Smplpix: Neural Avatars From 3d Human Models
2021 IEEE WINTER CONFERENCE ON APPLICATIONS OF COMPUTER VISION (WACV 2021)(2021)
摘要
Recent advances in deep generative models have led to an unprecedented level of realism for synthetically generated images of humans. However, one of the remaining fundamental limitations of these models is the ability to flexibly control the generative process, e.g. change the camera and human pose while retaining the subject identity. At the same time, deformable human body models like SMPL [34] and its successors provide full control over pose and shape, but rely on classic computer graphics pipelines for rendering. Such rendering pipelines require explicit mesh rasterization that (a) does not have the potential to fix artifacts or lack of realism in the original 3D geometry and (b) until recently, were not fully incorporated into deep learning frameworks. In this work, we propose to bridge the gap between classic geometry-based rendering and the latest generative networks operating in pixel space. We train a network that directly converts a sparse set of 3D mesh vertices into photorealistic images, alleviating the need for traditional rasterization mechanism. We train our model on a large corpus of human 3D models and corresponding real photos, and show the advantage over conventional differentiable renderers both in terms of the level of photorealism and rendering efficiency.
更多查看译文
关键词
pose shape,classic computer graphics pipelines,rendering pipelines,explicit mesh rasterization,original 3D geometry,deep learning frameworks,classic geometry-based rendering,latest generative networks,3D mesh vertices,photorealistic images,traditional rasterization mechanism,conventional differentiable renderers,rendering efficiency,SMPLpix,neural avatars,3D human models,deep generative models,synthetically generated images,generative process,subject identity,deformable human body models
AI 理解论文
溯源树
样例
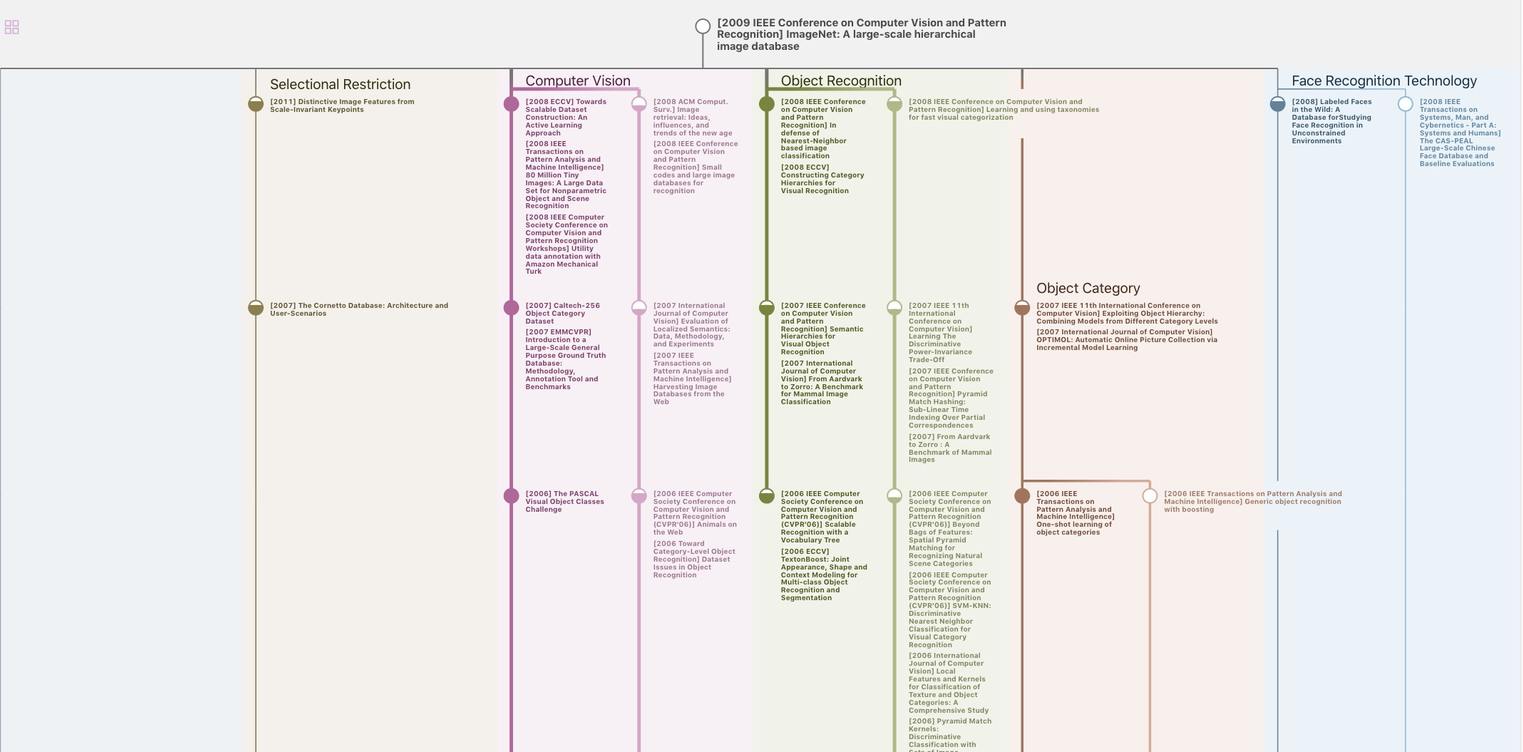
生成溯源树,研究论文发展脉络
Chat Paper
正在生成论文摘要