Data-Informed Decomposition For Localized Uncertainty Quantification Of Dynamical Systems
VIBRATION(2021)
摘要
Industrial dynamical systems often exhibit multi-scale responses due to material heterogeneity and complex operation conditions. The smallest length-scale of the systems dynamics controls the numerical resolution required to resolve the embedded physics. In practice however, high numerical resolution is only required in a confined region of the domain where fast dynamics or localized material variability is exhibited, whereas a coarser discretization can be sufficient in the rest majority of the domain. Partitioning the complex dynamical system into smaller easier-to-solve problems based on the localized dynamics and material variability can reduce the overall computational cost. The region of interest can be specified based on the localized features of the solution, user interest, and correlation length of the material properties. For problems where a region of interest is not evident, Bayesian inference can provide a feasible solution. In this work, we employ a Bayesian framework to update the prior knowledge of the localized region of interest using measurements of the system response. Once, the region of interest is identified, the localized uncertainty is propagate forward through the computational domain. We demonstrate our framework using numerical experiments on a three-dimensional elastodynamic problem.
更多查看译文
关键词
Bayesian inference, uncertainty quantification, dynamical systems, inverse problem, machine learning, system identification, Gaussian process, polynomial chaos
AI 理解论文
溯源树
样例
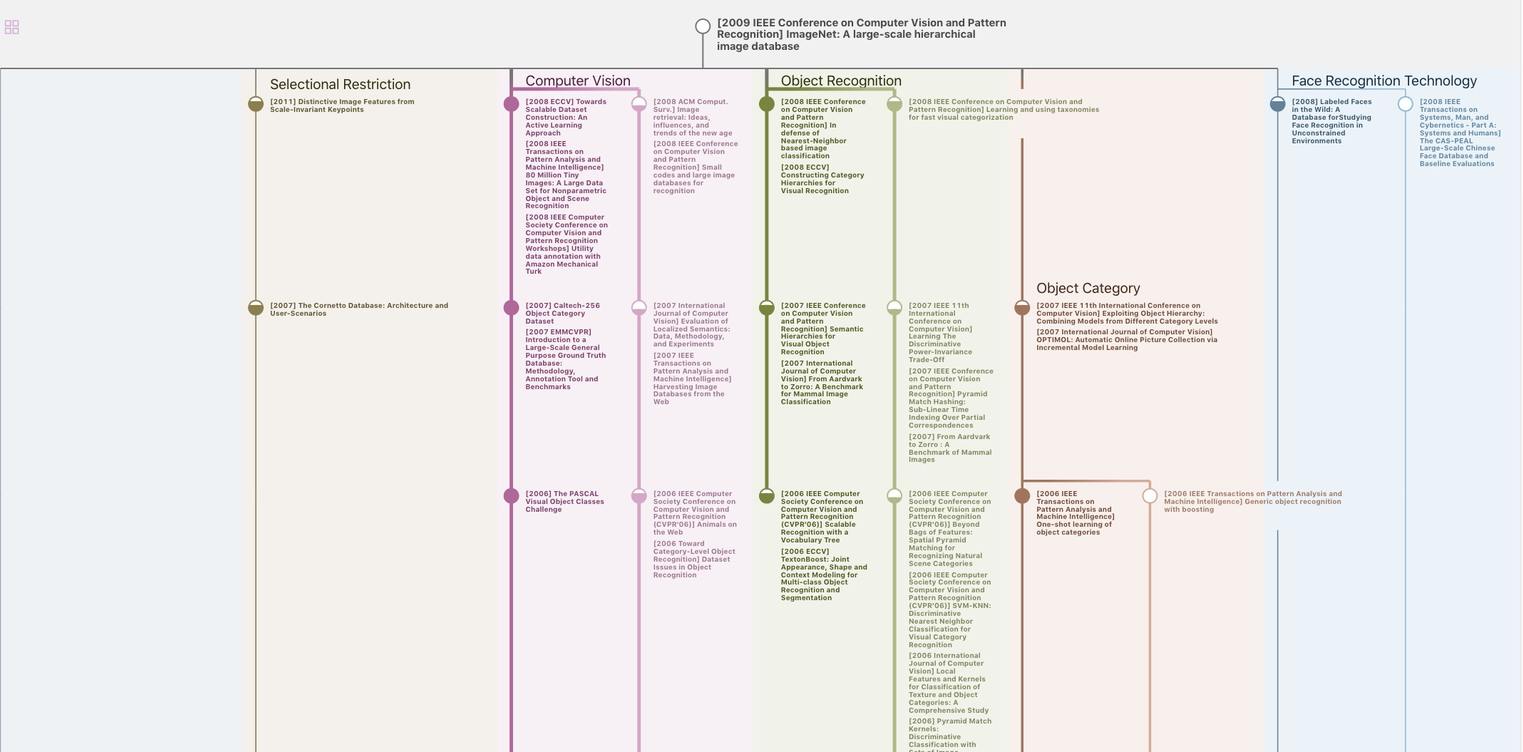
生成溯源树,研究论文发展脉络
Chat Paper
正在生成论文摘要