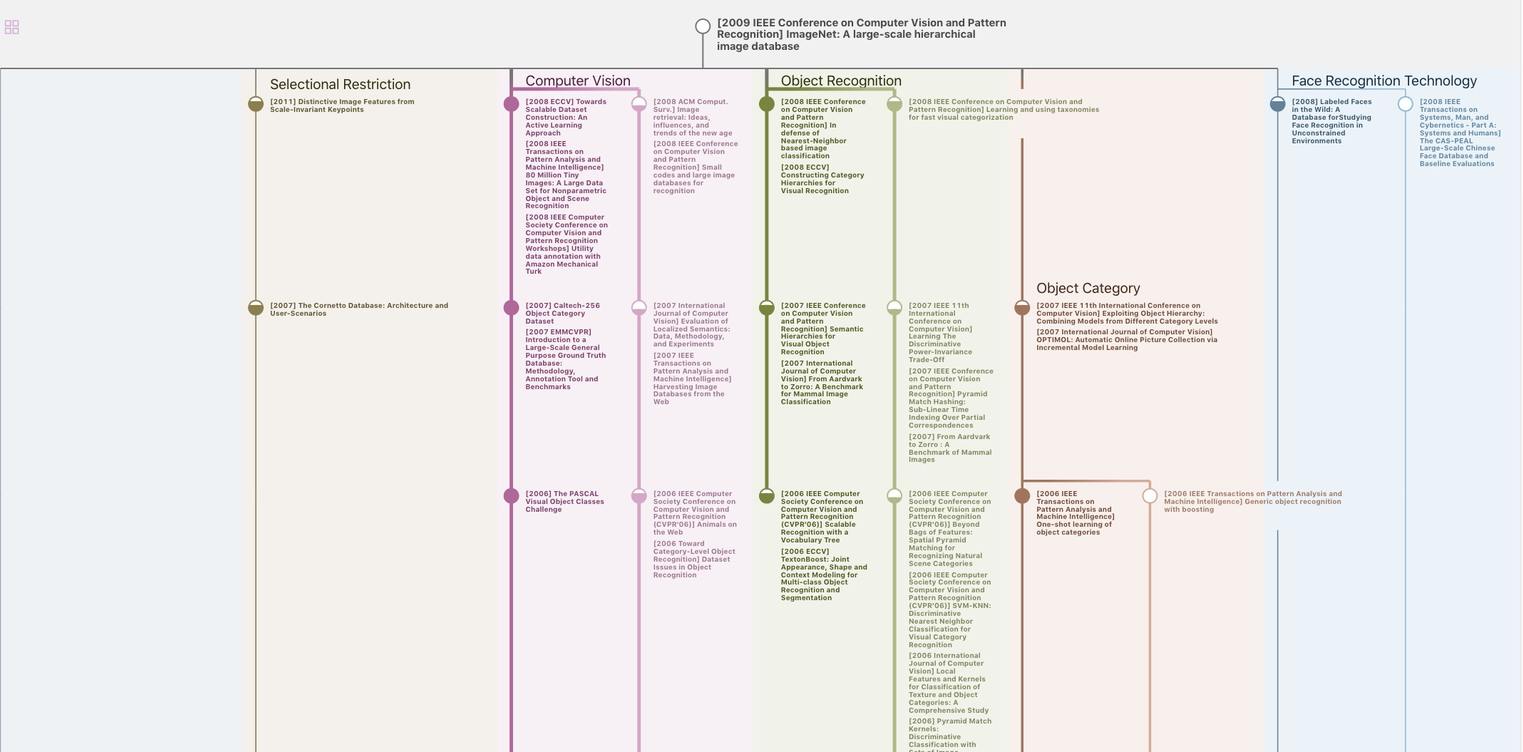
生成溯源树,研究论文发展脉络
Chat Paper
正在生成论文摘要